A data-driven approach to approximate the correlation functions in cluster variation method
MODELLING AND SIMULATION IN MATERIALS SCIENCE AND ENGINEERING(2022)
摘要
The cluster variation method is one of the thermodynamic models used to calculate phase diagrams considering short range order (SRO). This method predicts the SRO values through internal variables referred to as correlation functions (CFs), accurately up to the cluster chosen in modeling the system. Determination of these CFs at each thermodynamic state of the system requires solving a set of nonlinear equations using numerical methods. In this communication, a neural network model is proposed to predict the values of the CFs. This network is trained for the bcc phase under tetrahedron approximation for both ordering and phase separating systems. The results show that the network can predict the values of the CFs accurately and thereby Helmholtz energy and the phase diagram with significantly less computational burden than that of conventional methods used.
更多查看译文
关键词
short range order, correlation functions, neural network model, cluster variation method, tetrahedron approximation
AI 理解论文
溯源树
样例
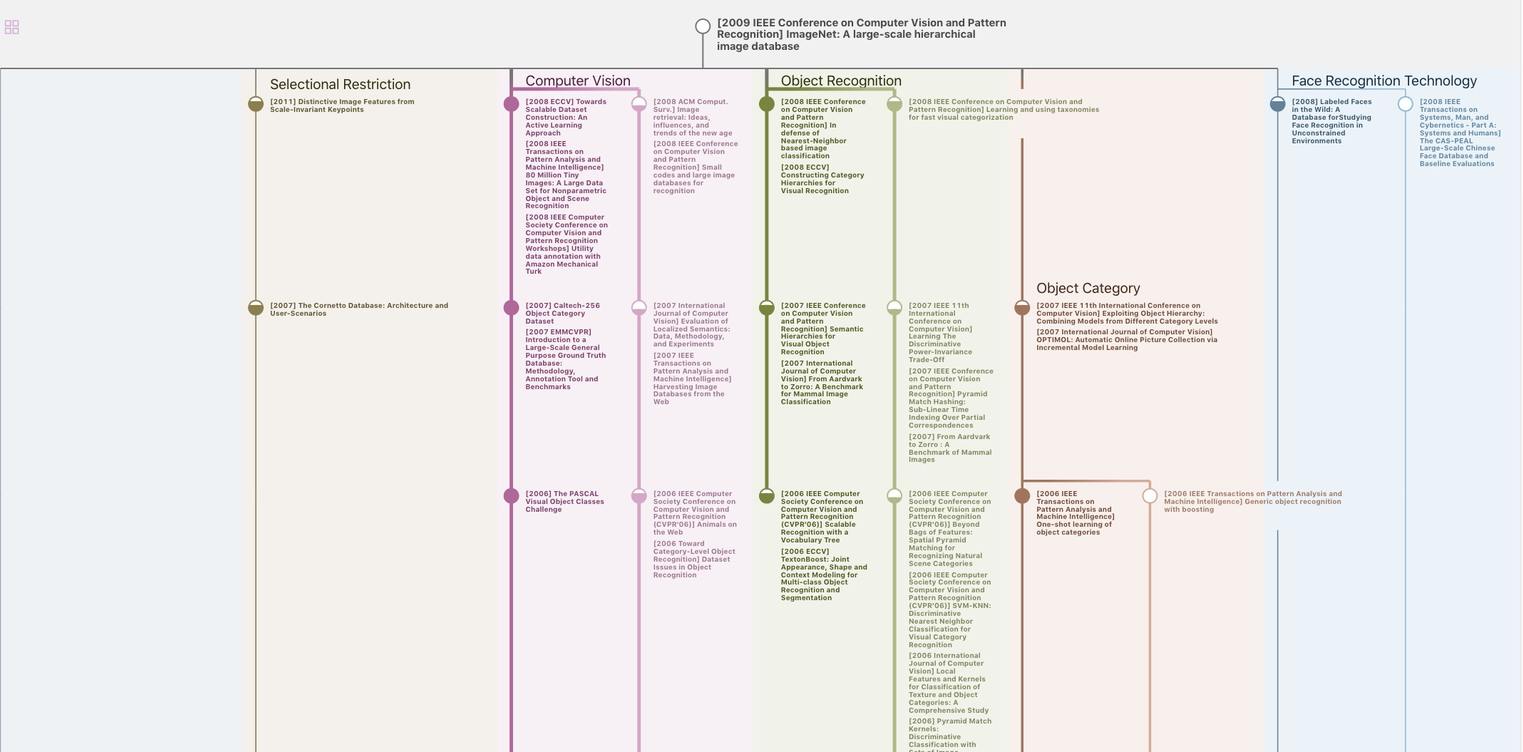
生成溯源树,研究论文发展脉络
Chat Paper
正在生成论文摘要