Memory-augmented meta-learning framework for session-based target behavior recommendation
WORLD WIDE WEB-INTERNET AND WEB INFORMATION SYSTEMS(2022)
摘要
Session-based recommendation aims to predict the next item to be interacted by a specific type of behavior (e.g., click or purchase) within a session. However, the main challenge comes from the lack of interactions in the target behavior. Despite state-of-the-art approaches aim to alleviate this issue by incorporating auxiliary behaviors through multi-behavior modeling, they are still weak in supporting cold-start recommendation due to the limited generalization ability. Having witnessed the effectiveness of meta-optimized models for few-shot learning, in this paper, we propose a memory-augmented meta-learning framework for session-based target behavior recommendation. It adopts meta-learning to learn well-generalized global sharing initialization parameters for all sessions, and derives personalized local parameters for each session through fine-tuning. Particularly, we first extract multi-behavior characteristics to derive dynamic user intentions within a session. Then we apply soft-clustering in meta-learning based on well-designed memory structures, so that multi-behavior sessions with similar intention could share related knowledge. The experimental results on two datasets show that our MMFSR model effectively outperforms the state-of-the-art methods.
更多查看译文
关键词
Session-based recommendation,Multi-behavior modeling,Meta learning,Heterogeneous network
AI 理解论文
溯源树
样例
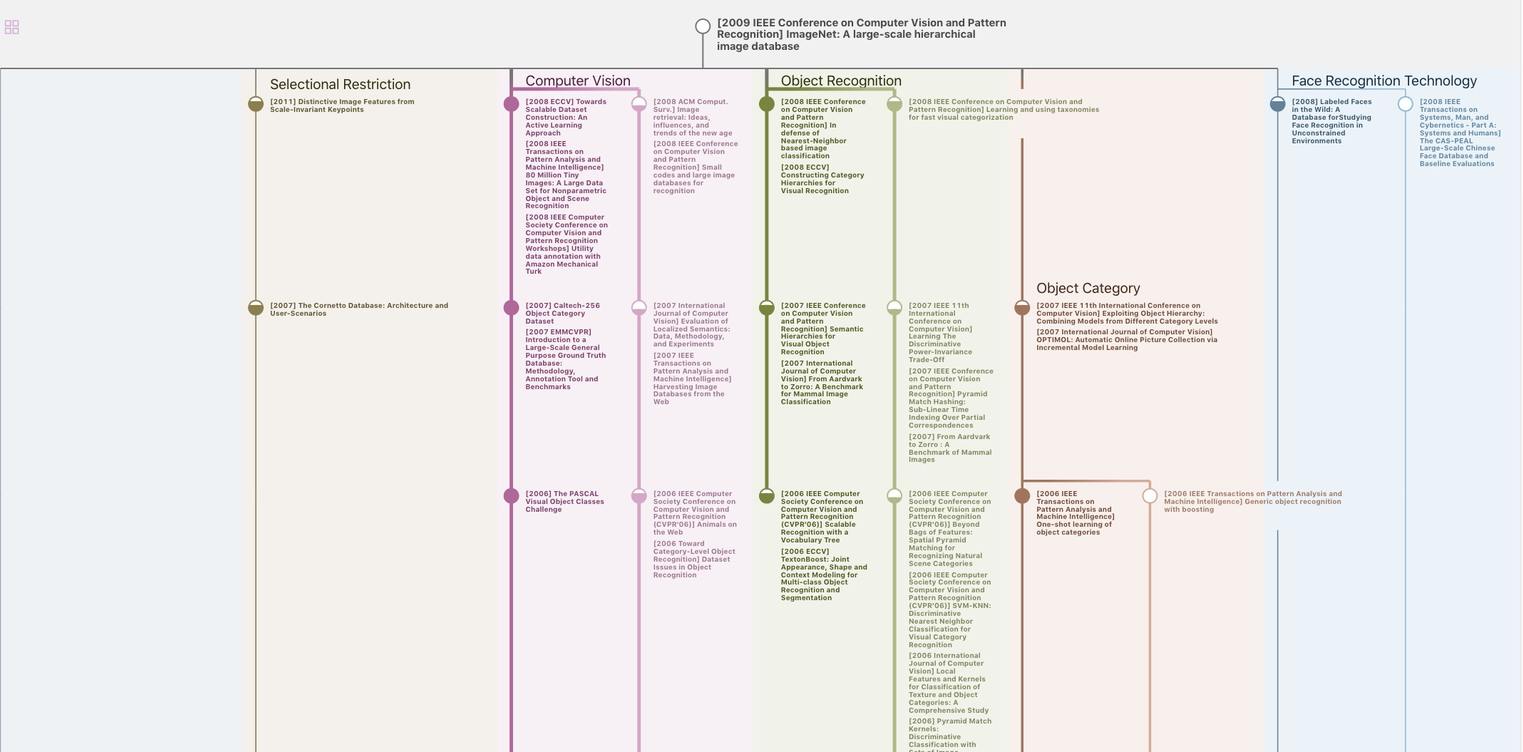
生成溯源树,研究论文发展脉络
Chat Paper
正在生成论文摘要