Machine Learning-Assisted Closed-Control Loops for Beyond 5G Multi-Domain Zero-Touch Networks
Journal of Network and Systems Management(2022)
摘要
End-to-End (E2E) services in beyond 5G (B5G) networks are expected to be built upon resources and services distributed in multi-domain, multi-technology environments. In such scenarios, key challenges around multi-domain management and collaboration need to be tackled. ETSI Zero-touch network and Service Management (ZSM) architectural framework provides the structure and methods for effectively delivering E2E network services. ZSM pursues cross-domain automation with minimum human intervention through two main enablers: Closed Control Loop (CCL) and Artificial Intelligence (AI). In this work, we propose a multi-domain ZSM-based architecture aiming at B5G scenarios where several per-domain CCLs leverage Machine Learning (ML) methods to collaborate in E2E service management tasks. We instantiate the architecture in the use case scenario of multi-domain automated healing of Dynamic Adaptive Streaming over HTTP (DASH) video services. We present two ML-assisted techniques, first to estimate a Service Level Agreement (SLA) violation through a Edge-based Quality of Experience (QoE) Probe, and second to identify the root cause at the core transport network. Results from the experimental evaluation in an emulation environment using real mobile network traces point to the potential benefits of applying ML techniques for QoS-to-QoE estimation at Multi-Access Edge Computing facilities and correlation to faulty transport network links. Altogether, the work contributes towards a vision of ML-based sandbox environments in the spirit of E2E service and network digital twins towards the realization of automated, multi-domain CCLs for B5G.
更多查看译文
关键词
Beyond 5G Networks,End-to-End Network Services,Network and Service Management,Artificial Intelligence,Self-healing
AI 理解论文
溯源树
样例
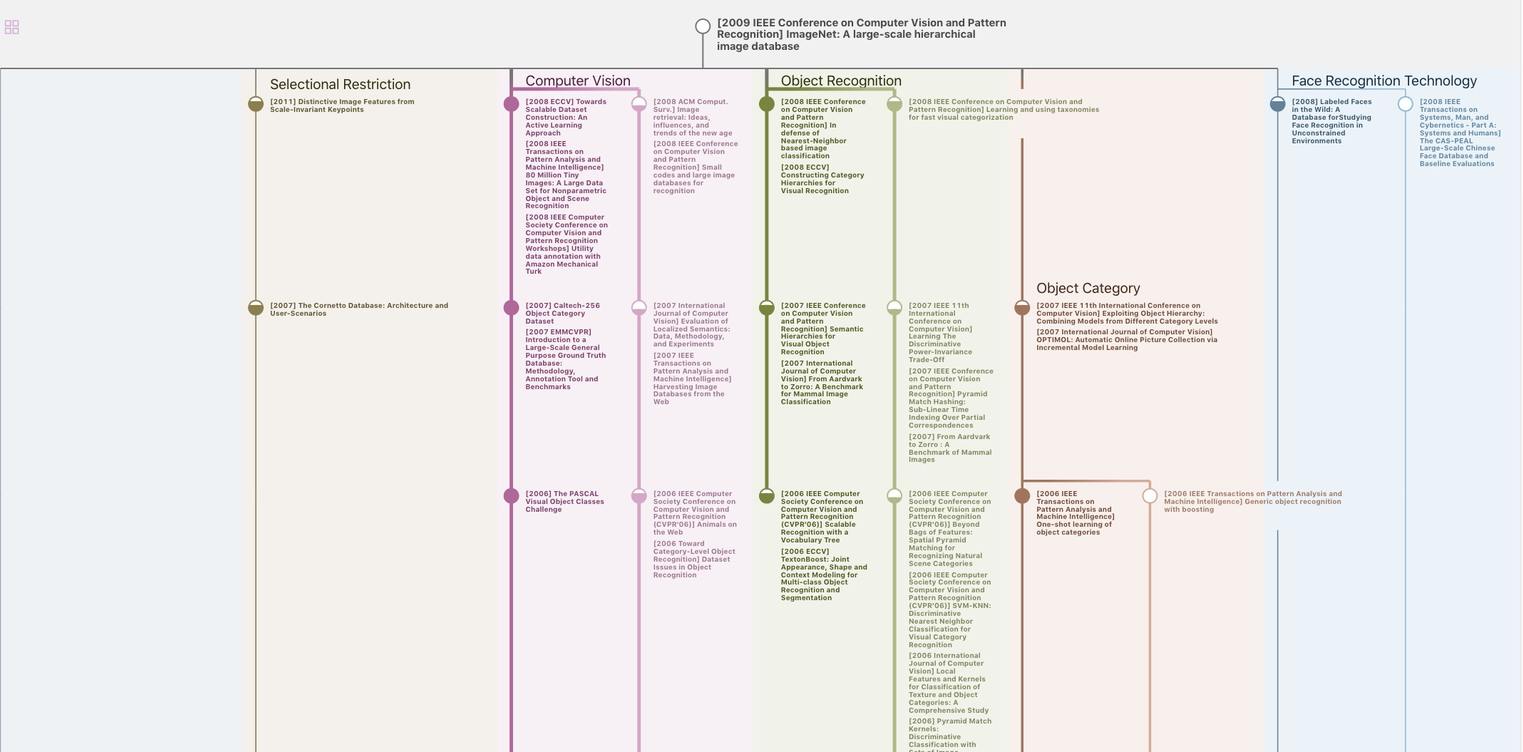
生成溯源树,研究论文发展脉络
Chat Paper
正在生成论文摘要