On robust probabilistic principal component analysis using multivariate t-distributions
COMMUNICATIONS IN STATISTICS-THEORY AND METHODS(2023)
摘要
Probabilistic principal component analysis (PPCA) is a probabilistic reformulation of principal component analysis (PCA), under the framework of a Gaussian latent variable model. To improve the robustness of PPCA, it has been proposed to change the underlying Gaussian distributions to multivariate t-distributions. Based on the representation of t-distribution as a scale mixture of Gaussian distributions, a hierarchical model is used for implementation. However, in the existing literature, the hierarchical model implemented does not yield the equivalent interpretation. In this paper, we present two sets of equivalent relationships between the high-level multivariate t-PPCA framework and the hierarchical model used for implementation. In doing so, we clarify a current misrepresentation in the literature, by specifying the correct correspondence. In addition, we discuss the performance of different multivariate t robust PPCA methods both in theory and simulation studies, and propose a new Monte Carlo expectation-maximization (MCEM) algorithm to implement one general type of such models.
更多查看译文
关键词
Heavy-tailed regression, latent variable, principal components, robust estimation
AI 理解论文
溯源树
样例
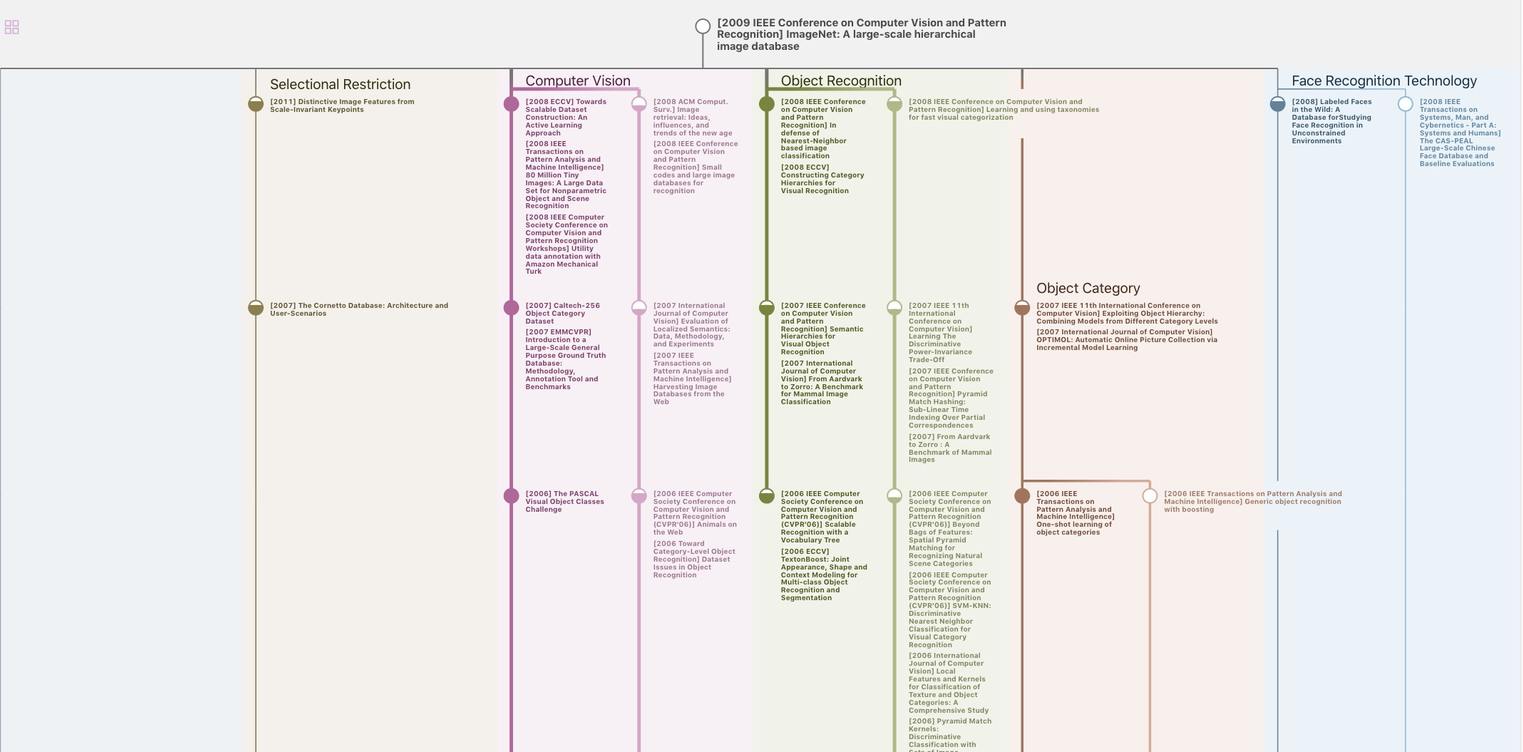
生成溯源树,研究论文发展脉络
Chat Paper
正在生成论文摘要