Status Recognition Using Pre-Trained YOLOv5 for Sustainable Human-Robot Collaboration (HRC) System in Mold Assembly
SUSTAINABILITY(2021)
摘要
Molds are still assembled manually because of frequent demand changes and the requirement for comprehensive knowledge related to their high flexibility and adaptability in operation. We propose the application of human-robot collaboration (HRC) systems to improve manual mold assembly. In the existing HRC systems, humans control the execution of robot tasks, and this causes delays in the operation. Therefore, we propose a status recognition system to enable the early execution of robot tasks without human control during the HRC mold assembly operation. First, we decompose the mold assembly operation into task and sub-tasks, and define the actions representing the status of sub-tasks. Second, we develop status recognition based on parts, tools, and actions using a pre-trained YOLOv5 model, a one-stage object detection model. We compared four YOLOv5 models with and without a freezing backbone. The YOLOv5l model without a freezing backbone gave the optimal performance with a mean average precision (mAP) value of 84.8% and an inference time of 0.271 s. Given the success of the status recognition, we simulated the mold assembly operations in the HRC environment and reduced the assembly time by 7.84%. This study improves the sustainability of the mold assembly from the point of view of human safety, with reductions in human workload and assembly time.
更多查看译文
关键词
human-robot collaboration, transfer learning, status recognition, mold assembly
AI 理解论文
溯源树
样例
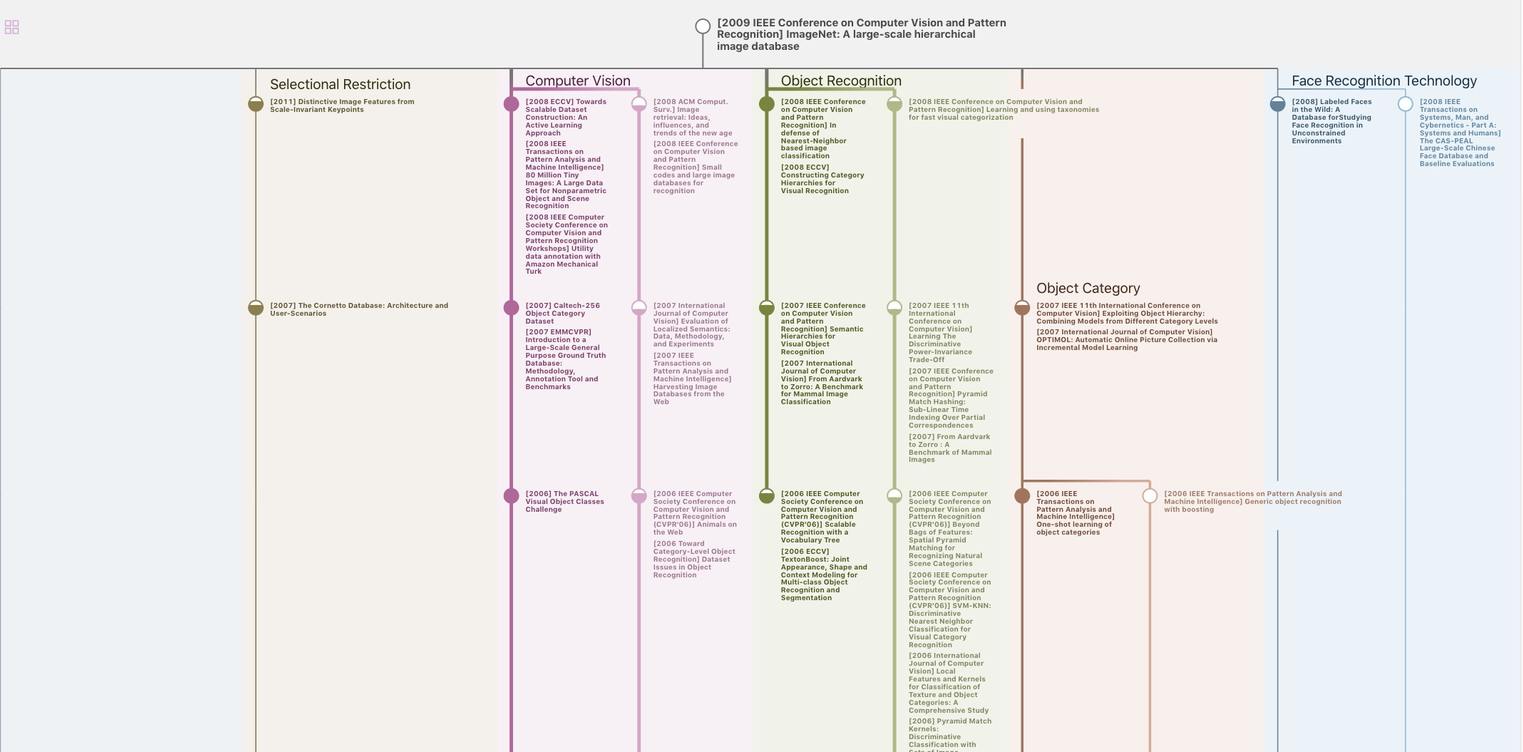
生成溯源树,研究论文发展脉络
Chat Paper
正在生成论文摘要