MFVT: an anomaly traffic detection method merging feature fusion network and vision transformer architecture
EURASIP Journal on Wireless Communications and Networking(2022)
摘要
Network intrusion detection, which takes the extraction and analysis of network traffic features as the main method, plays a vital role in network security protection. The current network traffic feature extraction and analysis for network intrusion detection mostly uses deep learning algorithms. Currently, deep learning requires a lot of training resources and has weak processing capabilities for imbalanced datasets. In this paper, a deep learning model (MFVT) based on feature fusion network and vision transformer architecture is proposed, which improves the processing ability of imbalanced datasets and reduces the sample data resources needed for training. Besides, to improve the traditional raw traffic features extraction methods, a new raw traffic features extraction method (CRP) is proposed, and the CPR uses PCA algorithm to reduce all the processed digital traffic features to the specified dimension. On the IDS 2017 dataset and the IDS 2012 dataset, the ablation experiments show that the performance of the proposed MFVT model is significantly better than other network intrusion detection models, and the detection accuracy can reach the state-of-the-art level. And, when MFVT model is combined with CRP algorithm, the detection accuracy is further improved to 99.99%.
更多查看译文
关键词
Network intrusion detection, Traffic features, Deep learning, Feature fusion network, Vision transformer, MFVT, CRP, Detection accuracy
AI 理解论文
溯源树
样例
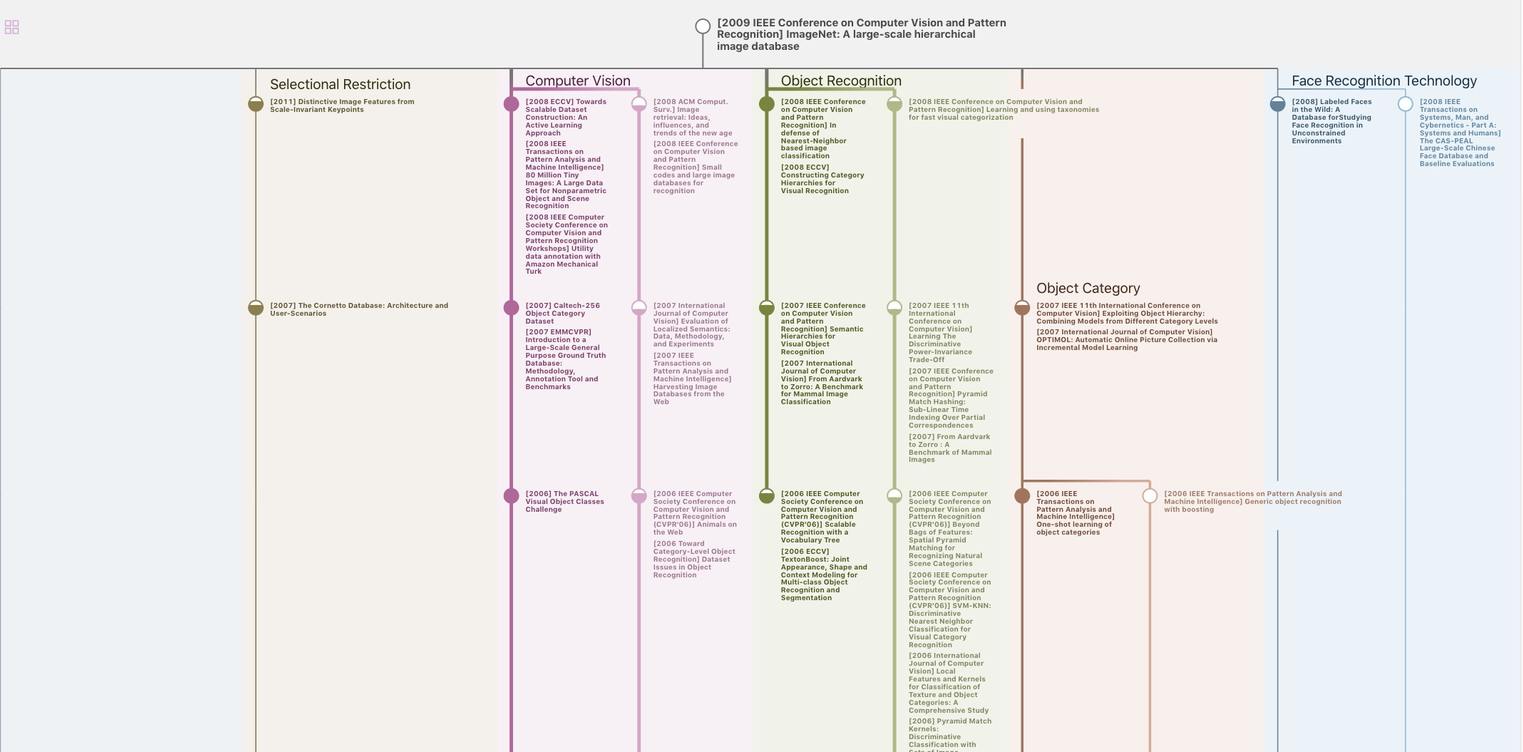
生成溯源树,研究论文发展脉络
Chat Paper
正在生成论文摘要