A multi process value-based reinforcement learning environment framework for adaptive traffic signal control
JOURNAL OF CONTROL AND DECISION(2023)
摘要
Realising adaptive traffic signal control (ATSC) through reinforcement learning (RL) is an important means to easetraffic congestion. This paper finds the computing power of the central processing unit (CPU) cannot fully usedwhen Simulation of Urban MObility (SUMO) is used as an environment simulator for RL. We propose a multi-process framework under value-basedRL. First, we propose a shared memory mechanism to improve exploration efficiency. Second, we use the weight sharing mechanism to solve the problem of asynchronous multi-process agents. We also explained the reason shared memory in ATSC does not lead to early local optima of the agent. We have verified in experiments the sampling efficiency of the 10-process method is 8.259 times that of the single process. The sampling efficiency of the 20-process method is 13.409 times that of the single process. Moreover, the agent can also converge to the optimal solution.
更多查看译文
关键词
Adaptive traffic signal control,Simulation of Urban MObility,multi-process,reinforcement learning,value-based
AI 理解论文
溯源树
样例
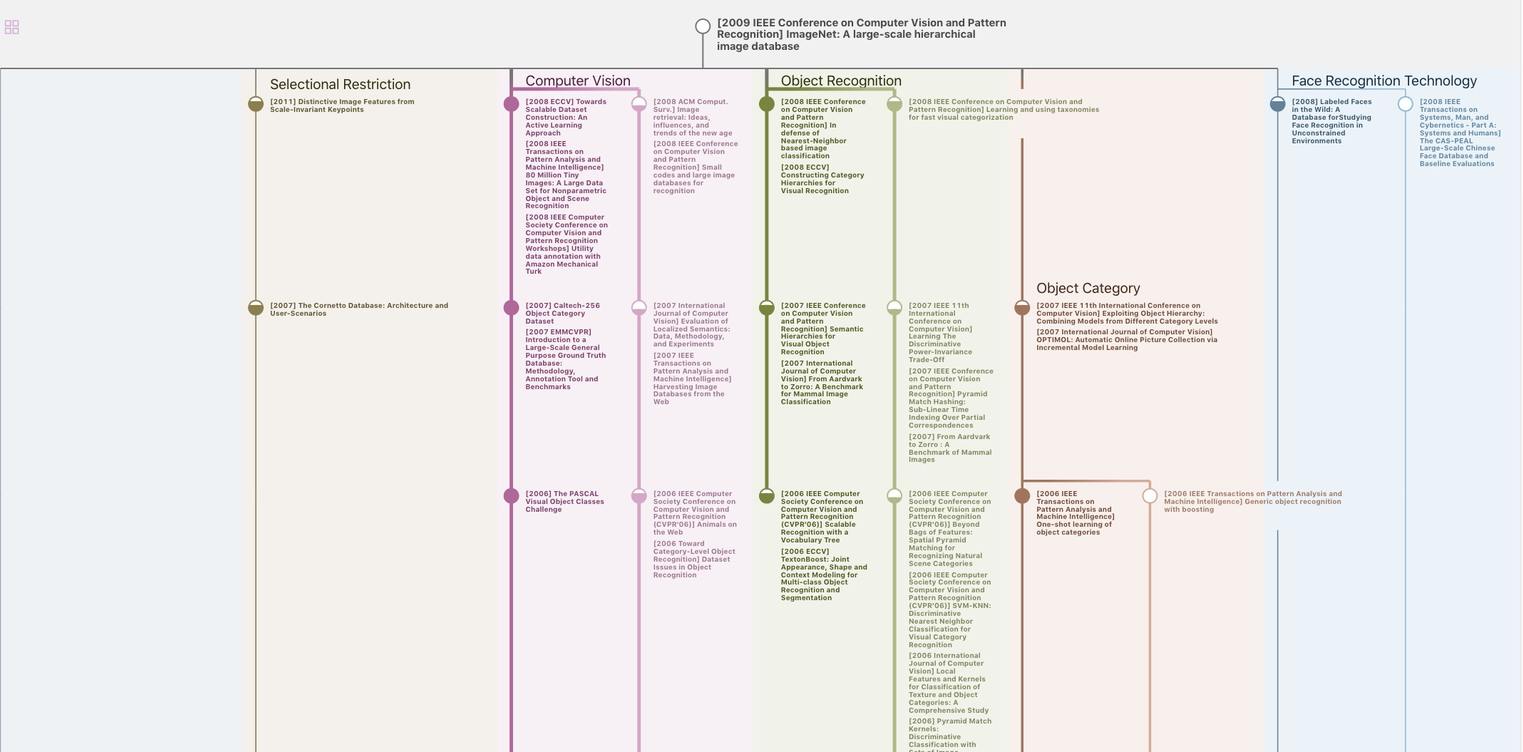
生成溯源树,研究论文发展脉络
Chat Paper
正在生成论文摘要