Towards accelerate d gree dy sampling and reconstruction of bandlimited graph signals
SIGNAL PROCESSING(2022)
摘要
We study the problem of sampling and reconstructing spectrally sparse graph signals where the objec-tive is to select a subset of nodes of prespecified cardinality that ensures interpolation of the original signal with the lowest possible reconstruction error. This task is of critical importance in Graph signal processing (GSP) and while existing methods generally provide satisfactory performance, they typically entail a prohibitive computational cost when it comes to the study of large-scale problems. Thus, there is a need for accelerated and efficient methods tailored for high-dimensional and large-scale sampling and reconstruction tasks. To this end, we first consider a non-Bayesian scenario and propose an efficient iterative node sampling procedure that in the noiseless case enables exact recovery of the original signal from the set of selected nodes. In the case of noisy measurements, a bound on the reconstruction error of the proposed algorithm is established. Then, we consider the Bayesian scenario where we formulate the sampling task as the problem of maximizing a monotone weak submodular function, and propose a randomized-greedy algorithm to find a sub-optimal subset of informative nodes. We derive worst-case performance guarantees on the mean-square error achieved by the randomized-greedy algorithm for gen-eral non-stationary graph signals.(c) 2022 Elsevier B.V. All rights reserved.
更多查看译文
关键词
Graph signal processing,Sampling,Reconstruction,Weak submodularity,Iterative algorithms
AI 理解论文
溯源树
样例
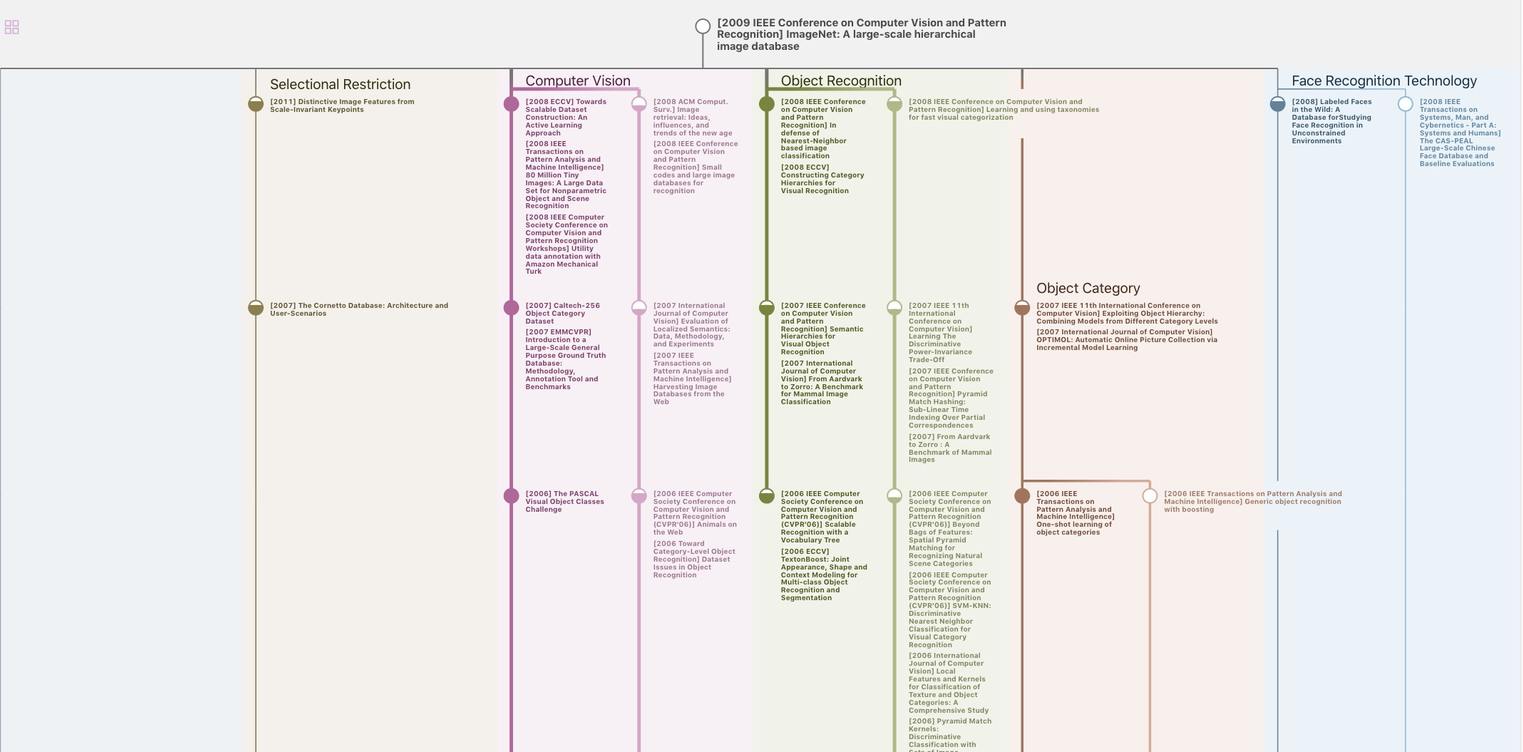
生成溯源树,研究论文发展脉络
Chat Paper
正在生成论文摘要