Semi-paired Image-to-Image Translation using Neighbor-based Generative Adversarial Networks
2021 INTERNATIONAL JOINT CONFERENCE ON NEURAL NETWORKS (IJCNN)(2021)
摘要
Image-to-image translation aims at learning the mapping between an input image and an output image using a training set of aligned image pairs. In reality, obtaining paired images is difficult and expensive. Generally, the data often exist in the form of partial pairing, that is, a small number of images are paired and most of the images are not paired. In this paper, we present a semi-paired image-to-image translation approach using neighbor-based generative adversarial networks. Our goal is to break the restriction that training images must be paired, and meanwhile guarantee the quality of image translation. For the unpaired images, we introduce an inverse mapping and cycle consistency loss to enforce the image reconstruction; for the paired images, we make full use of the one-to-one strong correlation to guide the image translation. To further take advantage of the paired images, our approach employs neighbor images to further expand the paired information and establishes the neighbor-based cycle consistency. Our method is characterized by flexibility and adaptability under various scenarios, such as target deformation, day-night transformation, etc. Compared with the previous methods, the experimental results prove the superiority of our method.
更多查看译文
关键词
Semi-paired image-to-image translation, Neighbor information, Generative adversarial networks
AI 理解论文
溯源树
样例
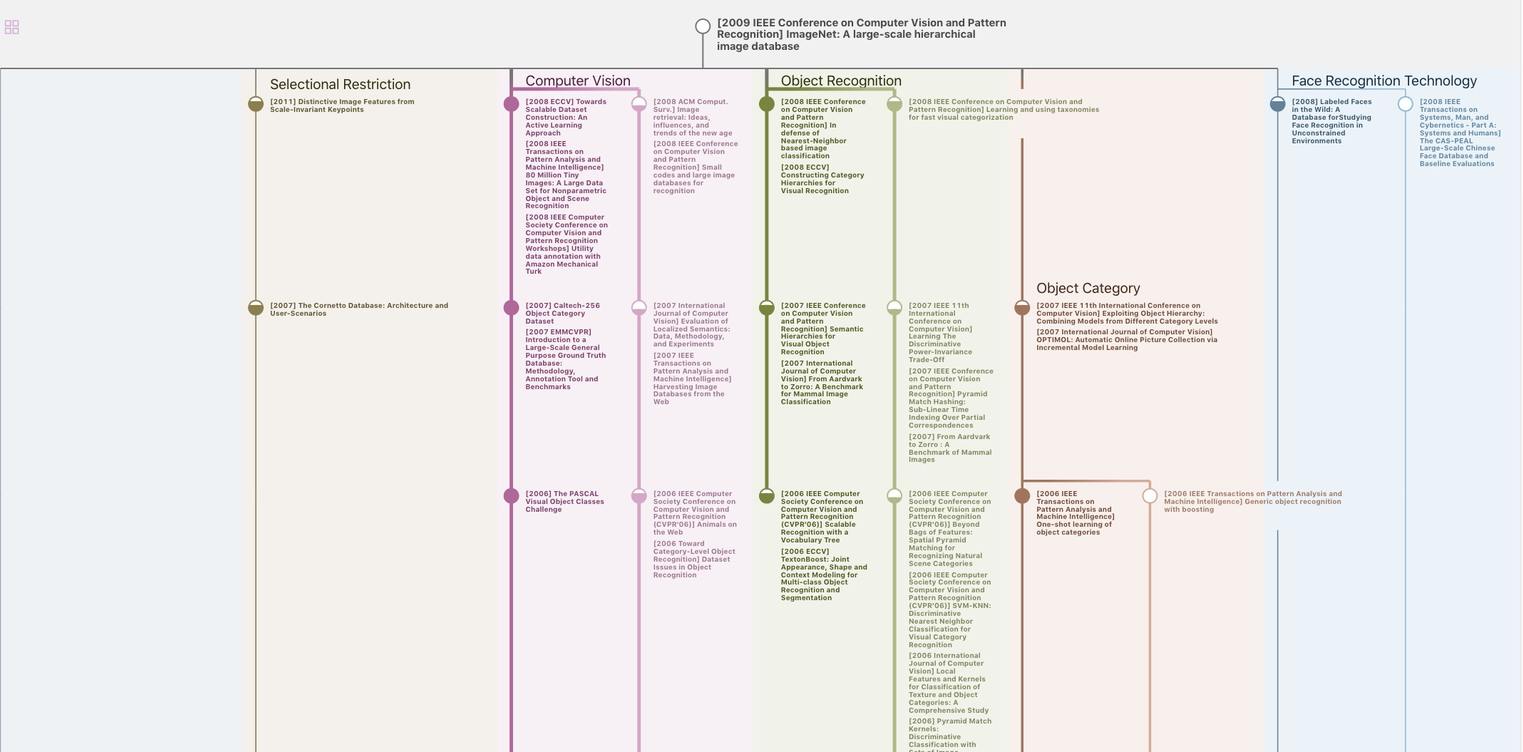
生成溯源树,研究论文发展脉络
Chat Paper
正在生成论文摘要