Cognitive Knowledge-aware Social Recommendation Via Group-enhanced Ranking Model.
Cognitive computation(2022)
摘要
Cognitive inspired recommendation systems have attracted increasing attention in recent years, aiming at fitting user ratings on certain items. However, the performance of recommendation approaches has been limited due to the sparsity and ambiguity of cognitive knowledge user-item ratings. Top-k recommendation has therefore been addressed and has become one of the most popular research areas. The goal of top-k recommendation is to capture the relative preferences of users and fit the optimal ranking list of items. Meanwhile, the development of social networks provides a new way to model user preferences to improve the accuracy and interpretation ability of cognition-aware recommendation models. To integrate user social information into top-k recommendation, we propose a group-enhanced ranking method based on matrix factorization. In our method, we first compute trust values between users based on user trust relationships. Then, we incorporate a trust matrix into the loss function with a social-based penalty term that reduces the distances between preference vectors of trusted users. Experimental results on two real datasets from Epinions and BaiduMovies show that the proposed method outperforms several state-of-the-art methods in terms of the normalized discounted cumulative gain (NDCG) value. Our model effectively improves the accuracy of social recommendations. We propose a novel cognitive knowledge-aware group-enhanced social recommendation method for item recommendation. The model modifies the loss function by considering the user trust relationship and group-enhanced ranking and significantly improves the performance of social recommendations.
更多查看译文
关键词
Recommendation System,Social Networks,Learning to Rank,Domain Knowledge
AI 理解论文
溯源树
样例
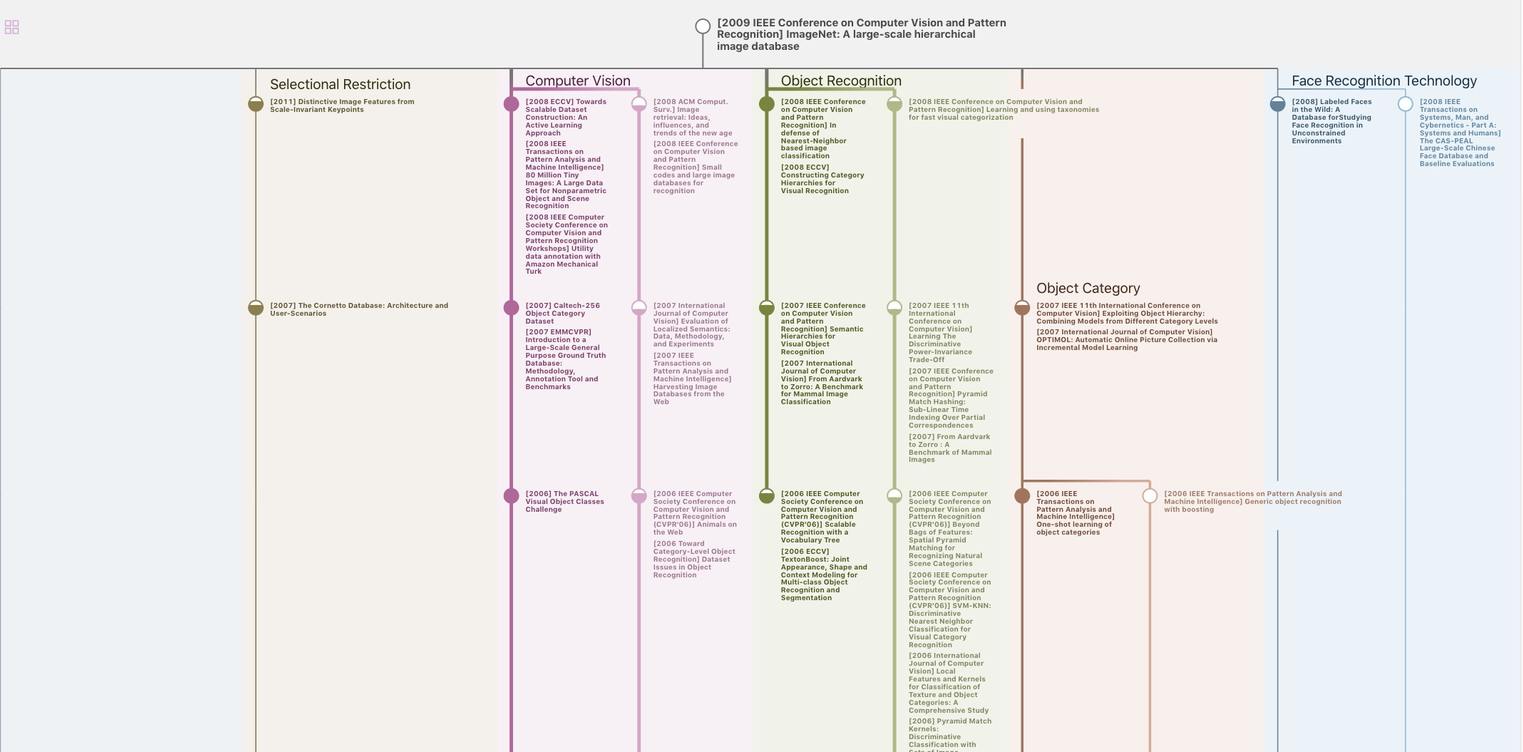
生成溯源树,研究论文发展脉络
Chat Paper
正在生成论文摘要