A Quantitative and Content-Based Approach for Evaluating the Impact of Counter Narratives on Affective Polarization in Online Discussions
IEEE Transactions on Computational Social Systems(2022)
摘要
The increasingly widespread usage of the Internet and social networks has changed the way people interact with each other and react to events. These interactions enable positive collective outcomes such as enhancing collaboration in science. Conversely, undesirable effects have emerged: the self-segregation of online users within “bubbles” of biased content, the spread of misinformation, and the growing diffusion of aggressive speech with radical emotional valence. Affective polarization is the extent to which two opposing groups dislike one another, and it could be measured as the degree to which the two groups are willing to discriminate one against the other. Such social mechanism could occur in online social networks as a result of a controversial event in the offline world. To counter affective polarization, influential actors often make interventions using counter narratives in online social networks. However, a quantitative measure of the effectiveness of such counter narratives is typically not provided. In this study, we propose an approach to evaluate the affective polarization in online discussions generated by an offline controversial event and a measure of the effectiveness of counter narratives made by influential actors to attenuate the rise of affective polarization. The proposed approach was applied to five cases of controversial events that occurred in European soccer leagues using data collected from Twitter. Such an approach could be generalized to any other scenario involving an offline event that sparks divergent emotional reactions in online discussions and an official social media account that intervenes with a counter narrative to impact affective polarization.
更多查看译文
关键词
Affective polarization,counter narratives,natural language processing,online social networks,sentiment
AI 理解论文
溯源树
样例
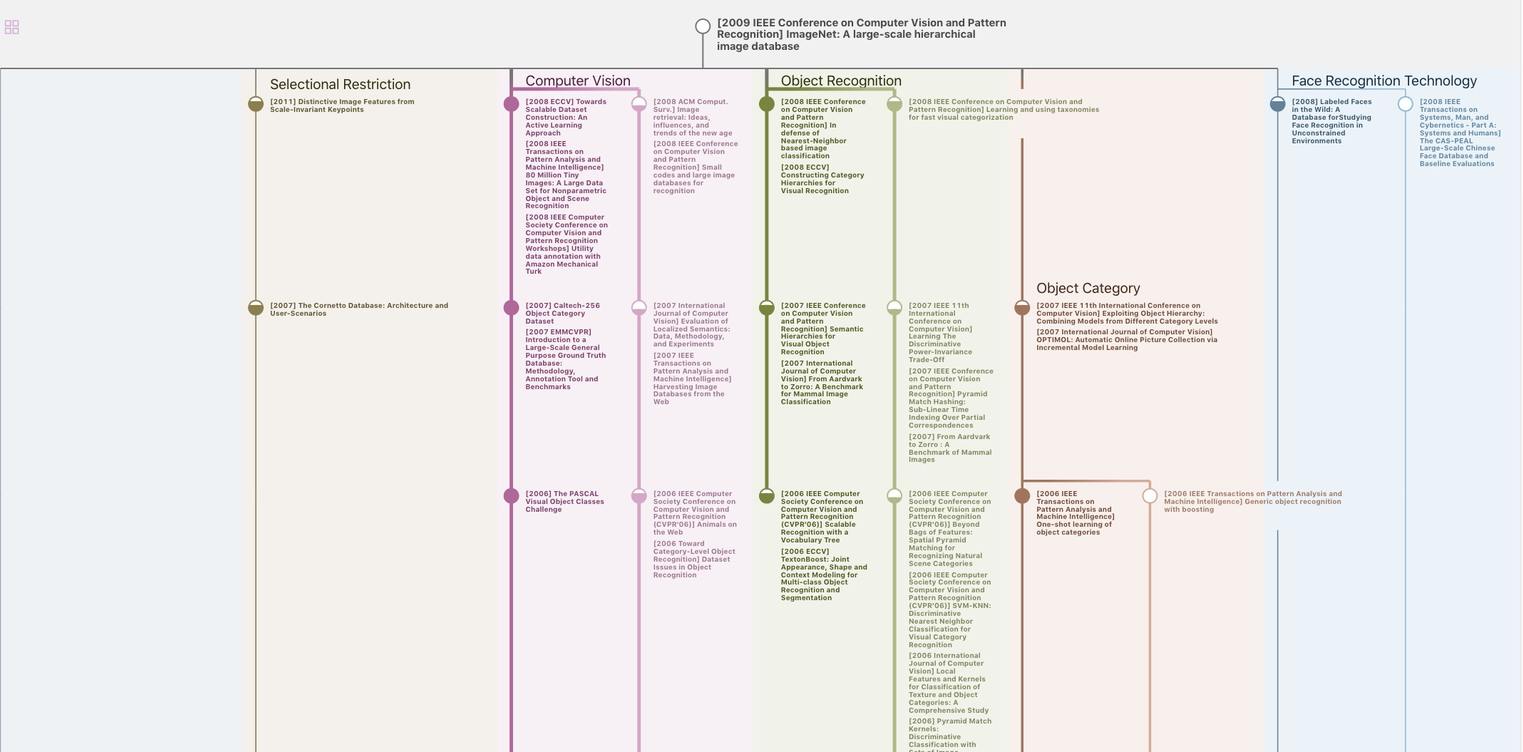
生成溯源树,研究论文发展脉络
Chat Paper
正在生成论文摘要