Comparison of Selection Criteria for Model Selection of Support Vector Machine on Physiological Data with Inter-Subject Variance
APPLIED SCIENCES-BASEL(2022)
摘要
Support vector machines (SVMs) utilize hyper-parameters for classification. Model selection (MS) is an essential step in the construction of the SVM classifier as it involves the identification of the appropriate parameters. Several selection criteria have been proposed for MS, but their usefulness is limited for physiological data exhibiting inter-subject variance (ISV) that makes different characteristics between training and test data. To identify an effective solution for the constraint, this study considered a leave-one-subject-out cross validation-based selection criterion (LSSC) with six well-known selection criteria and compared their effectiveness. Nine classification problems were examined for the comparison, and the MS results of each selection criterion were obtained and analyzed. The results showed that the SVM model selected by the LSSC yielded the highest average classification accuracy among all selection criteria in the nine problems. The average accuracy was 2.96% higher than that obtained with the conventional K-fold cross validation-based selection criterion. In addition, the advantage of the LSSC was more evident for data with larger ISV. Thus, the results of this study can help optimize SVM classifiers for physiological data and are expected to be useful for the analysis of physiological data to develop various medical decision systems.
更多查看译文
关键词
support vector machine, model selection, physiological data, inter-subject variance
AI 理解论文
溯源树
样例
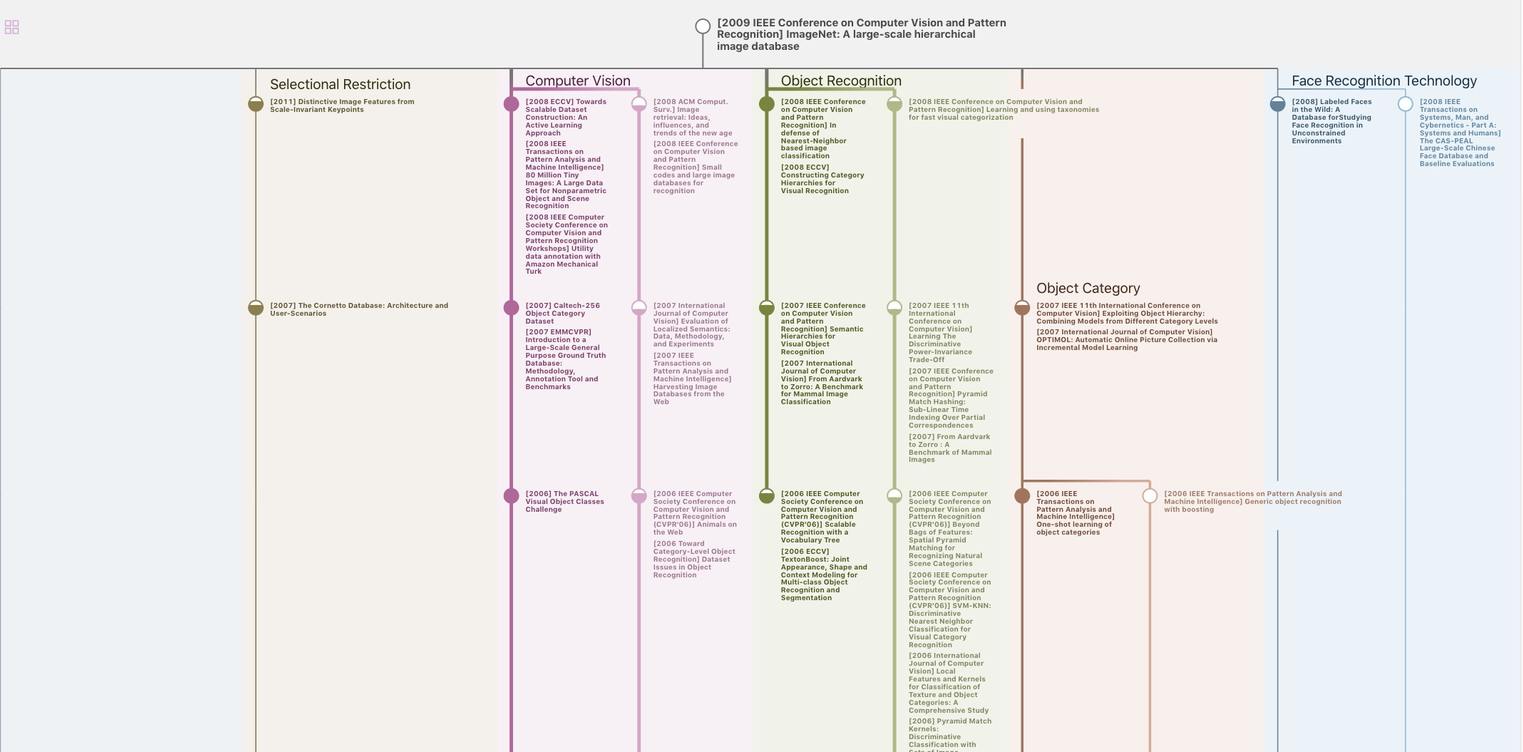
生成溯源树,研究论文发展脉络
Chat Paper
正在生成论文摘要