Real-Time Text Classification of User-Generated Content on Social Media: Systematic Review
IEEE Transactions on Computational Social Systems(2022)
摘要
The aim of this systematic review is to determine the current state of the art in the real-time classification of user-generated content from social media. Focus is on the identification of the main characteristics of data used for training and testing, the types of text processing and normalization that are required, the machine learning methods used most commonly, and how these methods compare to one another in terms of classification performance. Relevant studies were selected from subscription-based digital libraries, free-to-access bibliographies, and self-curated repositories and then screened for relevance with key information extracted and structured against the following facets: natural language processing (NLP) methods, data characteristics, classification methods, and evaluation results. A total of 25 studies published between 2014 and 2018 covering 15 types of classification algorithms were included in this review. Support vector machines (SVMs), Bayesian classifiers, and decision trees were the most commonly employed algorithms with recent emergence of neural network approaches. Domain-specific, application programming interface (API)-driven collection is the most prevalent origin of datasets. The reuse of previously published datasets as a means of benchmarking algorithms against other studies is also prevalent. In conclusion, there are consistent approaches taken when normalizing social media data for text mining and traditional text mining techniques are suited to the task of real-time analysis of social media.
更多查看译文
关键词
Data preprocessing,decision trees,naive Bayes,neural networks,real-time systems,social media,support vector machines (SVMs),systematic literature review,text classification,text mining
AI 理解论文
溯源树
样例
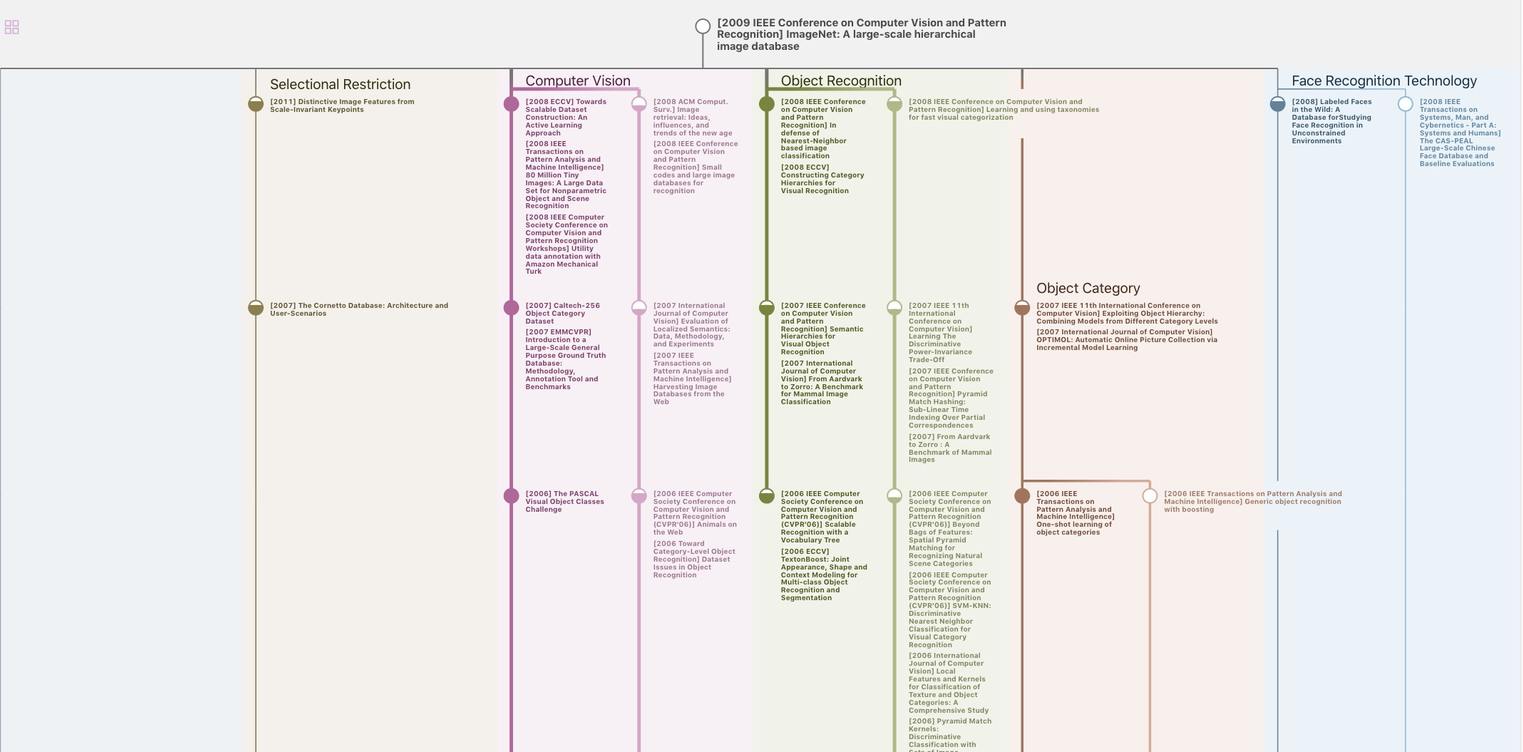
生成溯源树,研究论文发展脉络
Chat Paper
正在生成论文摘要