A nonstationary and non-Gaussian moving average model for solar irradiance
ENVIRONMETRICS(2022)
摘要
Historically, power has flowed from large power plants to customers. Increasing penetration of distributed energy resources such as solar power from rooftop photovoltaic has made the distribution network a two-way-street with power being generated at the customer level. The incorporation of renewables introduces additional uncertainty and variability into the power grid. Distribution network operation studies are being adapted to include renewables; however, such studies require high quality solar irradiance data that adequately reflect realistic meteorological variability. Data from satellite-based products are spatially complete, but temporally coarse, whereas solar irradiances exhibit high frequency variation at very fine timescales. We propose a new stochastic method for temporally downscaling global horizontal irradiance (GHI) to 1 min resolution, but we do not consider the spatial aspect due to limited availability of the in situ irradiance measurements. Solar irradiance's first and second-order structures vary diurnally and seasonally, and our model adapts to such nonstationarity. Empirical irradiance data exhibits highly non-Gaussian behavior; we develop a nonstationary and non-Gaussian moving average model that is shown to capture realistic solar variability at multiple timescales. We also propose a new estimation scheme based on Cholesky factors of empirical autocovariance matrices, bypassing difficult and inaccessible likelihood-based approaches. The model is demonstrated for a case study of three locations that are located in diverse climates through the United States. The model is compared against competitors from the literature and is shown to provide better uncertainty and variability quantification on testing data.
更多查看译文
关键词
Cholesky factor, high-resolution, statistical temporal downscaling
AI 理解论文
溯源树
样例
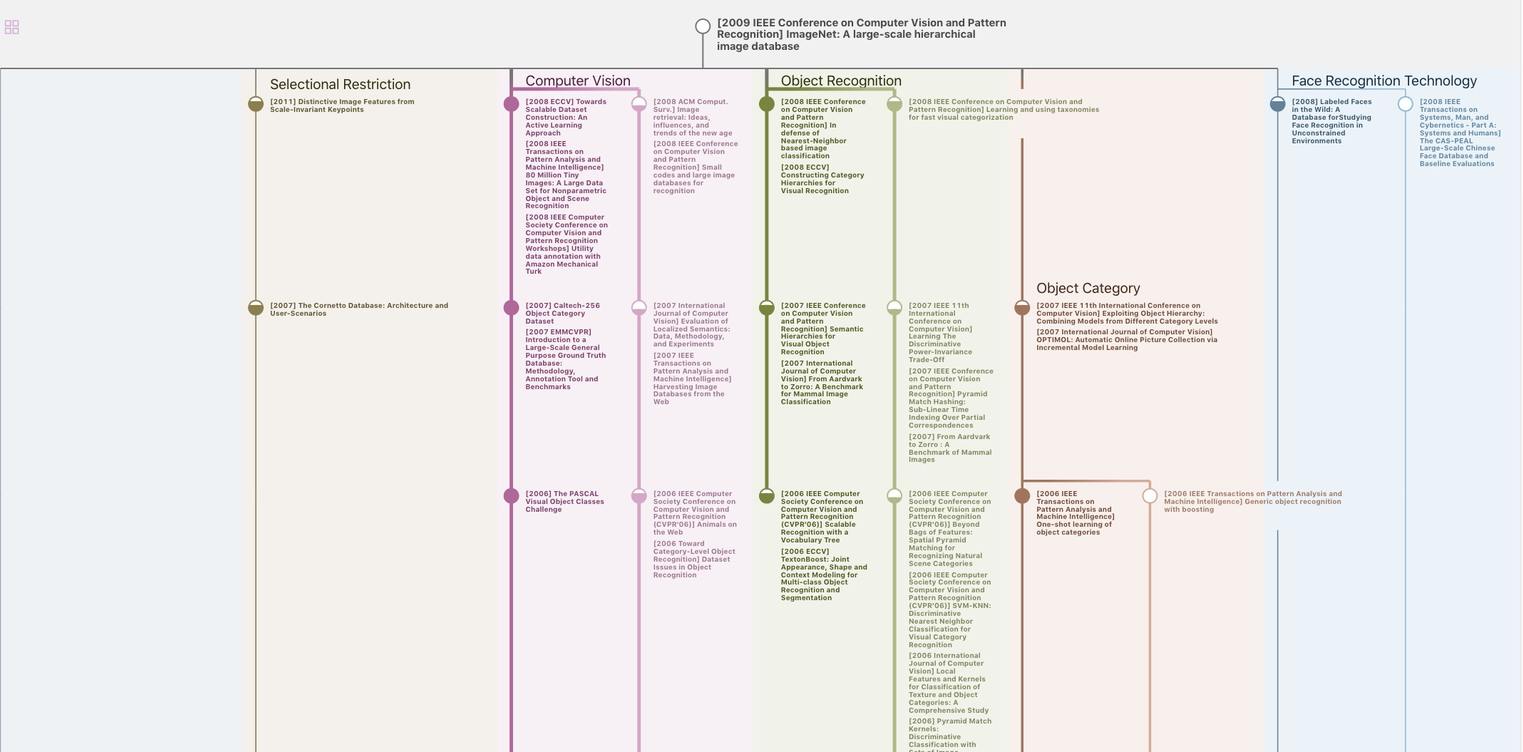
生成溯源树,研究论文发展脉络
Chat Paper
正在生成论文摘要