An automatic P-wave onset time picking method for mining-induced microseismic data based on long short-term memory deep neural network
GEOMATICS NATURAL HAZARDS & RISK(2022)
摘要
The automatic P-wave onset time (P-onset) picking of microseismic (MS) waveforms generated during rock failure is the basis of and key to locating the source and exploring the failure mechanism of rock failure in underground engineering. However, it is hard to ensure the accuracy in picking P-onset of MS waveforms with a low signal-to-noise ratio (SNR) mixed with noises. Moreover, traditional P-onset picking methods mainly depend on manual intervention, which imposes onerous requirements on the SNR of MS waveforms. Hence, a new P-onset picking method is proposed based on the deep learning model: the principal components of MS waveforms are extracted based on the multi-channel singular spectrum analysis (MSSA) method to reduce the influence of noise; afterwards, a short-time Fourier transform (STFT) is applied to the processed MS waveforms to attain essential parameters of the waveforms; finally, a model for P-onset picking is established based on the long short-term memory (LSTM) network. Subsequently, a comparison is conducted between the proposed method and other methods based on actual field MS data collected from Xiadian Gold Mine and the seismic data from Stanford Earthquake Dataset. The results show that the proposed method can accurately extract data features of MS waveforms and further improve the P-onset picking performance.
更多查看译文
关键词
Onset time picking, LSTM, mine MS monitoring, feature recognition
AI 理解论文
溯源树
样例
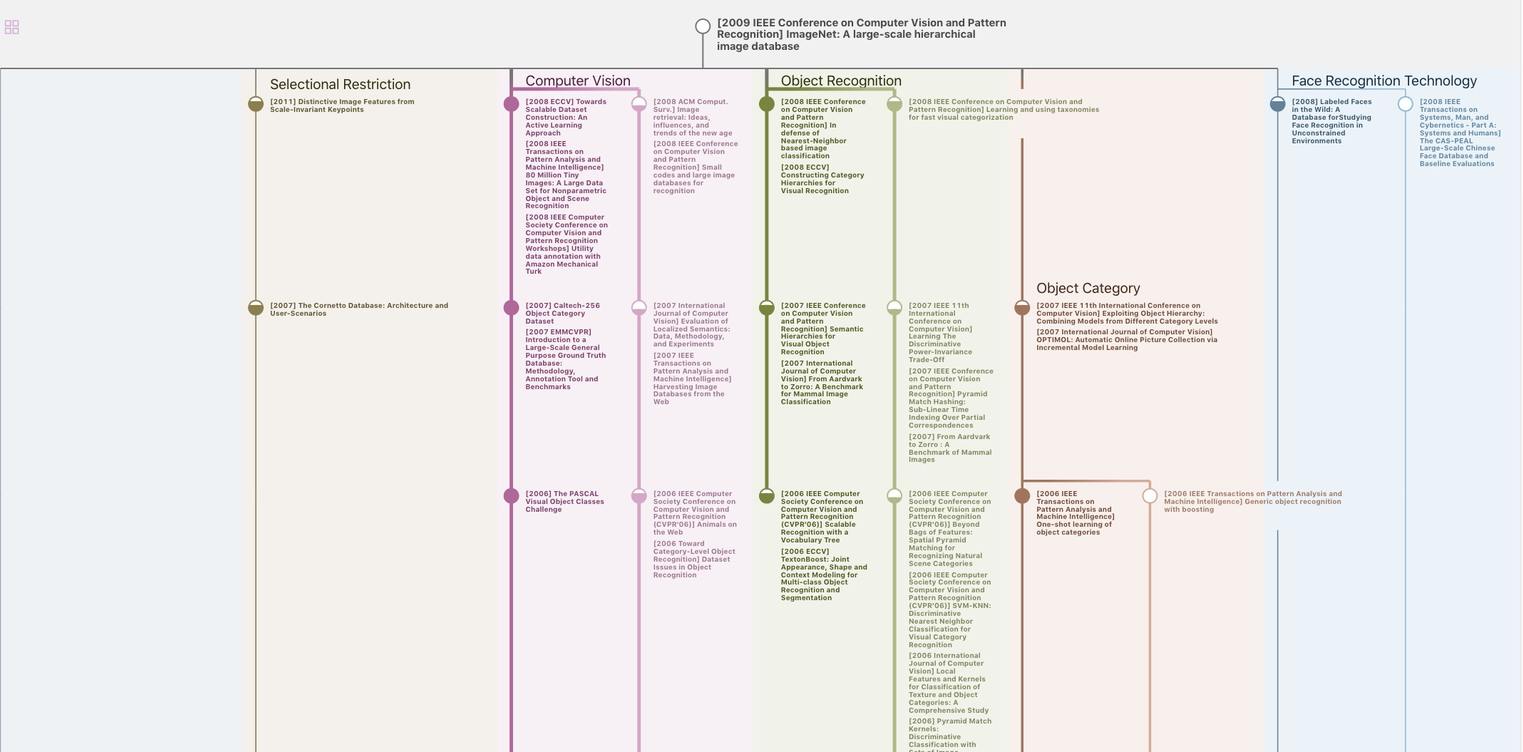
生成溯源树,研究论文发展脉络
Chat Paper
正在生成论文摘要