Knowledge based natural answer generation via masked-graph transformer
World Wide Web(2021)
摘要
Natural Answer Generation on Knowledge Base (NAG-KB), which generates natural answer sentences for the given question, has received much attention in recent years. Compared with traditional QA systems, NAG could offer specific entities fluently and naturally, which is more user-friendly in the real world. However, existing NAG systems usually utilize simple retrieval and embedding mechanism, which is hard to tackle complex questions. They suffer issues containing knowledge insufficiency, entity ambiguity, and especially poor expressiveness during generation. To address these challenges, we propose an improved knowledge extractor containing post disambiguation and simplifying strategy to retrieve supporting graphs from KB, an masked-graph transformer to encode the supporting graph, which introduce special vertex setting, communication path calculation and mask mechanism. Moreover we design a multi-task training combining classification and sequence decoding jointly. In summary, we propose a framework called G-NAG in this paper, including a knowledge extractor, an incorporating encoder, and an multi-task generator. Experimental results on two complex QA datasets demonstrate the efficiency of G-NAG compared with state-of-the-art NAG systems and transformer baselines.
更多查看译文
关键词
Question answering, Natural answer generation, Graph attention network, Mask mechanism
AI 理解论文
溯源树
样例
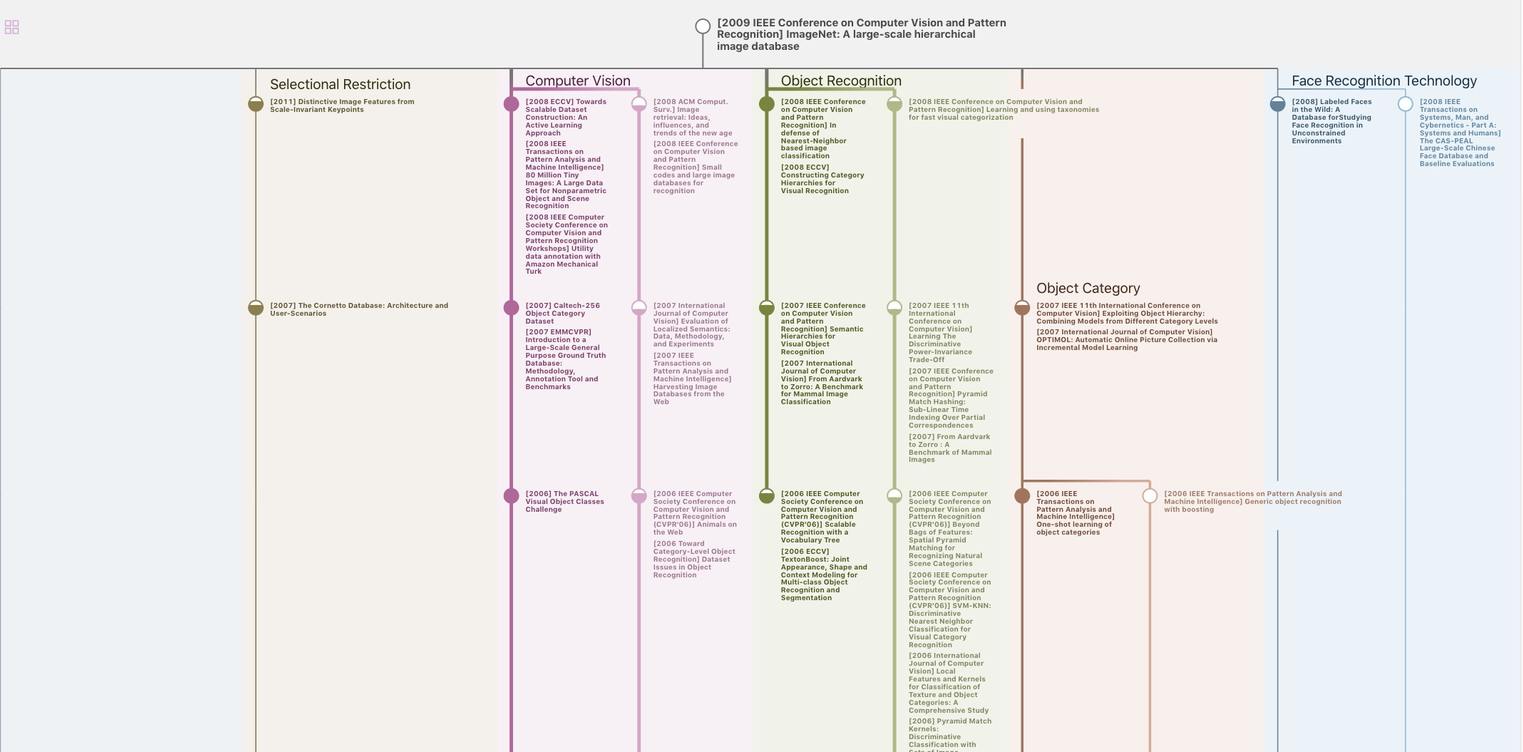
生成溯源树,研究论文发展脉络
Chat Paper
正在生成论文摘要