Enhanced Local Gradient Smoothing: Approaches to Attacked-region Identification and Defense
ICAART: PROCEEDINGS OF THE 14TH INTERNATIONAL CONFERENCE ON AGENTS AND ARTIFICIAL INTELLIGENCE - VOL 2(2022)
摘要
Mainstream deep learning algorithms have been shown vulnerable to adversarial attacks - the deep models could be misled by adding small unnoticeable perturbations to the original input image. These attacks could pose security challenges in real-world applications. The paper focuses on how to defend against an adversarial patch attack that confines such noises within a small and localized patch area. We will discuss how an adversarial sample affects the classifier output from the perspective of a deep model by visualizing its saliency map. On the basis of our baseline method: Local Gradients Smoothing, we further design two methods called Saliency-map-based Local Gradients Smoothing and Weighted Local Gradients Smoothing, integrating saliency maps with local gradient maps to accurately locate a possible attacked region and perform smoothing accordingly. Experimental results show that our proposed method could reduce the probability of false smoothing and increase the overall accuracy significantly.
更多查看译文
关键词
Adversarial Attack, Adversarial Defense, Reactive Defense, Data Pre-processing, Deep Learning
AI 理解论文
溯源树
样例
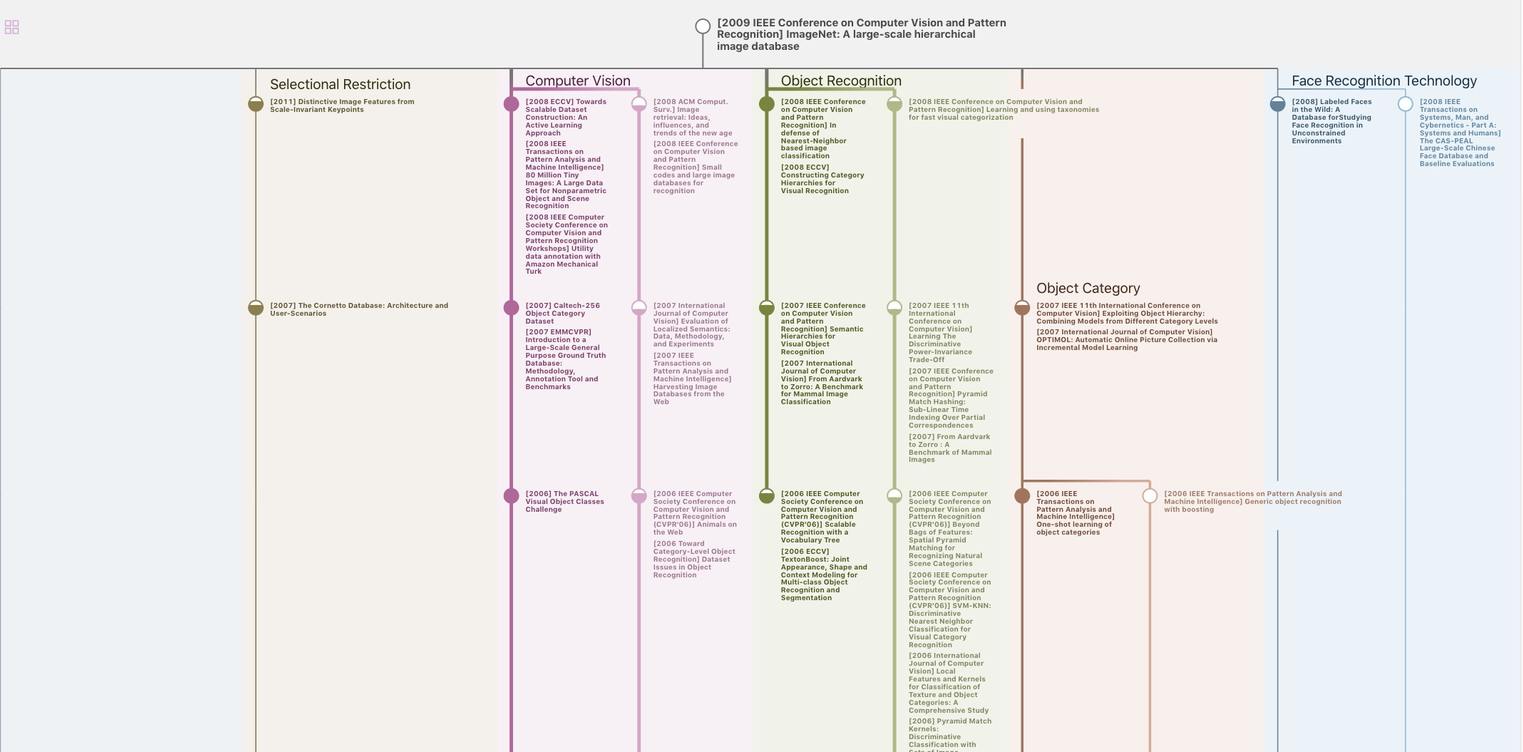
生成溯源树,研究论文发展脉络
Chat Paper
正在生成论文摘要