Landslide risk assessment of high-mountain settlements using Gaussian process classification combined with improved weight-based generalized objective function
International Journal of Disaster Risk Reduction(2022)
摘要
High-mountain landslides in the hydrogeological environment expose the residents and properties of mountain settlements to immediate risks, especially in the southwestern part of the Tibetan Plateau, where severe damage is frequent. The risk assessment of geohazards is provided as an effective disaster prevention and mitigation management measure. To reasonably predict the spatial distribution characteristics of high-mountain landslide risks, the Gaussian process classification (GPC) and the improved weight-based generalized objective function were applied to quantify the hazard, vulnerability, and risk values of Li County, Southwest China. The findings revealed that the hazard, vulnerability, and risk levels in the studied areas were comparatively high, with percentages in the moderate–high ranges of 41.715%, 40.634%, and 57.135%, respectively. The towns of Zagunao, Ganbu, and Taoping are among the regions with a high grade of risk, with risk values of 0.407, 0.347, and 0.335, respectively. These high-risk distribution sites are concentrated predominantly in the municipalities and their fringes, whereas other prefectures are in the medium–below risk level range. Following the examination of the GPC model and integral appraisal performance, this risk assessment framework exhibits good predictability, with an accurate proportion of predictions in the hazardous location interval reaching 99.857% and an ROC-AUC value of 0.97. The proceedings of this study can provide essential materials and references for decision-making for hydrogeological-environmental hazard policy formulation, territorial spatial planning, and other regional-related risk assessment projects.
更多查看译文
关键词
The high-mountain landslide,Risk assessment,Gaussian process classification,Improved weight-based generalized objective function,Southwest China
AI 理解论文
溯源树
样例
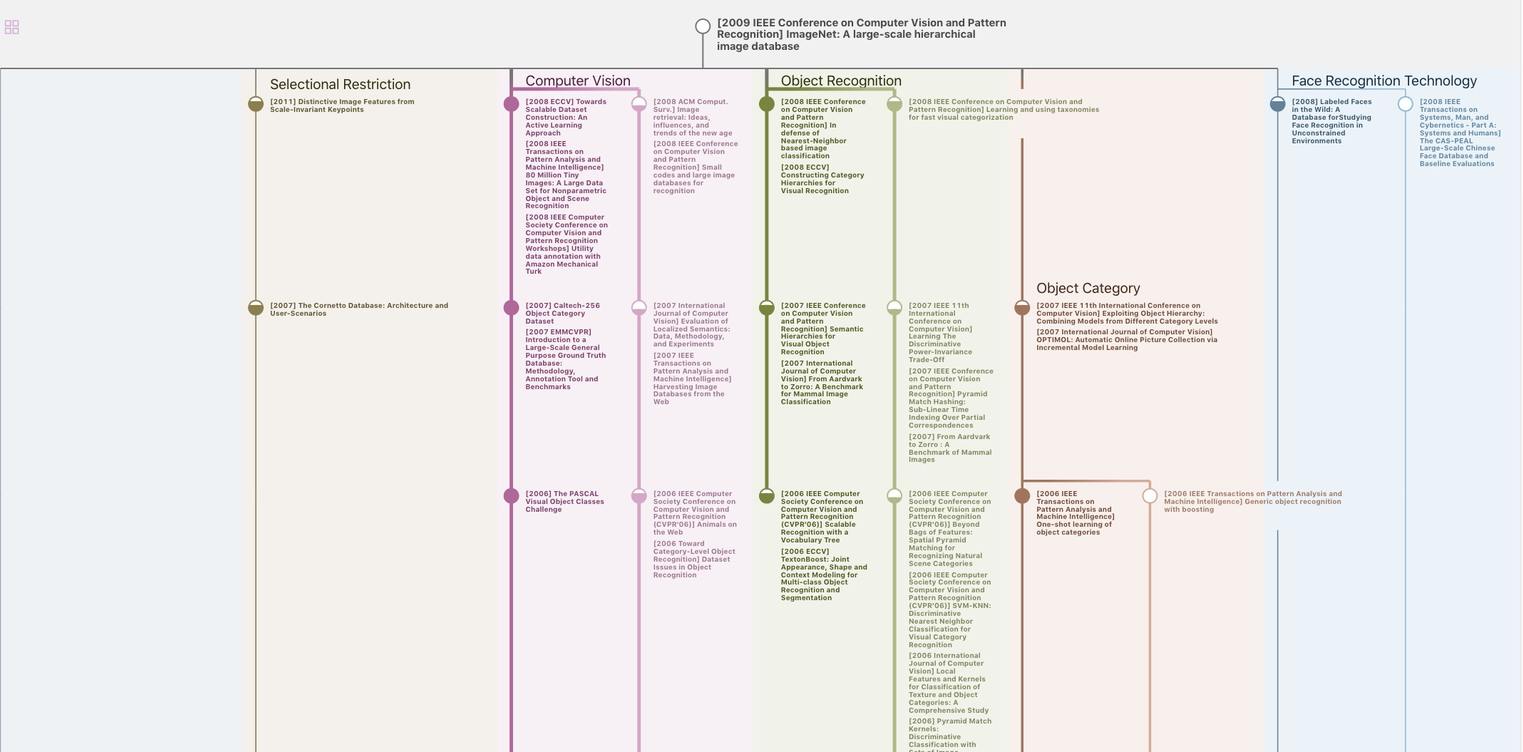
生成溯源树,研究论文发展脉络
Chat Paper
正在生成论文摘要