An Improved Hybrid Transfer Learning-Based Deep Learning Model for PM2.5 Concentration Prediction
APPLIED SCIENCES-BASEL(2022)
摘要
With the improvement of the living standards of the residents, it is a very important and challenging task to continuously improve the accuracy of PM2.5 (particulate matter less than 2.5 mu m in diameter) prediction. Deep learning-based networks, such as LSTM and CNN, have achieved good performance in recent years. However, these methods require sufficient data to train the model. The performance of these methods is limited for the sites where the data is lacking, such as the newly constructed monitoring sites. To deal with this problem, an improved deep learning model based on the hybrid transfer learning strategy is proposed for predicting PM2.5 concentration in this paper. In the proposed model, the maximum mean discrepancy (MMD) is used to select which station in the source domain is most suitable for migration to the target domain. An improved dual-stage two-phase (DSTP) model is used to extract the spatial-temporal features of the source domain and the target domain. Then the domain adversarial neural network (DANN) is used to find the domain invariant features between the source and target domains by domain adaptation. Thus, the model trained by source domain site data can be used to assist the prediction of the target site without degradation of the prediction performance due to domain drift. At last, some experiments are conducted. The experimental results show that the proposed model can effectively improve the accuracy of the PM2.5 prediction at the sites lacking data, and the proposed model outperforms most of the latest models.
更多查看译文
关键词
PM2.5 prediction, transfer learning, domain adversarial neural network, dual-stage two-phase model
AI 理解论文
溯源树
样例
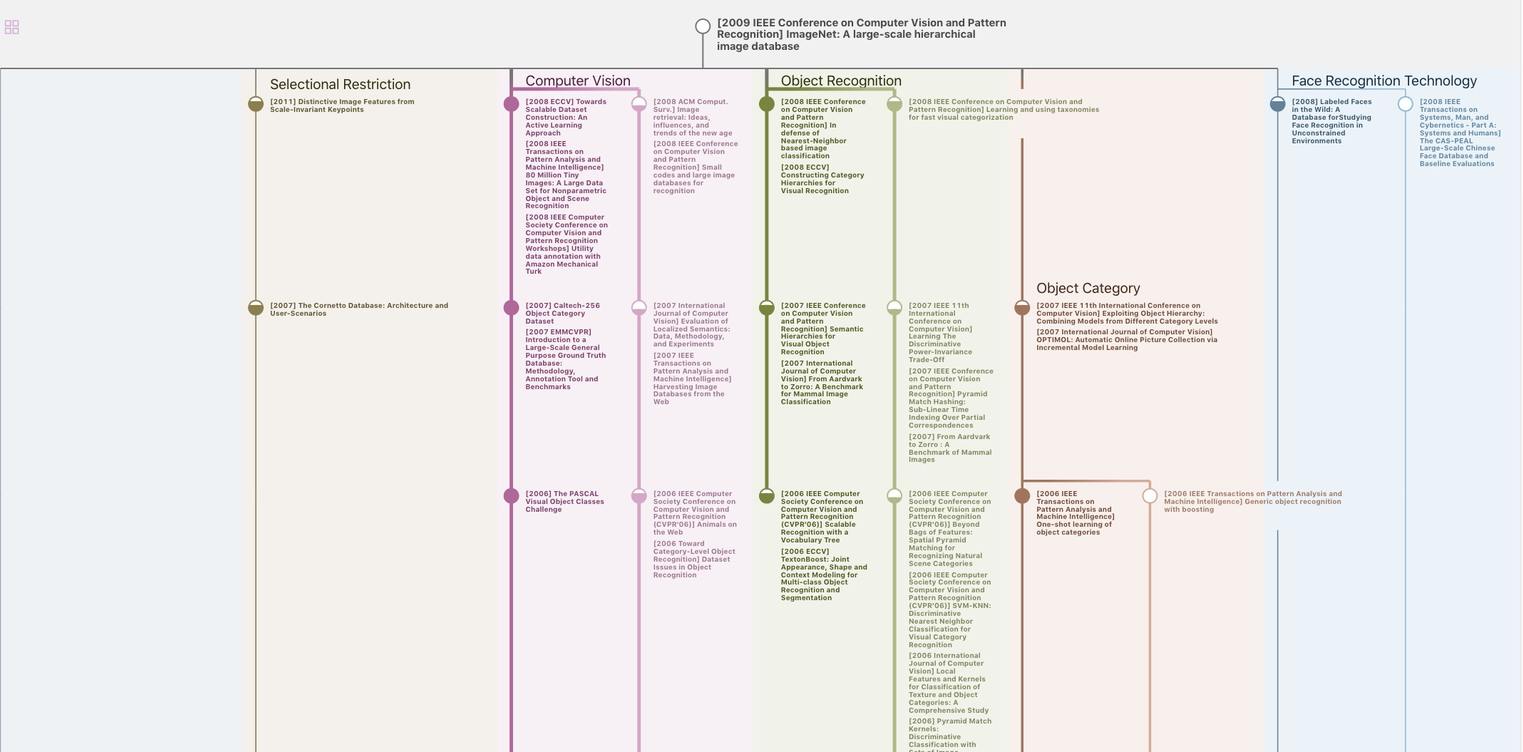
生成溯源树,研究论文发展脉络
Chat Paper
正在生成论文摘要