MFaster R-CNN for Maize Leaf Diseases Detection Based on Machine Vision
ARABIAN JOURNAL FOR SCIENCE AND ENGINEERING(2023)
摘要
In order to realize the intelligent diagnosis of maize diseases with complicated backgrounds and similar disease spot characteristics in the real field environment, MFaster R-CNN is proposed by improving the Faster R-CNN algorithm. Firstly, a batch normalization processing layer is added to the convolution layer to speed up the convergence speed of the network and improve the generalization ability of the model; secondly, a central cost function is proposed to construct a mixed loss function to improve the detection accuracy of similar lesions; then, four kinds of pre-trained convolution structures are selected as the basic feature extraction network of Faster R-CNN for training, and the random gradient descent algorithm is used to optimize the training model to test the optimal feature extraction network; finally, the trained model is used to select test sets under different weather conditions for comparison, and MFaster R-CNN is compared with Faster R-CNN and SSD. The experimental results show that in MFaster R-CNN disease detection framework, VGG16 convolution layer structure as feature extraction network has better performance, the average recall rate is 0.9719, F1 is 0.9718, the overall average accuracy rate can reach 97.23%; compared with Faster R-CNN, MFaster R-CNN has an average accuracy of 0.0886 higher and a single image detection time of 0.139 s less; compared with the SSD, the average accuracy is 0.0425 higher, and the single image detection time is reduced by 0.018 s. Our method also provides a basis for timely and accurate prevention and control of maize diseases in the field.
更多查看译文
关键词
Maize disease,Complicated background,Data augmentation,Regional convolution neural network,Batch normalization,Mixed loss function
AI 理解论文
溯源树
样例
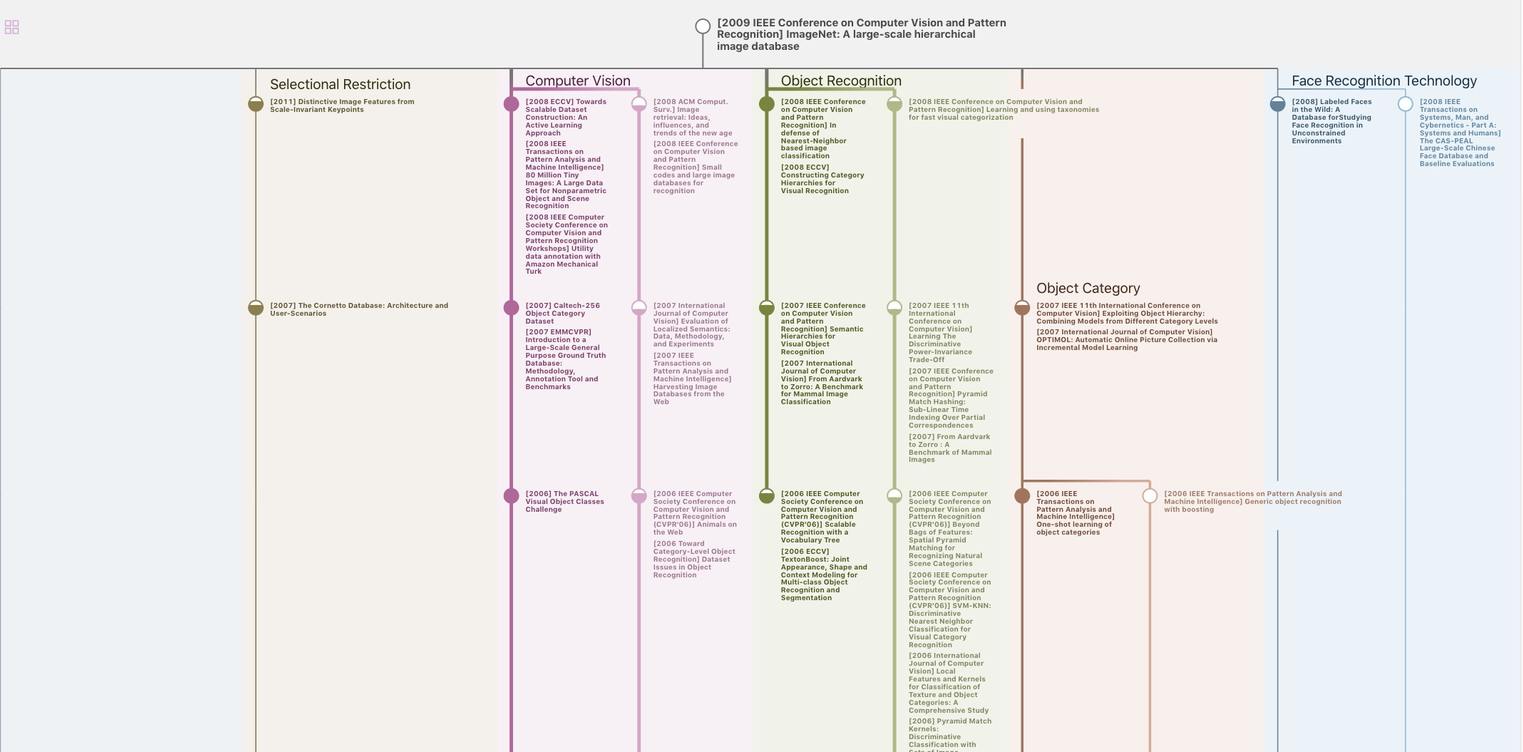
生成溯源树,研究论文发展脉络
Chat Paper
正在生成论文摘要