An Anomaly Detection Method for Multiple Time Series Based on Similarity Measurement and Louvain Algorithm
3RD INTERNATIONAL CONFERENCE ON INDUSTRY 4.0 AND SMART MANUFACTURING(2022)
摘要
Anomaly detection is an important task for data mining Detecting anomalies in the data collected from Municipal Solid Waste (MSW) incineration process is critical for their post processing, which helps to decrease emissions of typical flue gas pollutants and reduce costs. In this paper, we propose an unsupervised multiple time series anomaly detection model based on similarity measurement and Louvain algorithm, which consists of two stages. The first stage aims to find a subset of correlated time series data. The second stage is to detect anomalies in the subset, which can identify anomalous time windows and anomalous time subseries in the anomaly windows. The proposed method is applied on the data collected from an MSW incinerator in South China The results demonstrate the effectiveness of the proposed method. In addition, four similarity measure approaches are conducted and the results show that the model performs the best when using Pearson correlation coefficient, with an F2-score of 0.92. (C) 2022 The Authors. Published by Elsevier B.V.
更多查看译文
关键词
municipal solid waste incineration, anomaly detection, machine-learning, Louvain algorithm, similarity measurement
AI 理解论文
溯源树
样例
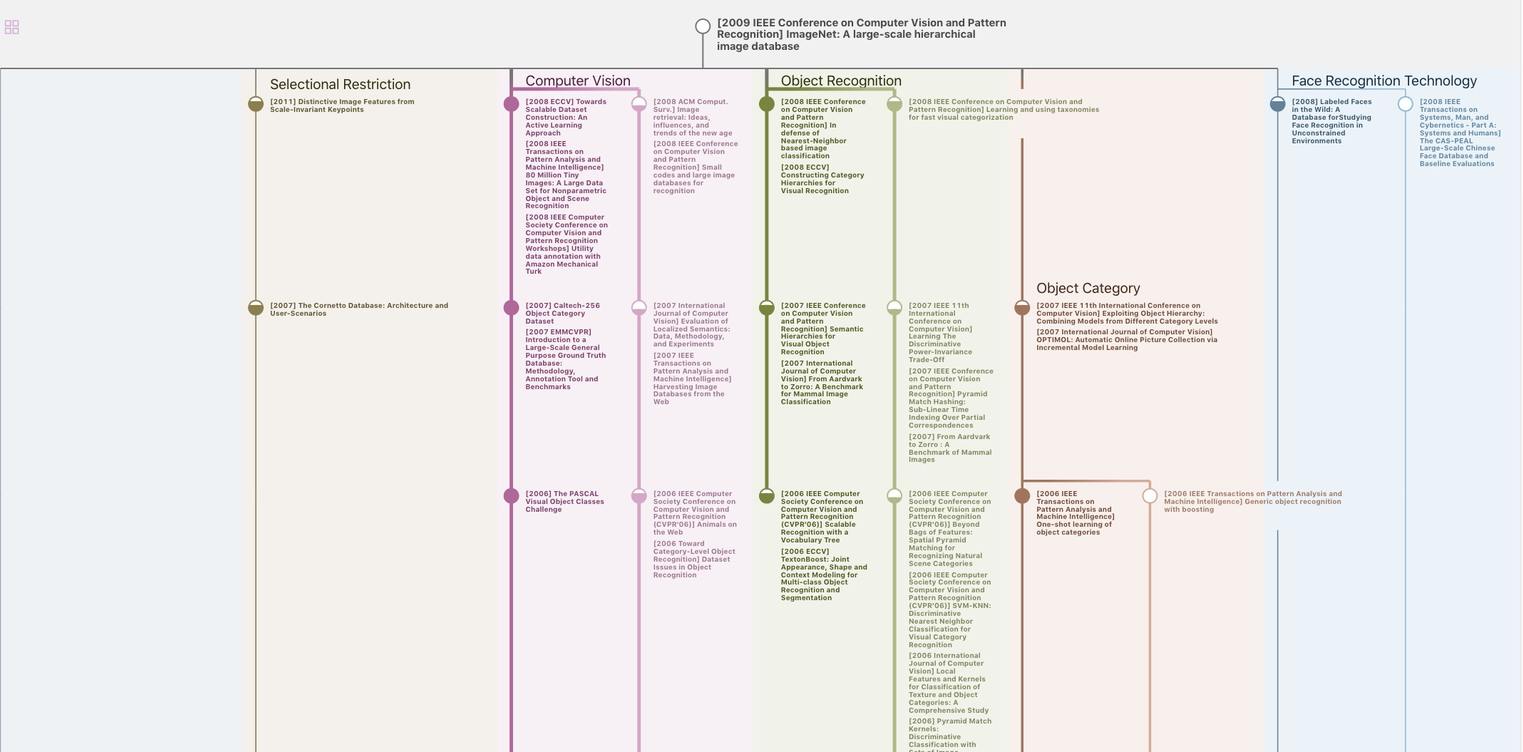
生成溯源树,研究论文发展脉络
Chat Paper
正在生成论文摘要