Data-driven selection of the number of change-points via error rate control
JOURNAL OF THE AMERICAN STATISTICAL ASSOCIATION(2023)
摘要
In multiple change-point analysis, one of the main difficulties is to determine the number of change-points. Various consistent selection methods, including the use of Schwarz information criterion and cross-validation, have been proposed to balance the model fitting and complexity. However, there is lack of systematic approaches to provide theoretical guarantee of significance in determining the number of changes. In this paper, we introduce a data-adaptive selection procedure via error rate control based on order-preserving sample-splitting, which is applicable to most existing change-point methods. The key idea is to construct a series of statistics with global symmetry property and then utilize the symmetry to derive a data-driven threshold. Under this general framework, we are able to rigorously investigate the false discovery proportion control, and show that the proposed method controls the false discovery rate (FDR) asymptotically under mild conditions while retaining the true change-points. Numerical experiments indicate that our selection procedure works well for many change-detection methods and is able to yield accurate FDR control in finite samples. Keywords: Empirical distribution; False discovery rate; Multiple change-point model; Sample-splitting; Symmetry; Uniform convergence.
更多查看译文
AI 理解论文
溯源树
样例
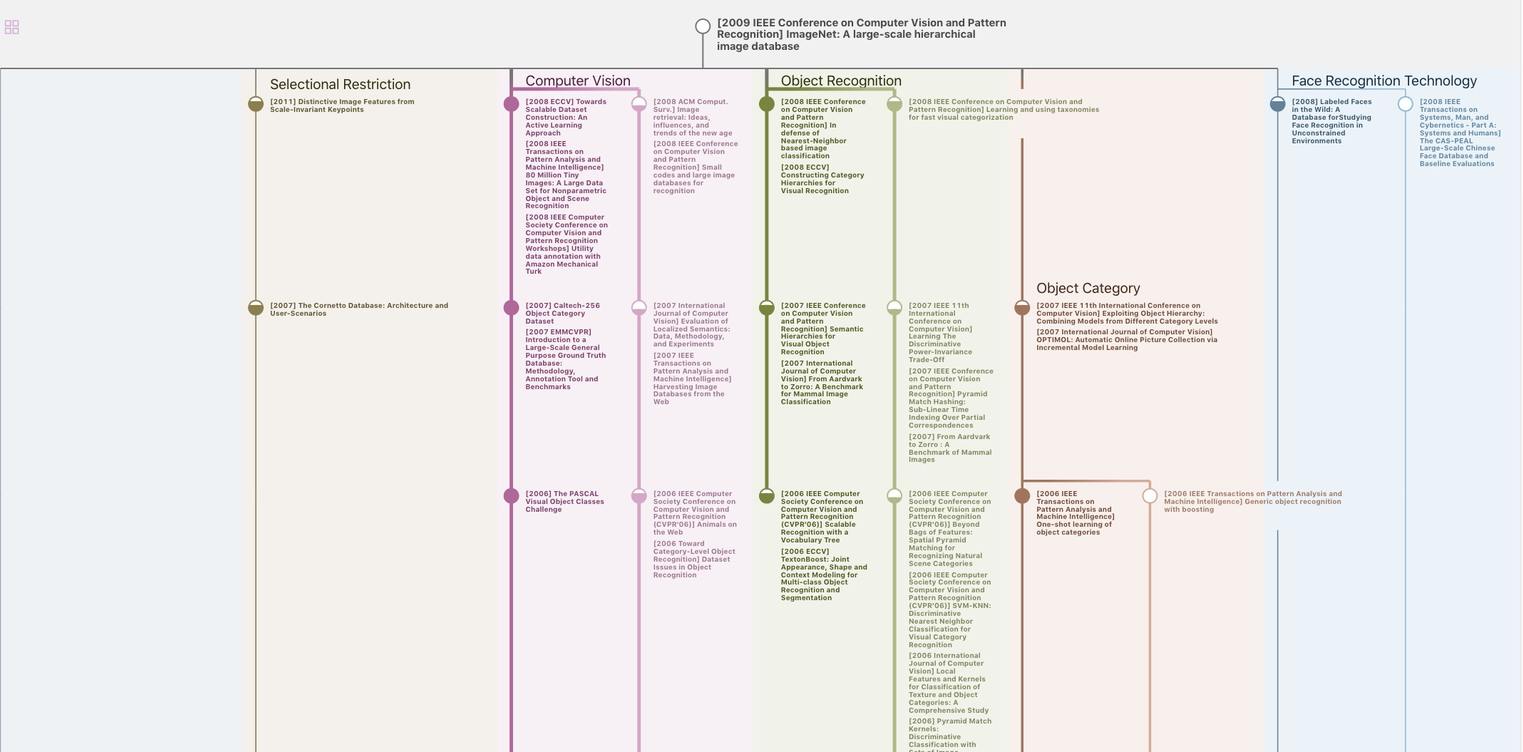
生成溯源树,研究论文发展脉络
Chat Paper
正在生成论文摘要