Inductive Generalized Zero-Shot Learning with Adversarial Relation Network
MACHINE LEARNING AND KNOWLEDGE DISCOVERY IN DATABASES, ECML PKDD 2020, PT II(2021)
摘要
We consider the inductive Generalized Zero Shot Learning (GZSL) problem where test information is assumed unavailable during training. In lack of training samples and attributes for unseen classes, most existing GZSL methods tend to classify target samples as seen classes. To alleviate such problem, we design an adversarial Relation Network that favors target samples towards unseen classes while enjoying robust recognition for seen classes. Specifically, through the adversarial framework, we can attain a robust recognizer where a small gradient adjustment to the instance will not affect too much the classification of seen classes but substantially increase the classification accuracy on unseen classes. We conduct a series of experiments extensively on four benchmarks i.e., AwA1, AwA2, aPY, and CUB. Experimental results show that our proposed method can attain encouraging performance, which is higher than the best of state-of-the-art models by 10.8%, 14.0%, 6.9%, and 1.9% on the four benchmark datasets, respectively in the inductive GZSL scenario. (The code is available on https://github.com/ygyvsys/AdvRN-with-SR)
更多查看译文
关键词
Zero-shot learning, Adversarial examples, Gradient penalty
AI 理解论文
溯源树
样例
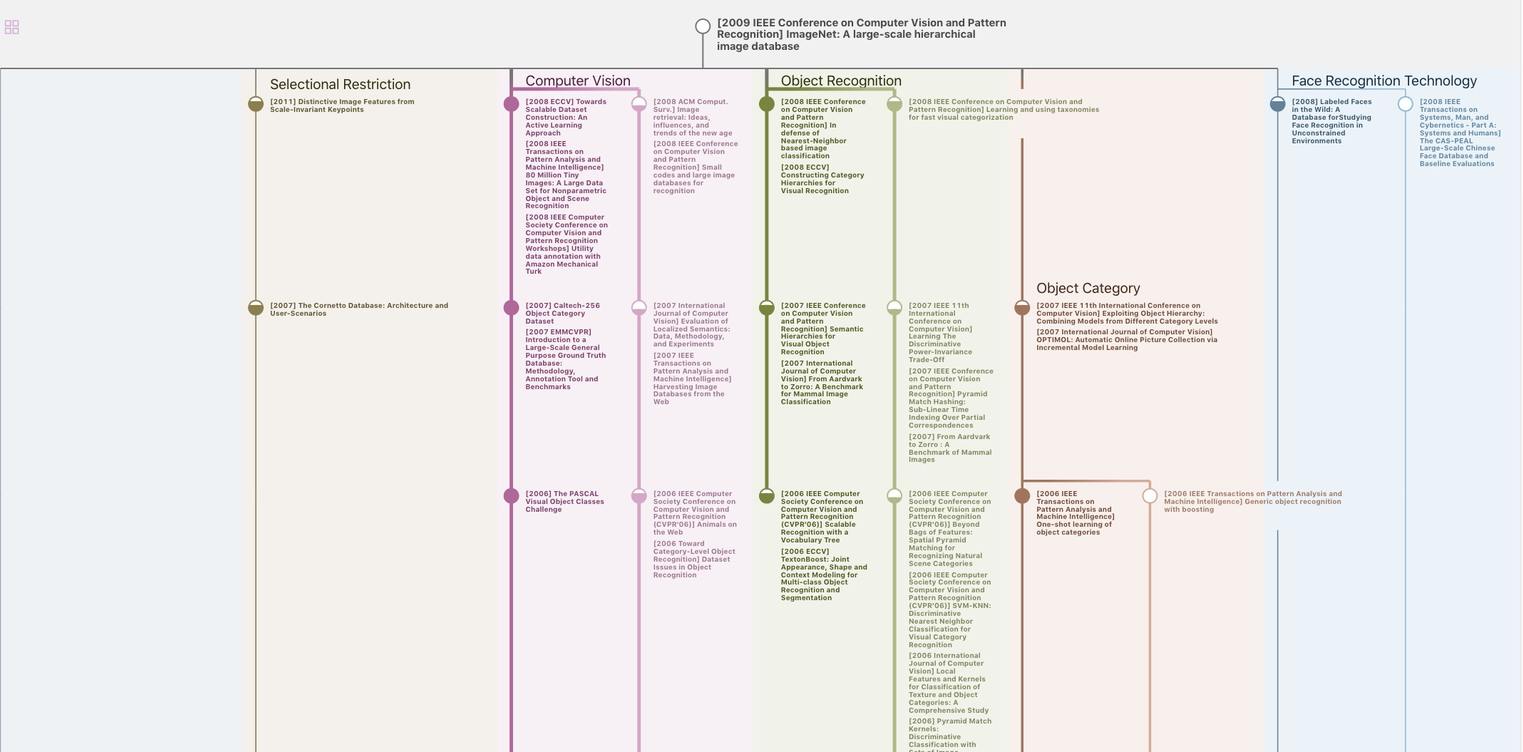
生成溯源树,研究论文发展脉络
Chat Paper
正在生成论文摘要