Using Graph Embedding Techniques in Process-Oriented Case-Based Reasoning
ALGORITHMS(2022)
摘要
Similarity-based retrieval of semantic graphs is a core task of Process-Oriented Case-Based Reasoning (POCBR) with applications in real-world scenarios, e.g., in smart manufacturing. The involved similarity computation is usually complex and time-consuming, as it requires some kind of inexact graph matching. To tackle these problems, we present an approach to modeling similarity measures based on embedding semantic graphs via Graph Neural Networks (GNNs). Therefore, we first examine how arbitrary semantic graphs, including node and edge types and their knowledge-rich semantic annotations, can be encoded in a numeric format that is usable by GNNs. Given this, the architecture of two generic graph embedding models from the literature is adapted to enable their usage as a similarity measure for similarity-based retrieval. Thereby, one of the two models is more optimized towards fast similarity prediction, while the other model is optimized towards knowledge-intensive, more expressive predictions. The evaluation examines the quality and performance of these models in preselecting retrieval candidates and in approximating the ground-truth similarities of a graph-matching-based similarity measure for two semantic graph domains. The results show the great potential of the approach for use in a retrieval scenario, either as a preselection model or as an approximation of a graph similarity measure.
更多查看译文
关键词
Case-Based Reasoning, Process-Oriented Case-Based Reasoning, graph embedding, Siamese Graph Neural Networks, similarity-based retrieval, neural networks, graph encoding, MAC, FAC retrieval
AI 理解论文
溯源树
样例
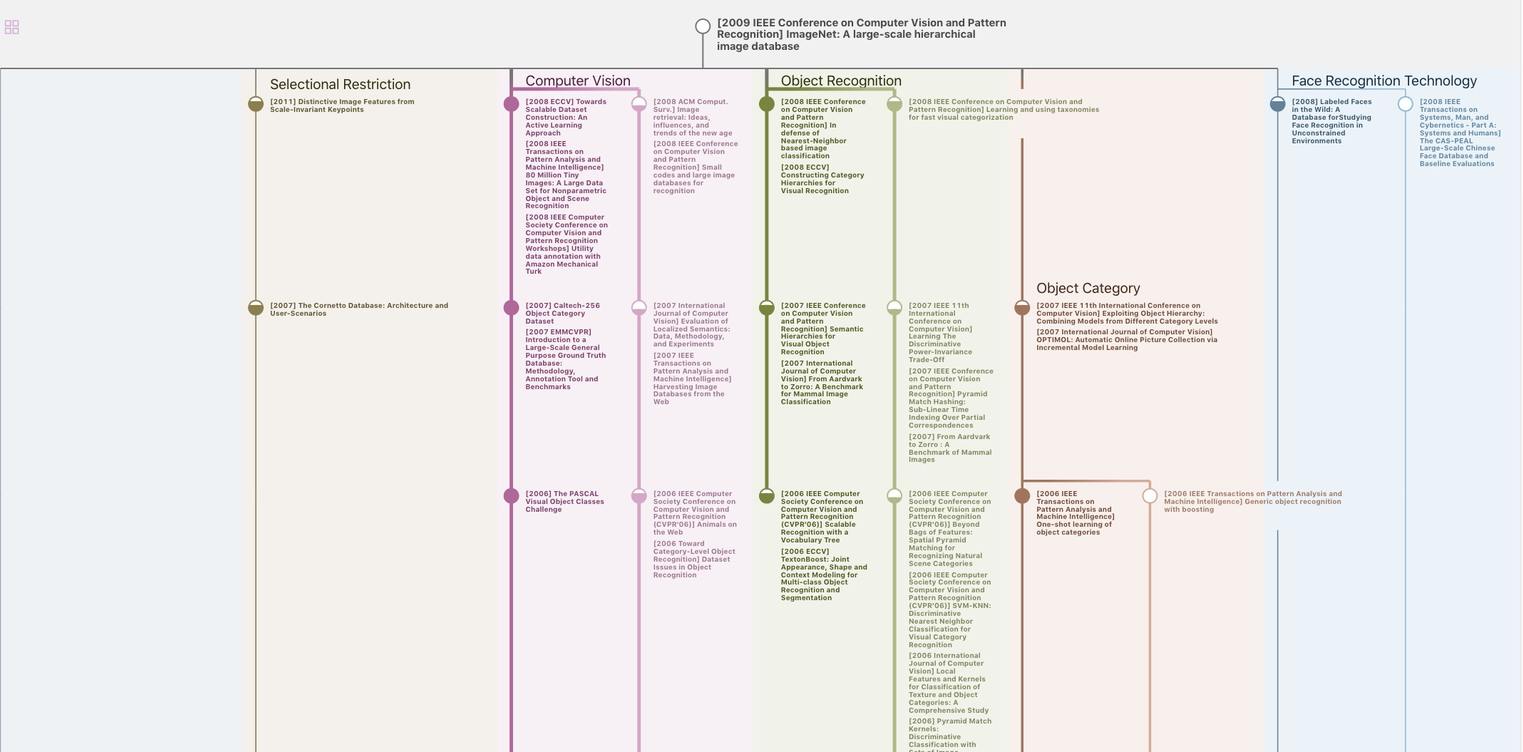
生成溯源树,研究论文发展脉络
Chat Paper
正在生成论文摘要