A COMPARISON OF STATIC, DYNAMIC AND MACHINE LEARNING MODELS IN PREDICTING THE FINANCIAL DISTRESS OF CHINESE FIRMS
ROMANIAN JOURNAL OF ECONOMIC FORECASTING(2022)
摘要
As recent studies have begun to pay increasing attention to financial distress prediction (FDP), this study compares the performance of static, dynamic and machine learning (ML) models in predicting the financial distress of firms. Balanced and imbalanced datasets of Chinese listed firms that span the years 1992 to 2018 (27 years) and contain 29,000 firm-year observations are used for the comparison. Results show an incremental improvement in the FDP accuracy of these models, with the random forest (RF) ML model demonstrating the best performance, followed by the Dynamic Hazard model and the static model. Including the growth variable improved the predictive ability of these models. Whilst all these models demonstrated a decreasing prediction accuracy in the imbalanced dataset, a weaker trend was observed for ML models. These results support the debate regarding the FDP superiority of computational methods. The findings provide new evidence that ML models can predict financial distress more accurately as compared to conventional models in the Chinese context.
更多查看译文
关键词
financial distress prediction, static, dynamic, machine learning, growth, China
AI 理解论文
溯源树
样例
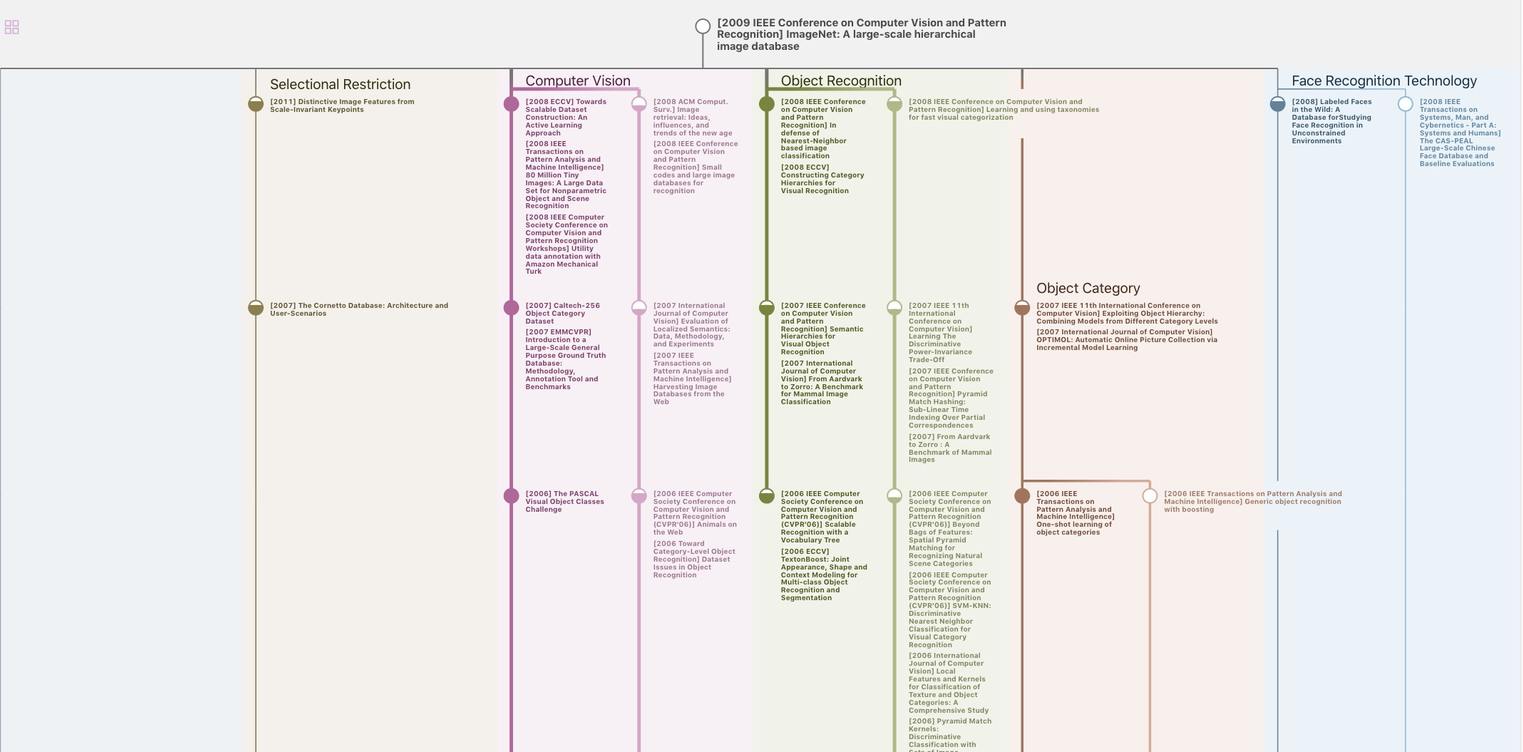
生成溯源树,研究论文发展脉络
Chat Paper
正在生成论文摘要