Inter-scanner brain MRI volumetric biases persist in a harmonized multi-subject study of multiple sclerosis
MULTIPLE SCLEROSIS JOURNAL(2021)
摘要
Background/Purpose Multicenter study designs involving a variety of MRI scanners have become increasingly common. However, these present the issue of biases in image-based measures due to scanner or site differences. To assess these biases, we imaged 11 volunteers with multiple sclerosis (MS) with scan and rescan data at 4 sites.
Materials and Methods Images were acquired on Siemens or Philips scanners at 3-tesla. Automated white matter lesion detection and whole brain, gray and white matter, and thalamic volumetry were performed, as well as expert manual delineations of T1 and T2 (FLAIR) lesions. Random effect and permutation-based nonparametric modeling was performed to assess differences in estimated volumes within and across sites.
Results Random effect modeling demonstrated model assumption violations for most comparisons of interest. Non-parametric modeling indicated that site explained > 50% of the variation for most estimated volumes. This expanded to > 75% when data from both Siemens and Philips scanners were included. Permutation tests revealed significant differences between average inter- and intra-scanner differences in most estimated brain volumes ( P < .05). The automatic activation of spine coil elements during some acquisitions resulted in a shading artifact in these images. Permutation tests revealed significant differences between thalamic volume measurements from acquisitions with and without this artifact.
Conclusion Differences in brain volumetry persisted across MR scanners despite protocol harmonization. These differences were not well explained by variance component modeling; however, statistical innovations for mitigating inter-scanner differences show promise in reducing biases in multi-center studies of MS.
### Competing Interest Statement
The authors have declared no competing interest.
更多查看译文
关键词
multiple sclerosis,inter-scanner,multi-subject
AI 理解论文
溯源树
样例
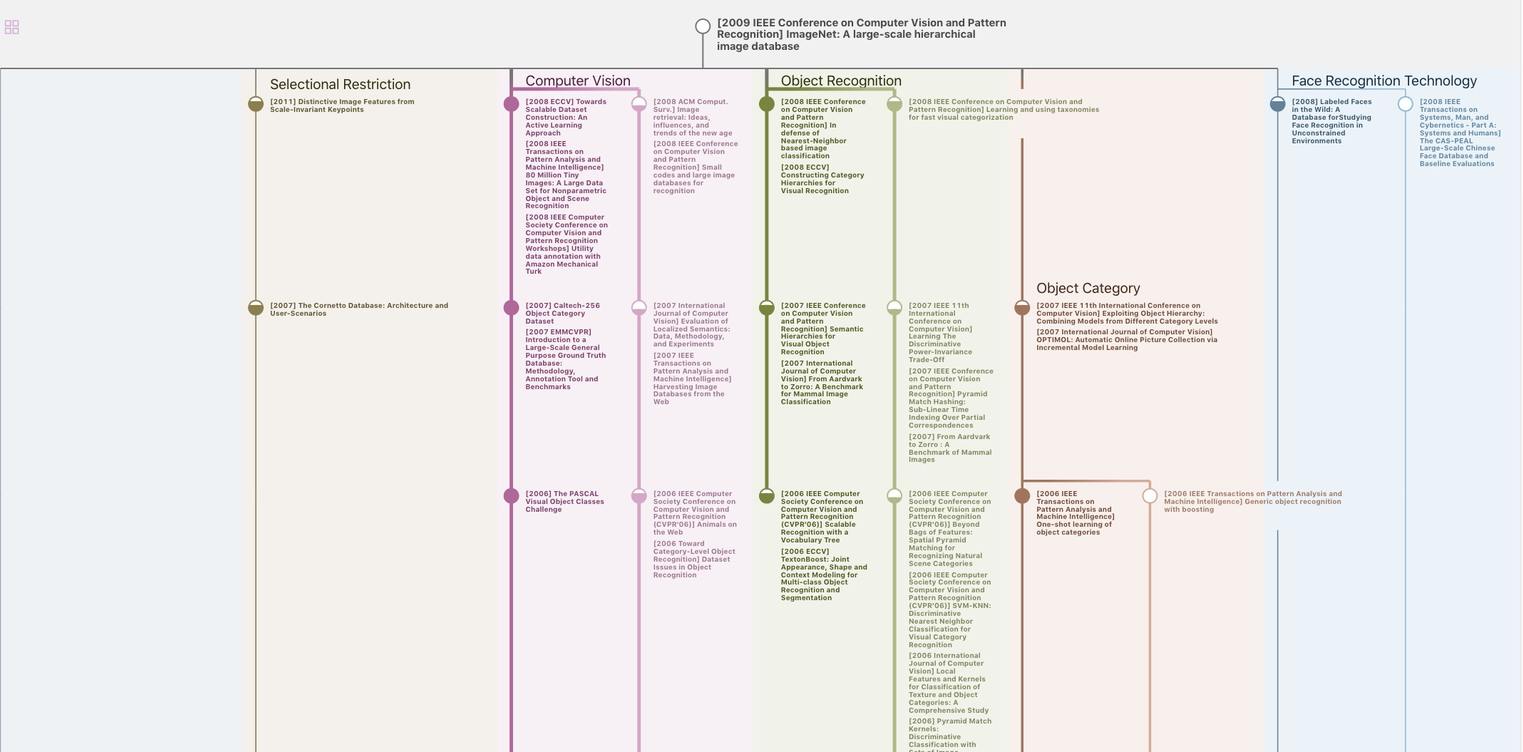
生成溯源树,研究论文发展脉络
Chat Paper
正在生成论文摘要