SoMoGym: A Toolkit for Developing and Evaluating Controllers and Reinforcement Learning Algorithms for Soft Robots
IEEE ROBOTICS AND AUTOMATION LETTERS(2022)
摘要
Soft robotsoffer a host of benefits over traditional rigid robots, including inherent compliance that lets them passively adapt to variable environments and operate safely around humans and fragile objects. However, that same compliance makes it hard to use model-based methods in planning tasks requiring high precision or complex actuation sequences. Reinforcement learning (RL) can potentially find effective control policies, but training RL using physical soft robots is often infeasible, and training using simulations has had a high barrier to adoption. To accelerate research in control and RL for soft robotic systems, we introduce SoMoGym (Soft Motion Gym), a software toolkit that facilitates training and evaluating controllers for continuum robots. SoMoGym provides a set of benchmark tasks in which soft robots interact with various objects and environments. It allows evaluation of performance on these tasks for controllers of interest, and enables the use of RL to generate new controllers. Custom environments and robots can likewise be added easily. We provide and evaluate baseline RL policies for each of the benchmark tasks. These results show that SoMoGym enables the use of RL for continuum robots, a class of robots not covered by existing benchmarks, giving them the capability to autonomously solve tasks that were previously unattainable.
更多查看译文
关键词
Task analysis, Robots, Benchmark testing, Soft robotics, Manipulators, Actuators, Training, Soft robot applications, modeling, control, and learning for soft robots, reinforcement learning
AI 理解论文
溯源树
样例
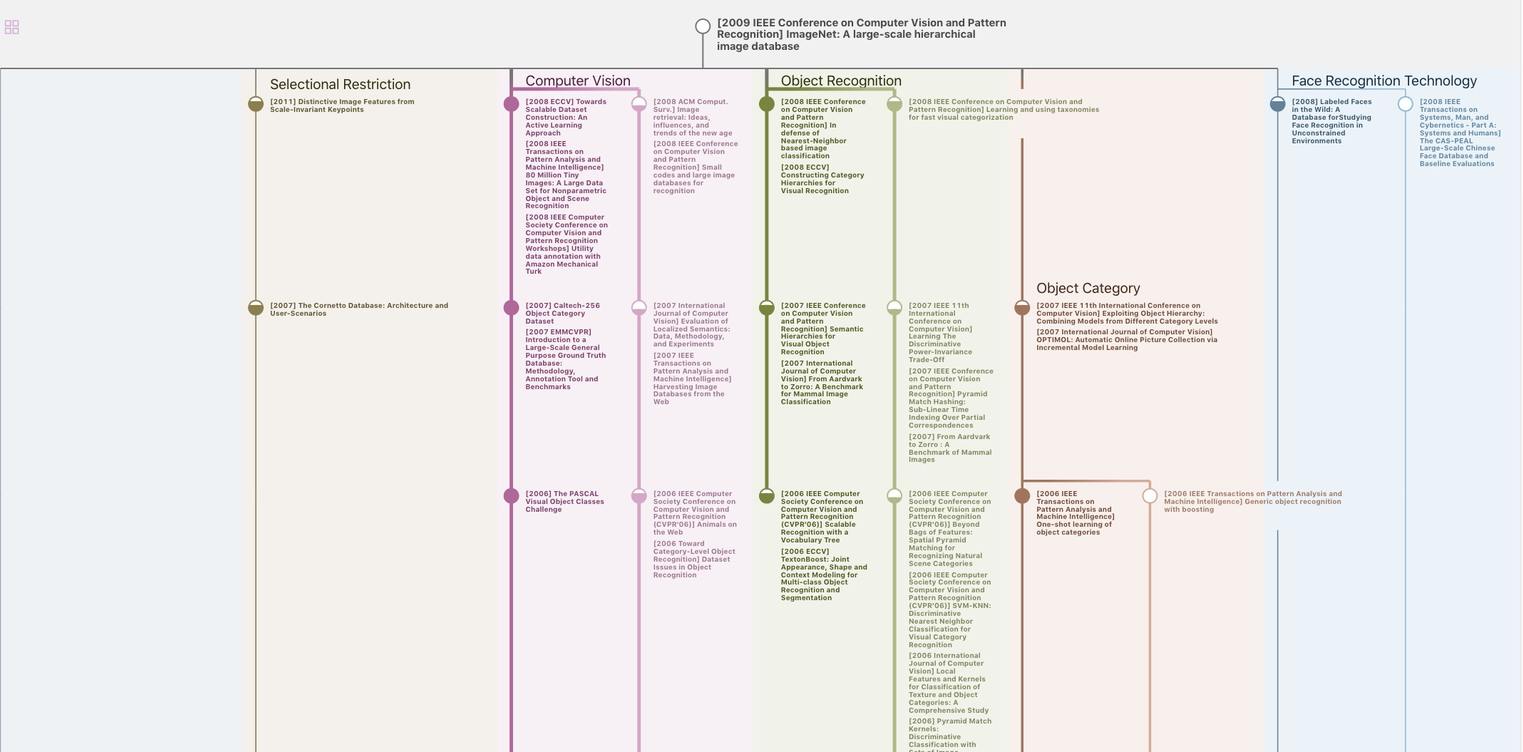
生成溯源树,研究论文发展脉络
Chat Paper
正在生成论文摘要