Few2Decide: towards a robust model via using few neuron connections to decide
International Journal of Multimedia Information Retrieval(2022)
摘要
Researches have shown that image classification networks are vulnerable to adversarial examples, which seriously limits their application in safely critical scenarios. Existing defense methods usually employ adversarial training or adjust the network structure to resist adversarial attack. Although these defense methods can improve the model robustness to some extent, they often significantly decrease the accuracy on the clean data and bring additional computational cost. In this work, we analyze the impact of adversarial example on neuron connections and propose a Few2Decide method to train a robust model by dropping part of non-robust connections in the fully connected layer. Our model can get high perturbed data accuracy without increasing trainable parameters, meanwhile, get high clean data accuracy. Experimental results prove that our method can provide a robust model and achieve state-of-the-art performance on the CIFAR-10 dataset. Specifically, our Few2Decide method achieves 73.01
更多查看译文
关键词
Few2Decide, Deep neural network, Adversarial attack and defense, Robustness
AI 理解论文
溯源树
样例
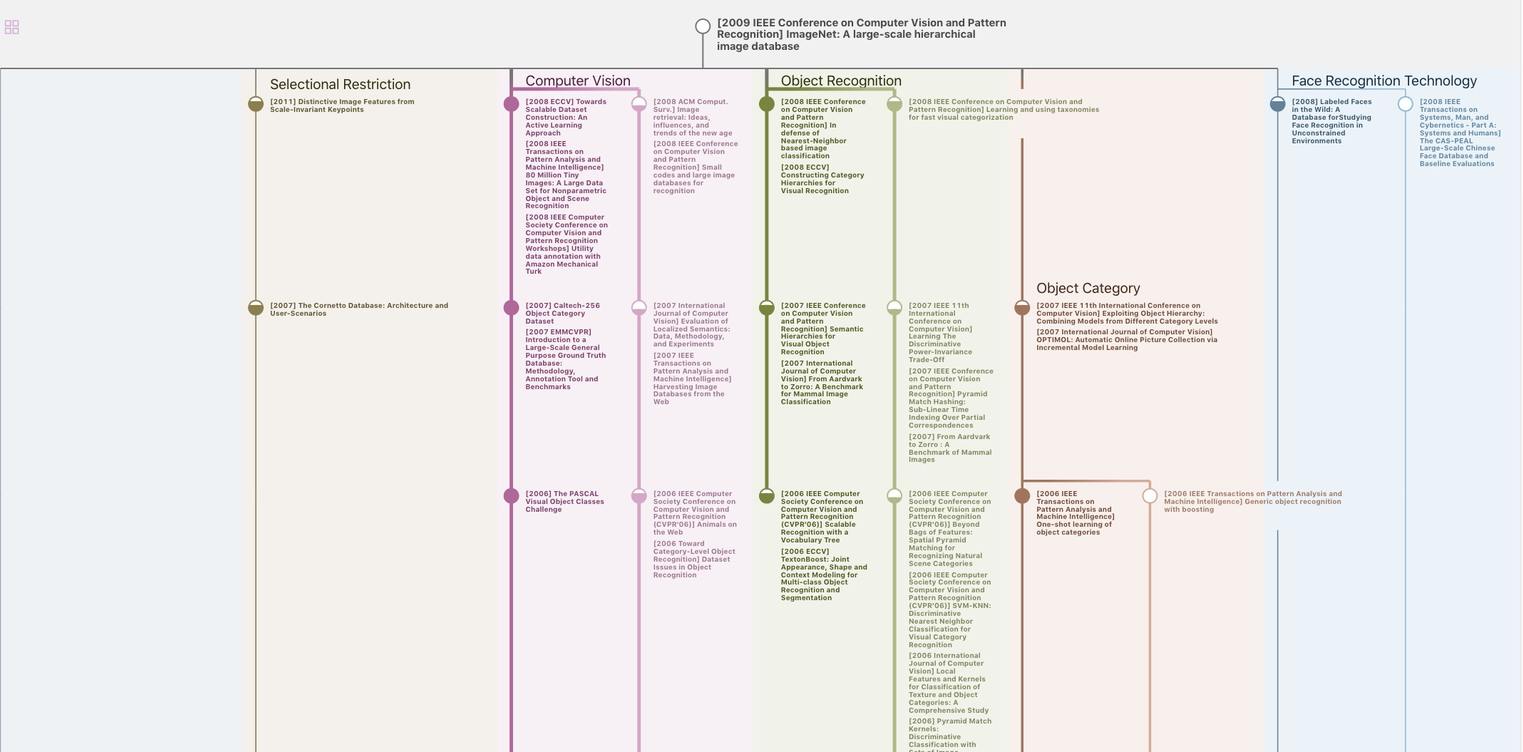
生成溯源树,研究论文发展脉络
Chat Paper
正在生成论文摘要