Statistical tools for water quality assessment and monitoring in river ecosystems - a scoping review and recommendations for data analysis
WATER QUALITY RESEARCH JOURNAL(2022)
摘要
Robust scientific inference is crucial to ensure evidence-based decision making. Accordingly, the selection of appropriate statistical tools and experimental designs is integral to achieve accuracy from data analytical processes. Environmental monitoring of water quality has become increasingly common and widespread as a result of technological advances, leading to an abundance of datasets. We conducted a scoping review of the water quality literature and found that correlation and linear regression are by far the most used statistical tools. However, the accuracy of inferences drawn from ordinary least squares (OLS) techniques depends on a set of assumptions, most prominently: (a) independence among observations, (b) normally distributed errors, (c) equal variances of errors, and (d) balanced designs. Environmental data, however, are often faced with temporal and spatial dependencies, and unbalanced designs, thus making OLS techniques not suitable to provide valid statistical inferences. Generalized least squares (GLS), linear mixed-effect models (LMMs), and generalized linear mixed-effect models (GLMMs), as well as Bayesian data analyses, have been developed to better tackle these problems. Recent progress in the development of statistical software has made these approaches more accessible and user-friendly. We provide a high-level summary and practical guidance for those statistical techniques.
更多查看译文
关键词
Bayesian data analysis, environmental monitoring, frequentist statistics, model diagnostics, multilevel models, oil sands
AI 理解论文
溯源树
样例
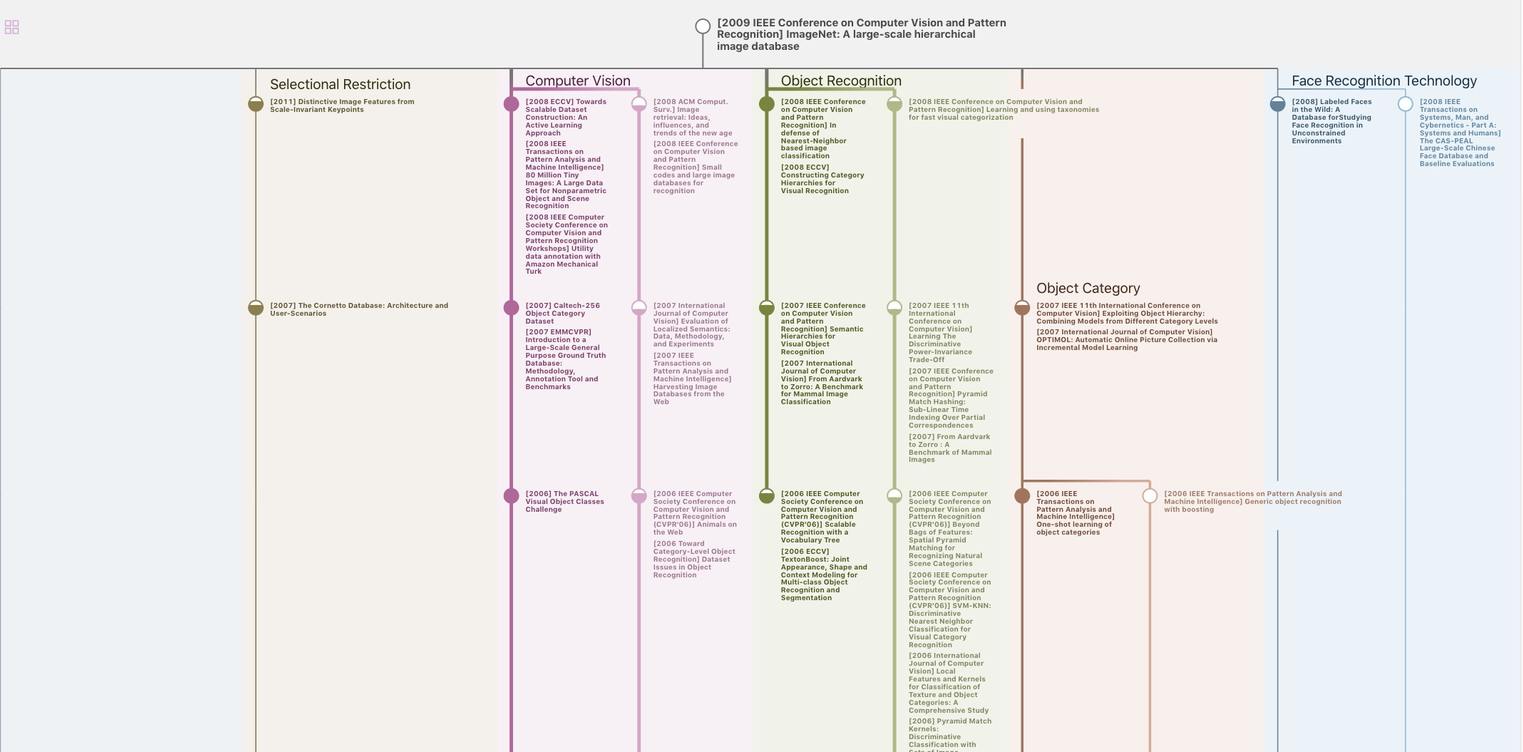
生成溯源树,研究论文发展脉络
Chat Paper
正在生成论文摘要