MACHINE LEARNING ALGORITHMS CAN PREDICT FUTURE BLASTOCYST DEVELOPMENT AND BLASTOCYST QUALITY BASED EMBRYOS CHARACTERISTICS ON DAY 1, 2 AND 3 WITH ACCURACY RESPECTFULLY OF 77% AND 69% BASED ON MORPHOLOGIC ASSESSMENT.
FERTILITY AND STERILITY(2021)
摘要
In common practice, one waits until day 5 (d5) to assess the blastocyst quality. The Istanbul Consensus established criteria for morphology assessment and what one can expect at each stage of embryo development. One of the most used scoring methods, the Gardner scoring, is based exclusively on d5 characteristics. Can Machine Learning (ML) algorithms predict the quality of the blastocyst at d5, based on morphologic assessment until day 3 (d3) without timelapse? A previous statistical analysis, realized in POMA fertility clinic, led to a new cumulative scoring method based on early development. Only embryos that cleave early or have no evidence of 2PN structures on d1 PM can receive the best grades on d5. The study was conducted on retrospective data collected from 2013 to 2020 consisting of 46740 embryos (4189 cycles), reduced to 38994 embryos (3432 c.) after donor exclusion and removing incomplete data. 19 features were selected amongst demographic parameters and morphological characteristics on d1, 2 and 3. Two ML models, Random Forest Classifier, were trained: one to predict if a d3 embryo will reach blastocyst stage, a second to predict the quality of a future blastocyst from d1, d2 and d3 characteristics. The blastocyst prediction model was trained on 24543 embryos (3204 cycles) which survived d3 (9976 blastocysts – 40,6%, 14567 no-blastocysts – 59,4%). The quality prediction model was trained on 8754 embryos (1332 cycles) which reach blastocyst stage. A high-quality blastocyst (HQB) is full, expanded or hatching, with ICM score of A or B and trophectoderm scored as A or B: 3436 HQB (40%), 5318 low-quality blastocysts (LQB, 60%). The two Random Forest Classifiers were trained on 80% of the dataset and blind tested on the remaining 20%. Their hyperparameters (not learned on data) were fine-tuned with a grid search. The first model determined if a d3 embryo would become blastocyst with 77% of accuracy on test data. Precision and recall were high, both at 77% and AUC reached 0.61. The model was equally performant to classify embryos as blastocyst or as no-blastocyst. Assuming that the d3 embryo will become blastocyst, the second model classified it as HQB or LQB with an accuracy of 69%. Precision, recall and AUC were respectfully 67%, 65% and 0.64. By combining the two models, the quality of a future blastocyst could be determined from the third day of its development.
更多查看译文
关键词
future blastocyst development,machine learning
AI 理解论文
溯源树
样例
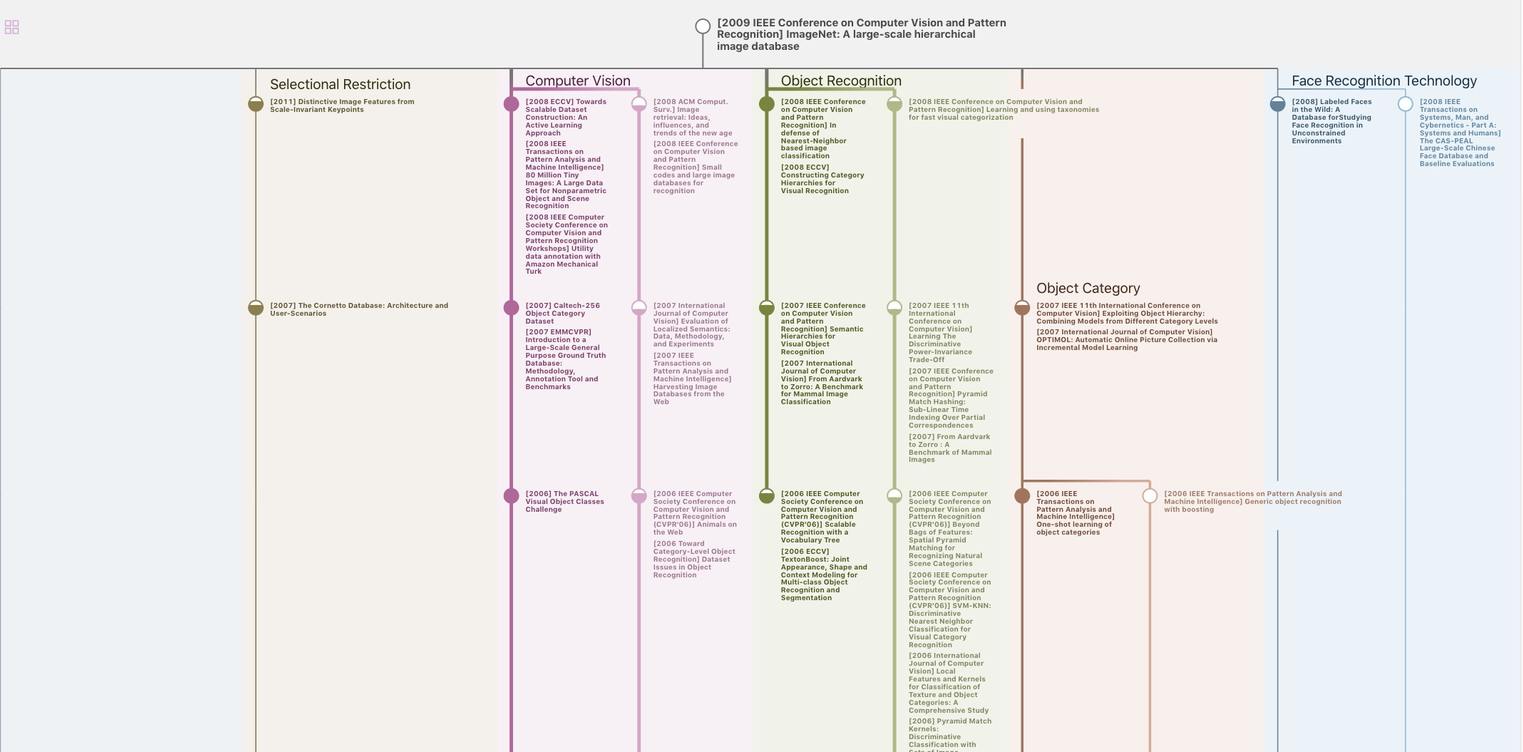
生成溯源树,研究论文发展脉络
Chat Paper
正在生成论文摘要