Improved Data-Driven Stochastic Subspace Identification with Autocorrelation Matrix Modal Order Estimation for Bridge Modal Parameter Extraction Using GB-SAR Data
BUILDINGS(2022)
摘要
With the advantage of non-contact measurement, ground-based synthetic aperture radar (GB-SAR) has been widely used to obtain the dynamic deflection of various bridges. Data-driven stochastic subspace recognition (Data-SSI), a popularized time-domain technique, is commonly used for modal parameter identification of bridges. To improve the computational efficiency and accuracy of the Data-SSI method for bridge modal parameter estimation using GB-SAR, this paper proposes an improved Data-SSI method. First, boxplot data filtering is applied to screen out the error points to generate a Hankel matrix. Second, the Hankel matrix compression method is presented to reduce the ill-conditioned vectors in the column vectors of the Hankel matrix to improve calculation efficiency. Finally, the exact modal order (EMO) modal estimation algorithm based on the autocorrelation matrix is adopted to reduce the generation of false modes and improve the calculation efficiency. The results of simulation and field experiments show that the natural frequency values for the improved Data-SSI method are 2.3208 and 2.3189 and the damping ratio coefficient values are 8.10 and 8.08, under windows 1 and 2, respectively. The operation times using the improved Data-SSI method are 2.02 s and 7.61 s under windows 1 and 2, respectively. This proves that the proposed improved Data-SSI method has higher accuracy and computational efficiency.
更多查看译文
关键词
bridge, modal parameter extraction, GB-SAR, dynamic deflection, Data-SSI, modal order estimation
AI 理解论文
溯源树
样例
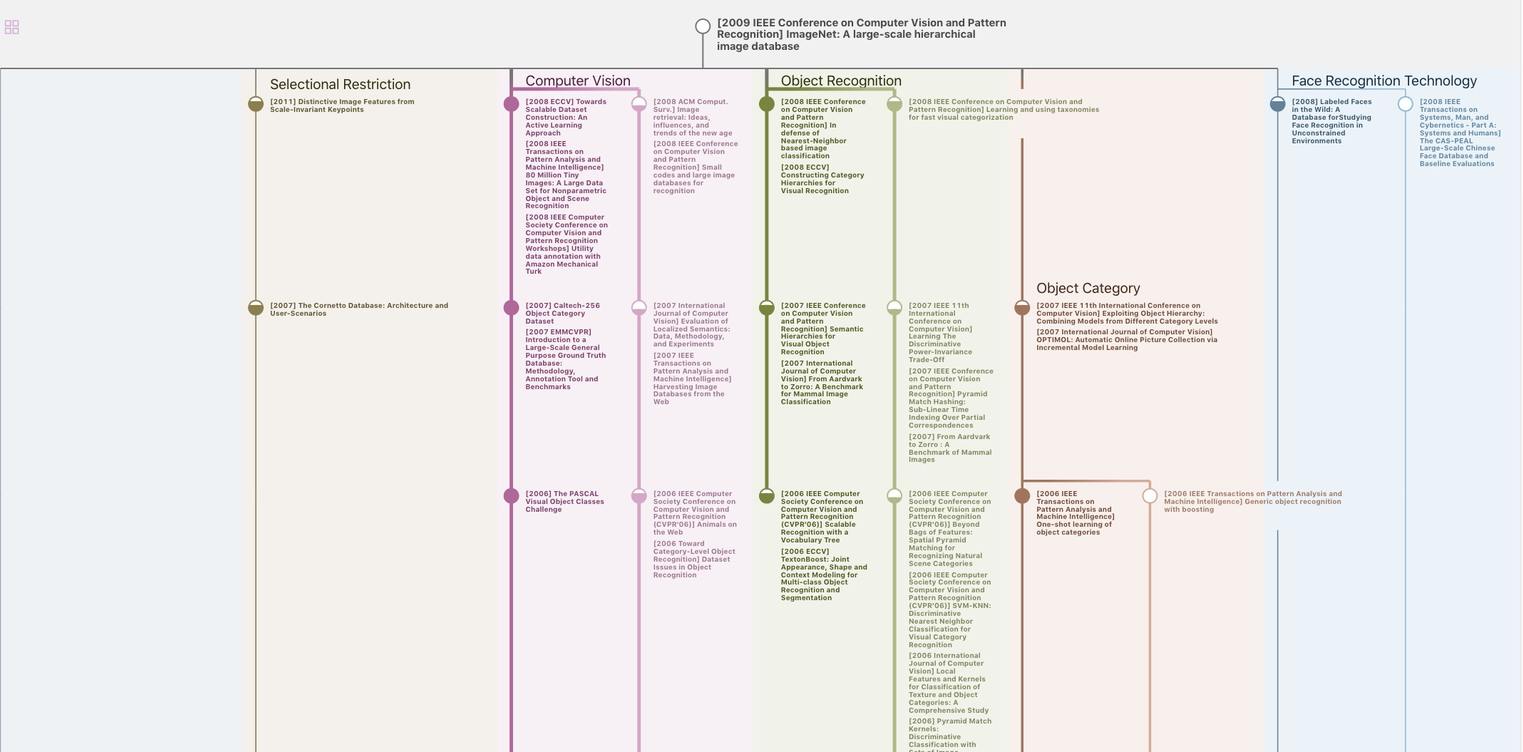
生成溯源树,研究论文发展脉络
Chat Paper
正在生成论文摘要