Partial Label Dimensional Reduction via Semantic Difference Information and Manifold Regularization
INTERNATIONAL JOURNAL ON ARTIFICIAL INTELLIGENCE TOOLS(2022)
摘要
Partial label learning is a rising weakly supervised learning framework that deals with the problem that each training instance is associated with a set of candidate labels, where only one is correct. The dimensionality reduction mechanism can effectively improve the learning system's generalization performance. However, due to the ambiguity of the ground-truth label, it is hard to use traditional dimensionality reduction methods directly on partially labeled data sets. Existing research attempts to exploit the linear discriminant analysis (LDA) strategy to reduce the dimensionality of partial label training examples. Unfortunately, this method still suffered from false-positive labels. This paper proposed an LDA-based dimensionality reduction approach named SDIMR for partially labeled datasets. On the one hand, introduce the manifold regularization term with semantic difference information to perform dimensionality reduction procedures and simultaneously maintain the local manifold structure. On the other hand, the iterative process alternates between dimensionality reduction and disambiguation. Briefly, our approach consists of three stages: Firstly, utilize the graph structure with semantic difference information to describe the topological information in the feature space. Secondly, we propose a new objective function containing manifold regularization terms to reduce the dimensionality of original training data to generate a new training data set. Finally, the k-nearest neighbor method is employed to disambiguate the dimensionality-reduced data. Extensive experiments on artificial and real-world data sets show that our method can be comparable to state-of-the-art partial label learning algorithms.
更多查看译文
关键词
Partial label learning, dimensional reduction, semantic difference information, manifold regularization
AI 理解论文
溯源树
样例
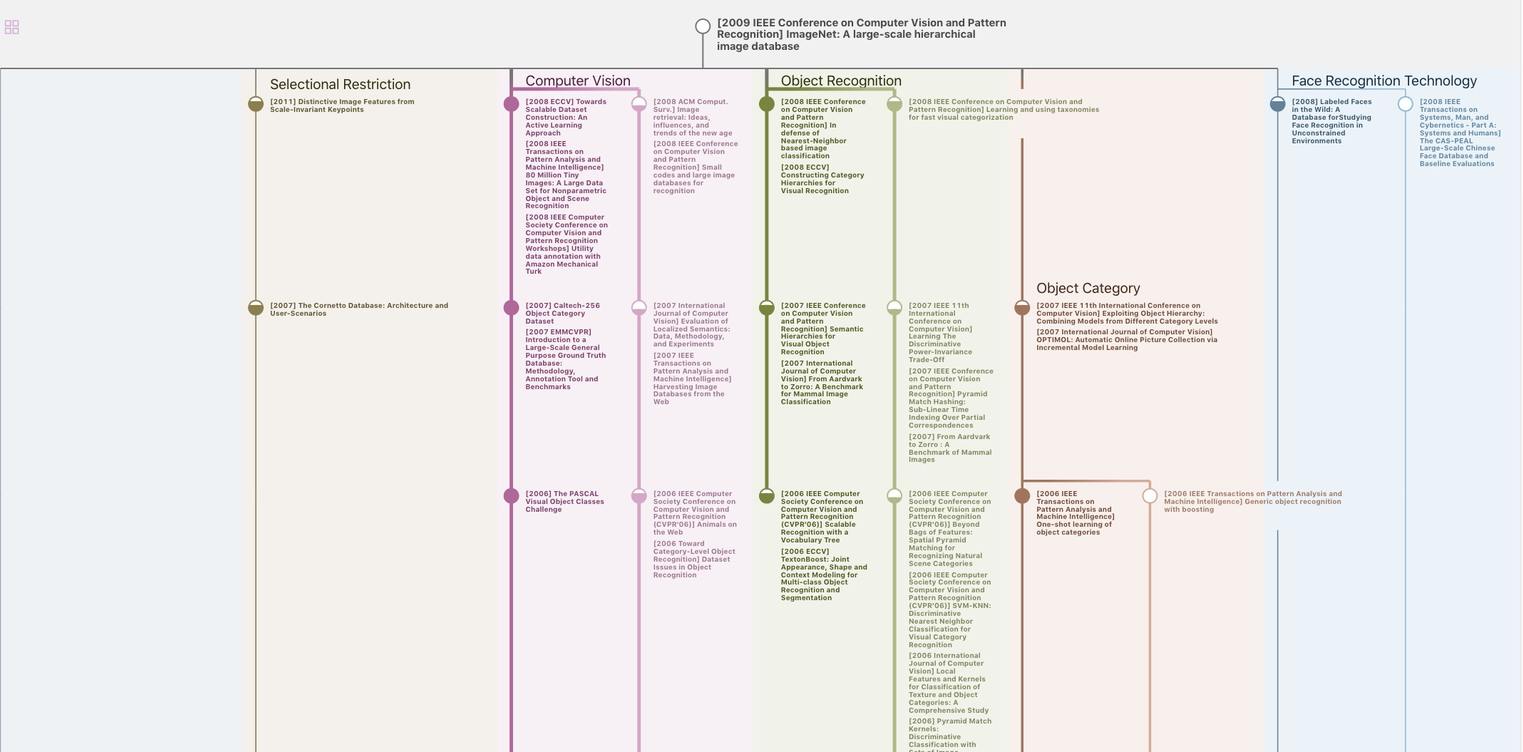
生成溯源树,研究论文发展脉络
Chat Paper
正在生成论文摘要