Online streaming feature selection based on neighborhood rough set
APPLIED SOFT COMPUTING(2021)
摘要
Feature selection is considered as a necessary and significant pre-processing step in many fields, especially in machine learning. However, in some real problems, in which features flow one by one, many existing approaches do not work well on the online streaming features, and most online streaming feature selection (OSFS) methods face the challenge of requiring domain knowledge before setting optimal parameters in advance. Therefore, an effective feature selection method for online streaming features, named OFS-Gapknn, is proposed in this paper. A new neighborhood rough set relation is firstly defined, which combines the advantages of both the k-nearest and the Gap neighborhood. The proposed neighborhood relation can not only work well on the unevenly distributed sample space, but also need not any parameters and domain knowledge. Then, the relevance and redundancy features are analyzed by using the dependency based on the neighborhood rough set. Finally, one of the optimal feature subsets is obtained. To validate the effectiveness of the proposed algorithm, four traditional methods and three OSFS methods are compared with it on 11 datasets. Experimental results indicate the dominance and significance of the proposed method. (C) 2021 Published by Elsevier B.V.
更多查看译文
关键词
Feature selection, Online streaming feature selection, Neighborhood rough set, K-nearest neighborhood, Gap neighborhood
AI 理解论文
溯源树
样例
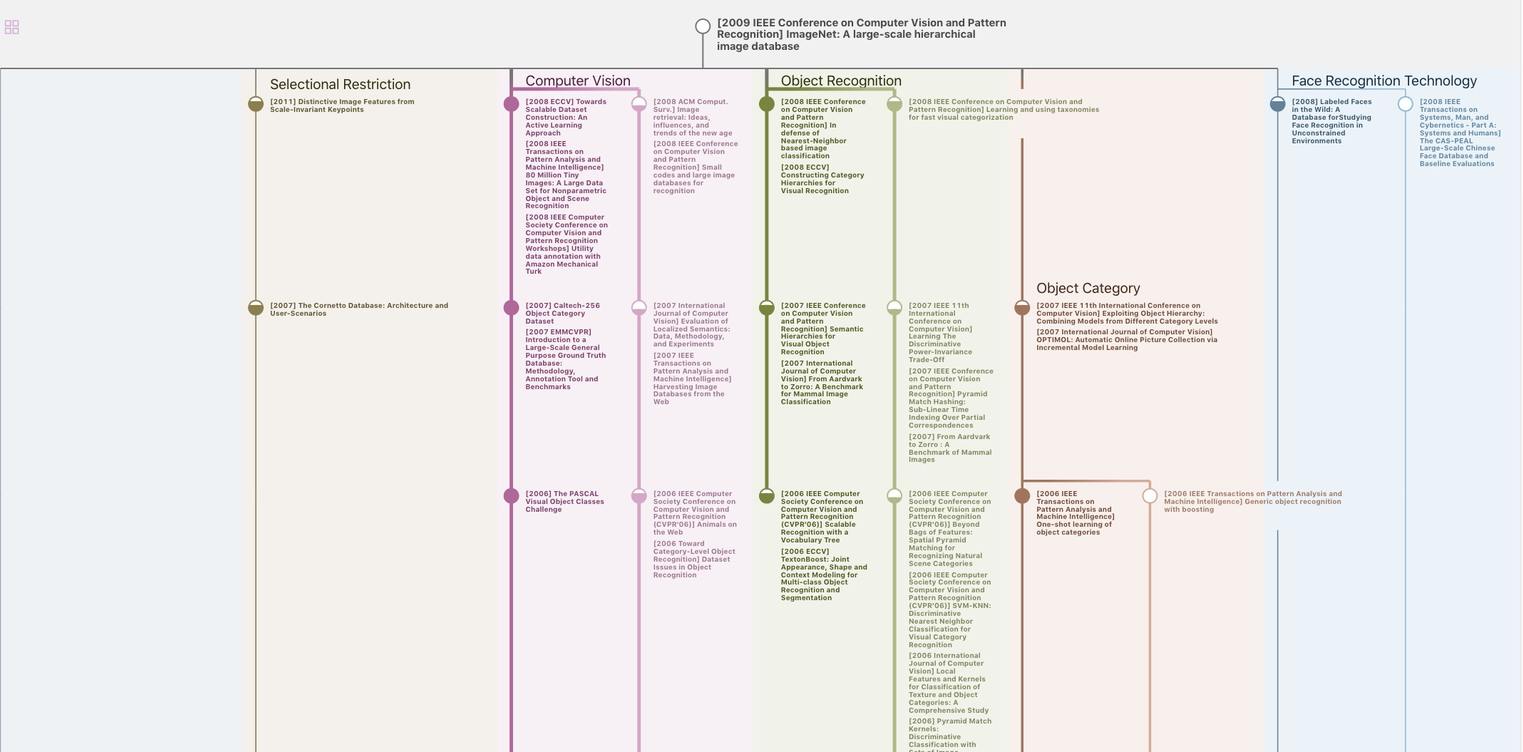
生成溯源树,研究论文发展脉络
Chat Paper
正在生成论文摘要