A penalized likelihood approach for dealing with separation in count data regression model
COMMUNICATIONS IN STATISTICS-SIMULATION AND COMPUTATION(2024)
摘要
Separation or monotone likelihood can be observed in fitting process of both Poisson or generalized Poisson (GP) regression, particularly in case of small and/or sparse count data, using maximum likelihood estimation (MLE) when one or more regression coefficients diverge to infinity. The study investigates the consequence of separation in the MLE based standard Poisson or GP model and addressed the problems by introducing a penalized likelihood approach. The penalized likelihood function is derived by adding a penalty term to the standard maximum likelihood function, which was originally proposed by Firth (1993) for reducing first order bias in MLE. The corresponding penalized likelihood score equation has shown to achieve convergence and provide finite estimate of the regression coefficient, which was not possible for the maximum likelihood method score equation. The simulation study, with different forms of separation, showed that penalized Poisson model or penalized GP outperform the standard Poisson and even the Zero-inflated Poisson (ZIP) in the presence of complete or quasi-complete separation by achieving convergence and providing finite estimate of the regression coefficients. Even in the presence of near-to-quasi-complete separation, which is very common in practice, the penalized method showed better results than the standard Poisson, GP and ZIP in all simulation scenarios. The method was illustrated using antenatal care data extracted from database of the Bangladesh demographic health survey 2018.
更多查看译文
关键词
Bias reduction,Jeffreys prior,Monotone likelihood,Score function,Zero-inflated Poisson model
AI 理解论文
溯源树
样例
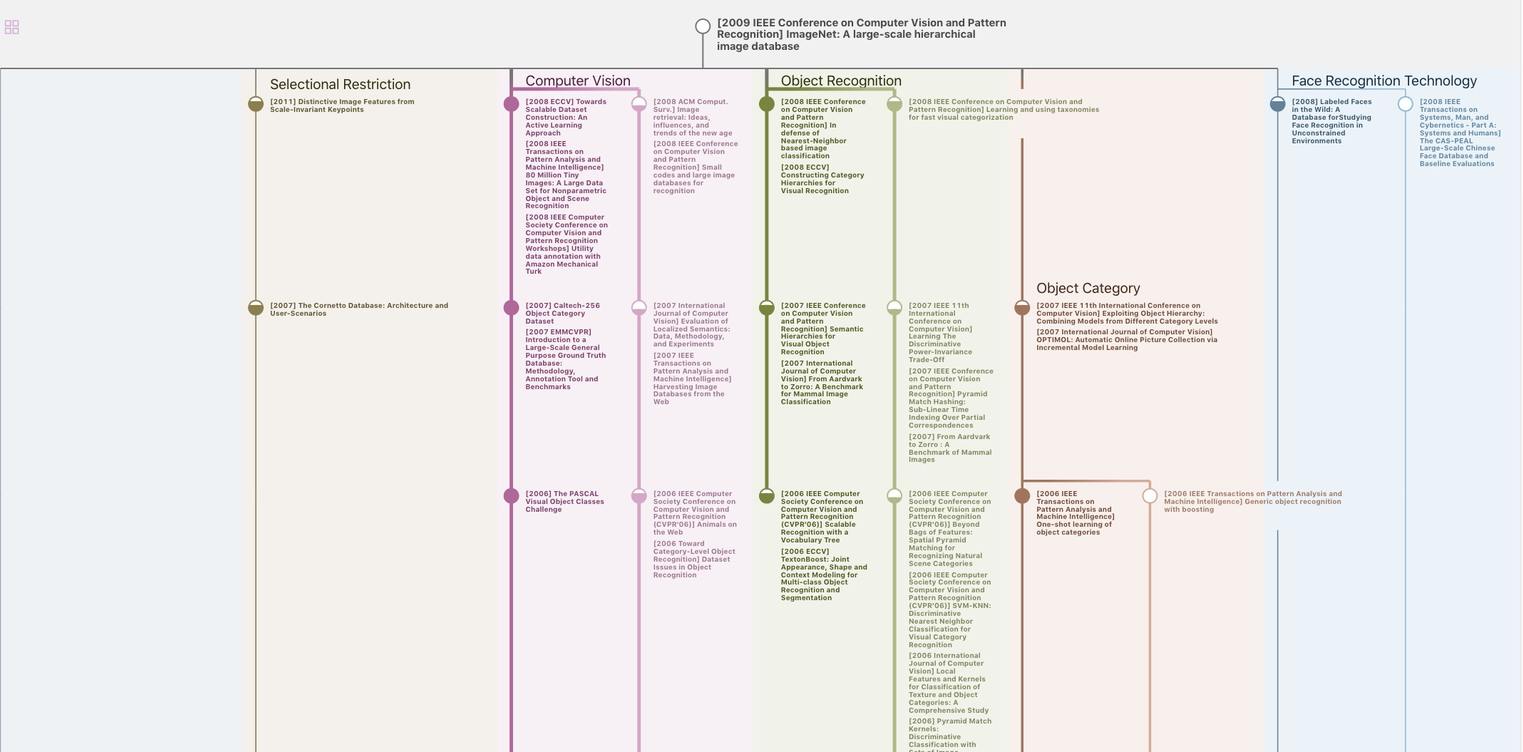
生成溯源树,研究论文发展脉络
Chat Paper
正在生成论文摘要