Bayesian adjustment for preferential testing in estimating infection fatality rates, as motivated by the covid-19 pandemic
ANNALS OF APPLIED STATISTICS(2022)
摘要
A key challenge in estimating the infection fatality rate (IFR), along with its relation with various factors of interest, is determining the total number of cases. The total number of cases is not known not only because not everyone is tested but also, more importantly, because tested individuals are not representative of the population at large. We refer to the phenomenon whereby infected individuals are more likely to be tested than noninfected individuals as "preferential testing." An open question is whether or not it is possible to reliably estimate the IFR without any specific knowledge about the degree to which the data are biased by preferential testing. In this paper we take a partial identifiability approach, formulating clearly where deliberate prior assumptions can be made and presenting a Bayesian model which pools information from different samples. When the model is fit to European data obtained from seroprevalence studies and national official COVID-19 statistics, we estimate the overall COVID-19 IFR for Europe to be 0.53%, 95% C.I. = [0.38%, 0.70%].
更多查看译文
关键词
Selection bias,partial identification,evidence synthesis
AI 理解论文
溯源树
样例
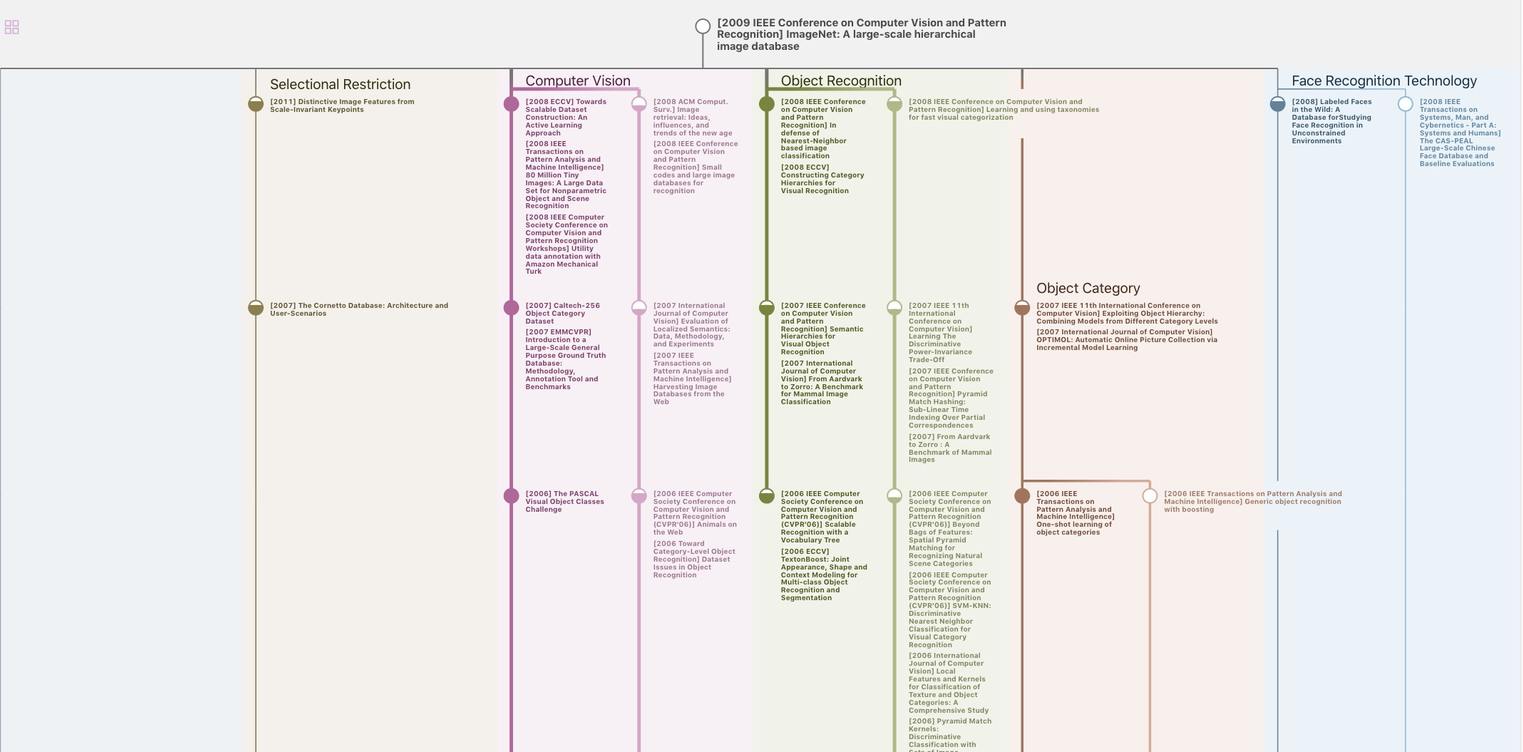
生成溯源树,研究论文发展脉络
Chat Paper
正在生成论文摘要