Detecting Lower MMSE Scores in Older Adults Using Cross-Trial Features From a Dual-Task With Gait and Arithmetic
IEEE ACCESS(2021)
摘要
The Mini-Mental State Examination (MMSE) is widely used in clinics to screen for low cognitive status. However, it is limited in that it requires examiners to be present; and has fixed questions that constrain its repeated use. Thus, the MMSE cannot be used as a daily assessment to facilitate early detection of cognitive impairment. To address this issue, we developed an automated system to detect older adults with lower MMSE scores by analyzing performance during a dual task involving stepping and calculation, which can be used repeatedly because its questions were randomly created. Leveraging this advantage, this paper proposes a learning-based method to detect subjects with lower MMSE scores using multiple trials with the dual-task system. We investigated various patterns for effectively combining the features acquired during multiple continuous trials, and analyzed the sensitivity of the number N of trials on detection performance to find the optimal N via experiments. We compared our approach with previous methods and demonstrated the superiority of our strategy. Using the cross-trial feature, our approach achieved an overall performance (sensitivity + specificity) as high as 1.79 for detecting older adults whose MMSE score is equal to or less than 23 (indicate a relatively high probability of dementia), and 1.75 for detecting older adults whose MMSE score is equal to or less than 27 (indicative of a relatively high probability of mild cognitive impairment (MCI)).
更多查看译文
关键词
Task analysis,Feature extraction,Dementia,Arithmetic,Standards,Sensitivity,Monitoring,Cognitive impairment,dementia,dual-task,machine learning,MCI,MMSE
AI 理解论文
溯源树
样例
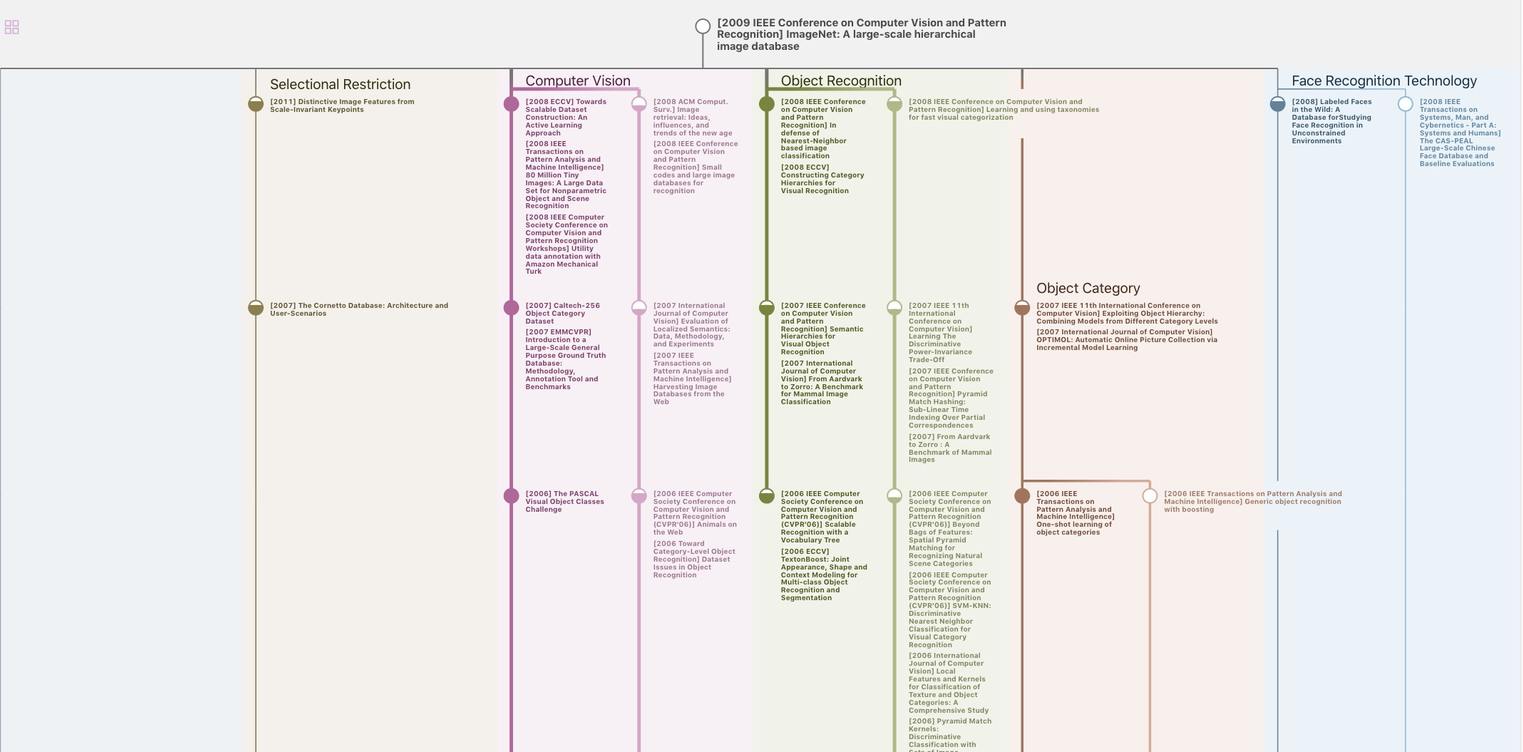
生成溯源树,研究论文发展脉络
Chat Paper
正在生成论文摘要