Dual Discriminator Weighted Mixture Generative Adversarial Network for image generation
JOURNAL OF AMBIENT INTELLIGENCE AND HUMANIZED COMPUTING(2022)
摘要
Image generation is a hot topic in the field of machine learning and computer vision. As a representative of its algorithm, the Generative Adversarial Network (GAN) has the problem of mode collapse in practice. The proposed Dual Discriminator Weighted Mixture Generative Adversarial Network (D2WMGAN) approach can cope with this problem. On the one hand, the D2WMGAN uses the mixed distribution of multiple generators to approximate the real distribution, in order to prevent the extreme situation that multiple generators learn the same distribution and generate the same class of samples, with a classifier to play games with generators to make different generators learn different distributions. On the other hand, the objective function of D2WMGAN weights the Kullback–Leibler (KL) divergence and the reverse KL divergence, and uses their complementary characteristics to improve the quality and diversity of samples from the generators. Then, the theoretical conditional optimality of the D2WMGAN is proved theoretically, which shows that multiple generators can learn the real data distribution in the case of the optimal discriminator and classifier. Finally, extensive experiments are conducted on a large amount of synthetic data and real-world large-scale datasets (such as, CIFAR-10 and MNIST), and the commonly used GAN evaluation indicators (Wasserstein distance, JS divergence, Inception score, and Frechet Inception Distance) are introduced for comparative analysis. Experimental results show that the proposed D2WMGAN approach can better learn multiple mode data, generate rich realistic samples, and effectively solve the problem of mode collapse.
更多查看译文
关键词
Generative Adversarial Network, Dual Discriminator Generative Adversarial Network, Mixture Generative Adversarial Network, Mode collapse
AI 理解论文
溯源树
样例
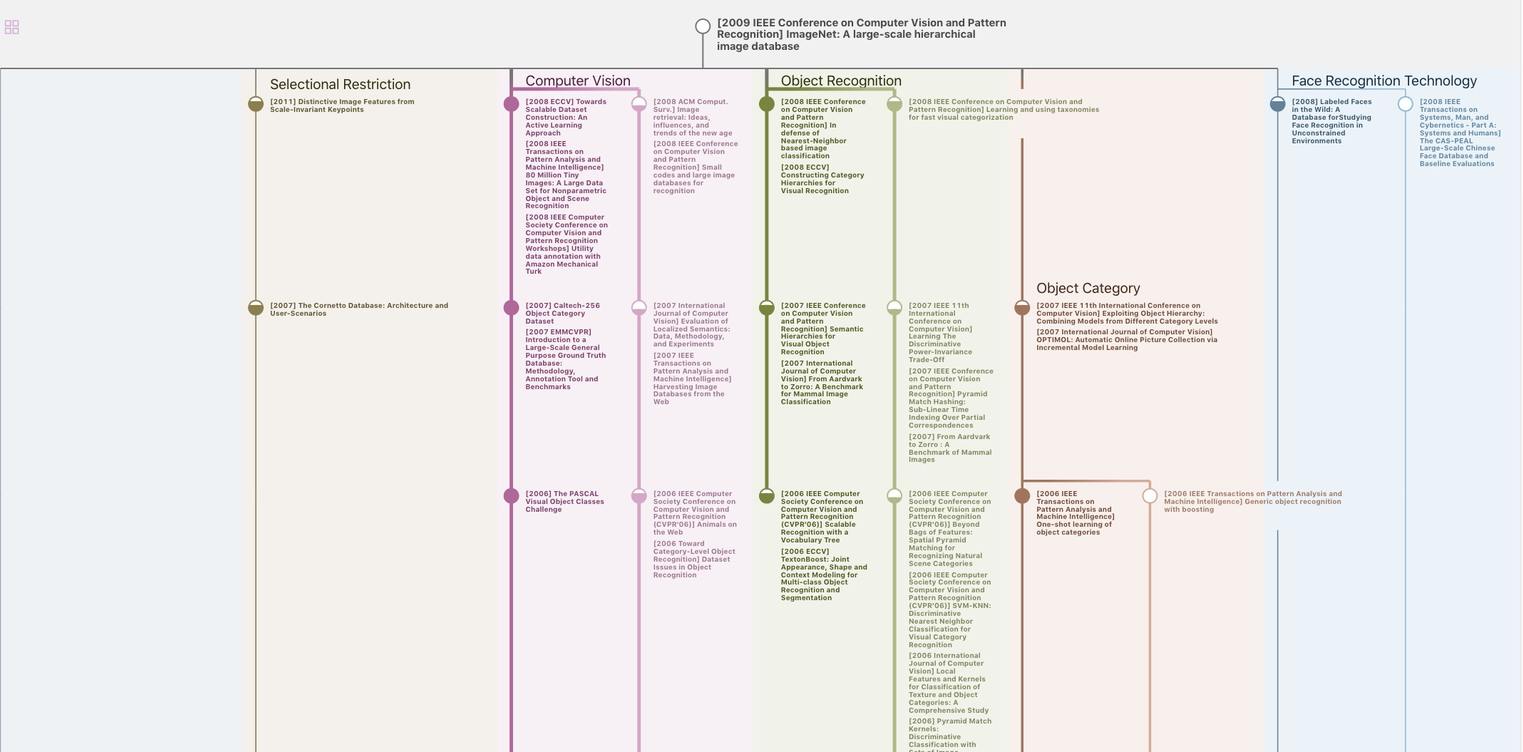
生成溯源树,研究论文发展脉络
Chat Paper
正在生成论文摘要