Generation of Data-Driven Expected Energy Models for Photovoltaic Systems
APPLIED SCIENCES-BASEL(2022)
摘要
Although unique expected energy models can be generated for a given photovoltaic (PV) site, a standardized model is also needed to facilitate performance comparisons across fleets. Current standardized expected energy models for PV work well with sparse data, but they have demonstrated significant over-estimations, which impacts accurate diagnoses of field operations and maintenance issues. This research addresses this issue by using machine learning to develop a data-driven expected energy model that can more accurately generate inferences for energy production of PV systems. Irradiance and system capacity information was used from 172 sites across the United States to train a series of models using Lasso linear regression. The trained models generally perform better than the commonly used expected energy model from international standard (IEC 61724-1), with the two highest performing models ranging in model complexity from a third-order polynomial with 10 parameters (R-adj(2) = 0.994) to a simpler, second-order polynomial with 4 parameters (R-adj(2)=0.993), the latter of which is subject to further evaluation. Subsequently, the trained models provide a more robust basis for identifying potential energy anomalies for operations and maintenance activities as well as informing planning-related financial assessments. We conclude with directions for future research, such as using splines to improve model continuity and better capture systems with low (& LE;1000 kW DC) capacity.
更多查看译文
关键词
photovoltaic systems, expected energy models, fleet-scale, lasso regression, performance modeling, machine learning
AI 理解论文
溯源树
样例
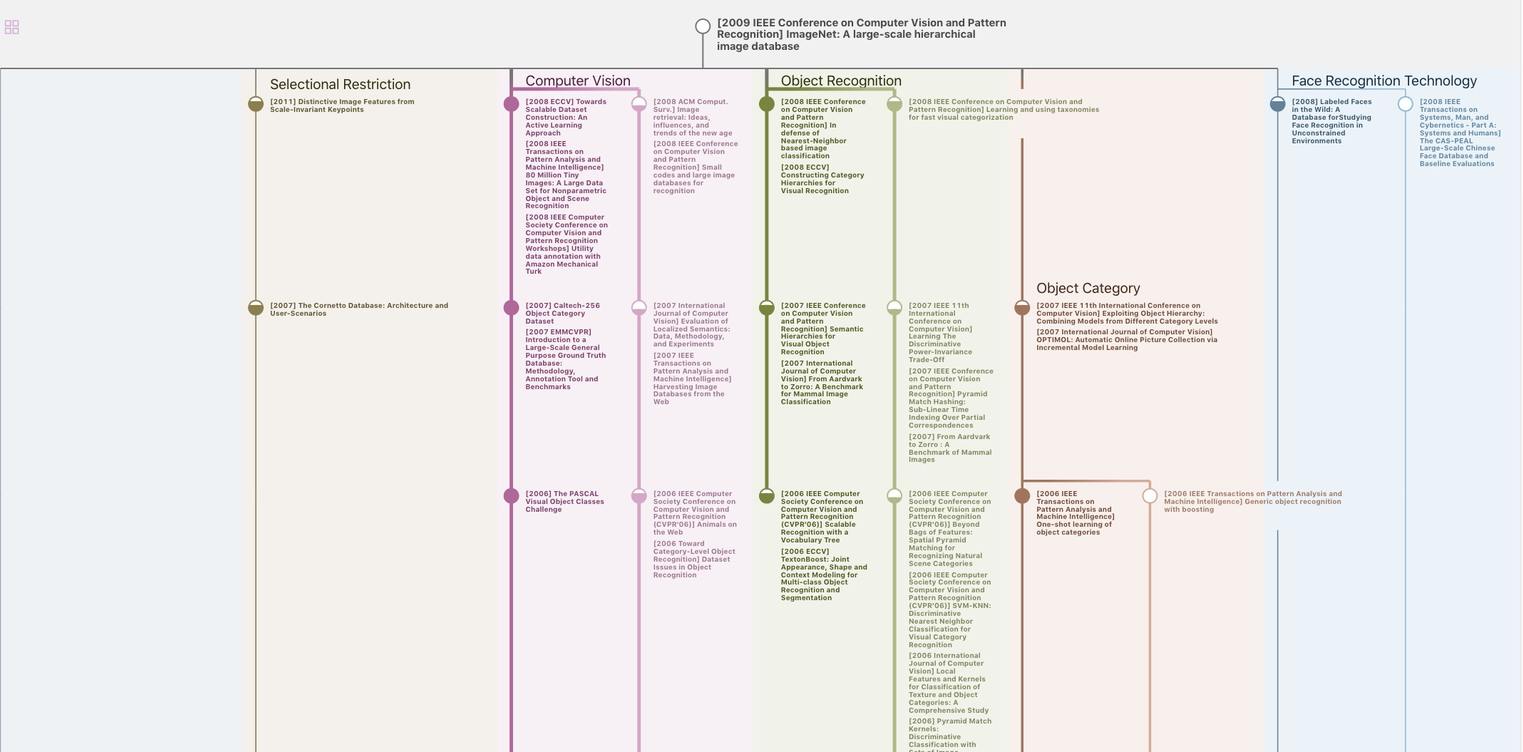
生成溯源树,研究论文发展脉络
Chat Paper
正在生成论文摘要