Gated Homogeneous Fusion Networks With Jointed Feature Extraction for Defect Prediction
IEEE Transactions on Reliability(2022)
摘要
Software defect prediction is aimed at helping developers to quickly locate defective components in the code repository and thus better allocate resources. However, most of the current traditional defect prediction methods mainly depend on the design of static metrics, but these methods ignore the semantic and structural information of the code. As a result, researchers have turned to building models by extracting semantic features from code through abstract syntax trees. In this article, we introduce
gated homogeneous fusion network
for defect prediction namely GHFNet, jointing high-level semantic feature extraction and weighted static feature extraction. Through the mechanism of homogeneous gating fusion, weights are adaptively assigned to the two types of features based on the correlation of these features to form fused features for defect prediction in the code. Experimental results show that the proposed approach is a significant improvement. Specifically, for the reference method we present GHFNet improved from 9.4 to 15.2 percentage points in effort-unaware scenarios (F-measure) and from 3.7 to 8 percentage points in effort-aware scenarios (Popt) for defect prediction.
更多查看译文
关键词
Attention mechanism,defect prediction (DP),neural network
AI 理解论文
溯源树
样例
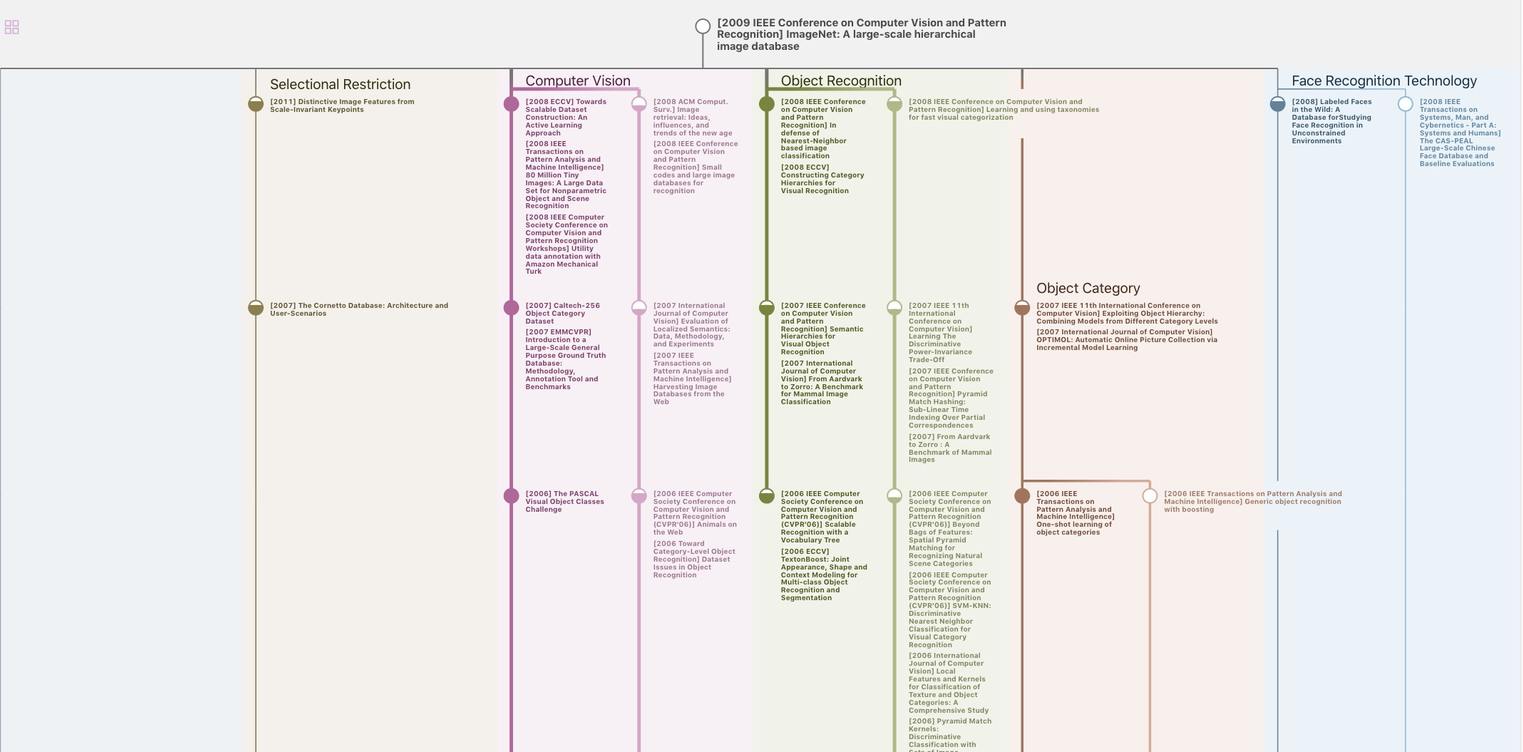
生成溯源树,研究论文发展脉络
Chat Paper
正在生成论文摘要