Method for predicting the remaining mileage of electric vehicles based on dimension expansion and model fusion
IET INTELLIGENT TRANSPORT SYSTEMS(2022)
摘要
Accurately predicting the remaining mileage of electric vehicles (EVs) can effectively alleviate user's mileage anxiety and develop refinement of energy management strategy. However, traditional prediction methods not only consume time and resources, but also accumulate errors and lack interpretability. In this paper, we proposed a model based on dimension expansion and model fusion strategy, which uses the extreme gradient boosting (XGBoost) algorithm to directly predict the remaining mileage of EVs. After pre-processing the real running data of EVs, we constructed the field of remaining driving range and analyzed the relationship between features and remaining driving range, and then directly predicted the remaining driving mileage. Compared with other machine learning methods, XGBoost model has the highest accuracy. Then dimensional extended data set was obtained based on prior knowledge and symbol conversion, which improved the model performance. Finally, the model fusion strategy was adopted to further improve the generalization ability and stability of the model. The experimental results show that the Bootstrap aggregating (Bagging) fusion model has the highest predictive performance on the test set and outperformed other methods. The maximum RAE is not more than 3.5%, RMSE is less than 3km and MAE is about 2 km.
更多查看译文
关键词
mileage,electric vehicles,dimension expansion
AI 理解论文
溯源树
样例
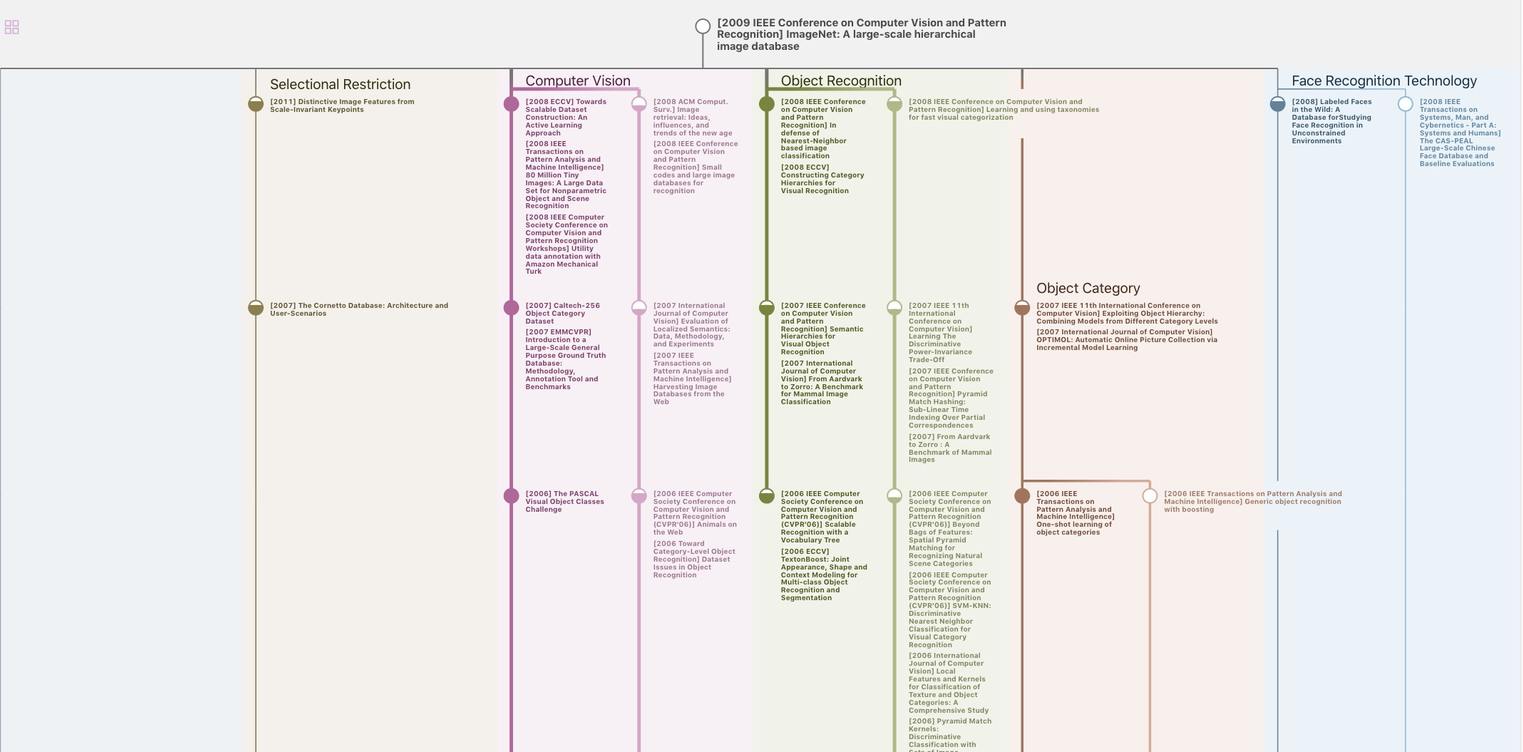
生成溯源树,研究论文发展脉络
Chat Paper
正在生成论文摘要