Arbitrarily-oriented tunnel lining defects detection from Ground Penetrating Radar images using deep Convolutional Neural networks
AUTOMATION IN CONSTRUCTION(2022)
摘要
Tunnel lining internal defect detection is essential for the safe operation of tunnels. This paper presents an automatic scheme based on rotational region deformable convolutional neural network ((RDCNN)-D-2) and Ground Penetrating Radar (GPR) images for the accurate detection of defects and rebars with arbitrary orientations. The (RDCNN)-D-2 comprises inter-related modules, specifically, deformable convolution, feature fusion, and rotated region detection modules. In this study, synthetic GPR images, including rebars and various structural defects with different permittivities, as well as real GPR images obtained by model experiments, were constructed for the (RDCNN)-D-2. Improved results were obtained while testing the (RDCNN)-D-2 on GPR images in comparative experiments. The mean average precision of the (RDCNN)-D-2 was enhanced by 8.21% compared to the (RCNN)-C-2 on synthetic GPR images. The (RDCNN)-D-2 showed satisfactory results in on-site experiments, which demonstrated the applicability of the (RDCNN)-D-2 to practical tunnels.
更多查看译文
关键词
Ground penetrating radar, Arbitrarily-oriented defect detection, Automation, Deep learning, Tunnel inspection
AI 理解论文
溯源树
样例
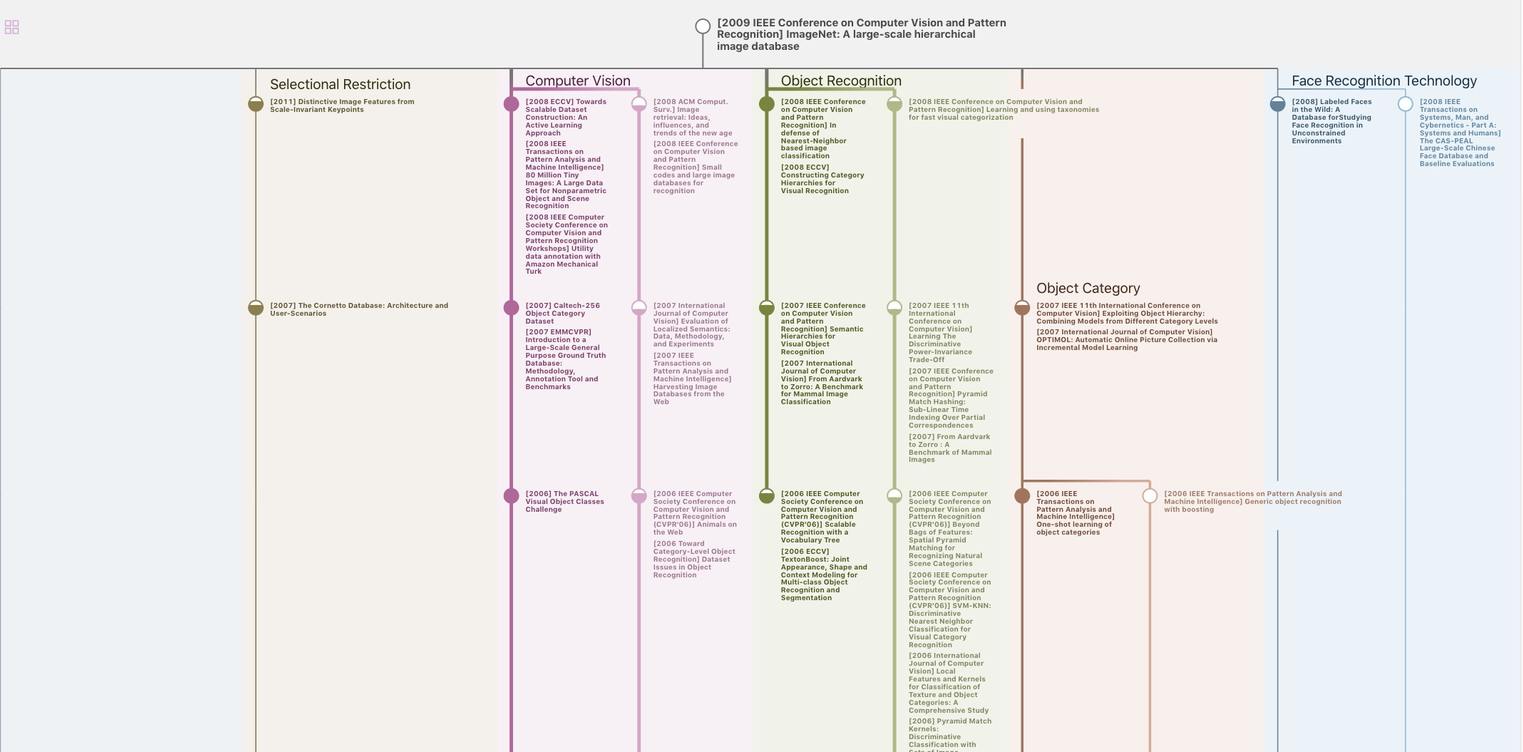
生成溯源树,研究论文发展脉络
Chat Paper
正在生成论文摘要