BOOTSTRAP INFERENCE FOR MULTIPLE CHANGE-POINTS IN TIME SERIES
Econometric Theory(2021)
Hang Seng Univ Hong Kong | Univ British Columbia | Chinese Univ Hong Kong
Abstract
In this paper, we propose two bootstrap procedures, namely parametric and block bootstrap, to approximate the finite sample distribution of change-point estimators for piecewise stationary time series. The bootstrap procedures are then used to develop a generalized likelihood ratio scan method (GLRSM) for multiple change-point inference in piecewise stationary time series, which estimates the number and locations of change-points and provides a confidence interval for each change-point. The computational complexity of using GLRSM for multiple change-point detection is as low as $O(n(\log n)^{3})$ for a series of length n. Extensive simulation studies are provided to demonstrate the effectiveness of the proposed methodology under different scenarios. Applications to financial time series are also illustrated.
MoreTranslated text
Key words
Covariance Estimation
求助PDF
上传PDF
View via Publisher
AI Read Science
AI Summary
AI Summary is the key point extracted automatically understanding the full text of the paper, including the background, methods, results, conclusions, icons and other key content, so that you can get the outline of the paper at a glance.
Example
Background
Key content
Introduction
Methods
Results
Related work
Fund
Key content
- Pretraining has recently greatly promoted the development of natural language processing (NLP)
- We show that M6 outperforms the baselines in multimodal downstream tasks, and the large M6 with 10 parameters can reach a better performance
- We propose a method called M6 that is able to process information of multiple modalities and perform both single-modal and cross-modal understanding and generation
- The model is scaled to large model with 10 billion parameters with sophisticated deployment, and the 10 -parameter M6-large is the largest pretrained model in Chinese
- Experimental results show that our proposed M6 outperforms the baseline in a number of downstream tasks concerning both single modality and multiple modalities We will continue the pretraining of extremely large models by increasing data to explore the limit of its performance
Upload PDF to Generate Summary
Must-Reading Tree
Example
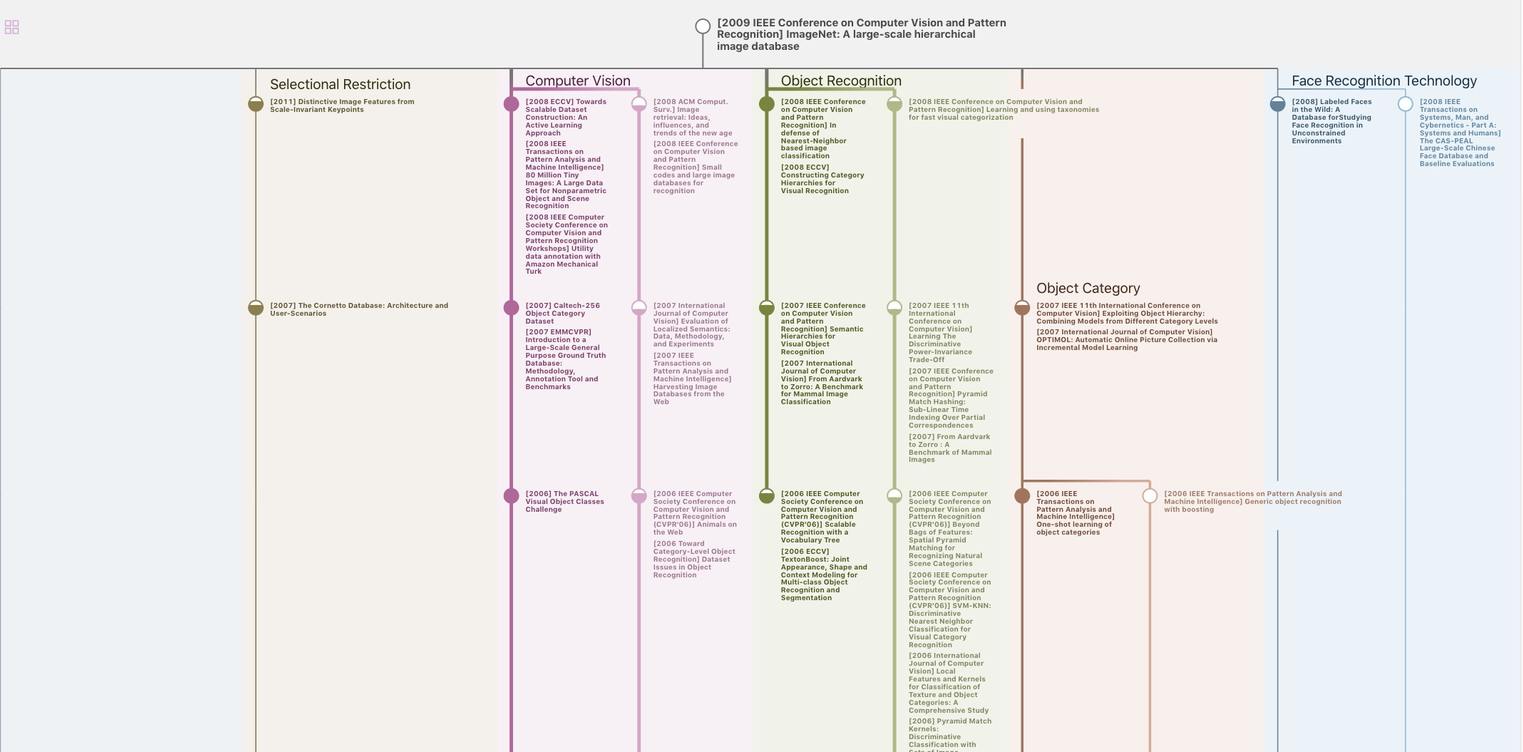
Generate MRT to find the research sequence of this paper
Related Papers
Bootstrap Confidence Intervals for Multiple Change Points Based on Moving Sum Procedures
Computational Statistics & Data Analysis 2022
被引用9
Linking Frequentist and Bayesian Change-Point Methods
JOURNAL OF BUSINESS & ECONOMIC STATISTICS 2024
被引用1
Data Disclaimer
The page data are from open Internet sources, cooperative publishers and automatic analysis results through AI technology. We do not make any commitments and guarantees for the validity, accuracy, correctness, reliability, completeness and timeliness of the page data. If you have any questions, please contact us by email: report@aminer.cn
Chat Paper