An Advanced Satisfaction-Based Home Energy Management System Using Deep Reinforcement Learning
IEEE ACCESS(2022)
摘要
Home energy management (HEM) systems optimize electricity demand of appliances according to the price-based demand response (DR) programs. Undoubtedly, customer satisfaction is of such importance that if not taken into consideration, it prevents customers from participating in the DR. HEM systems suffer from high nonlinearity due to the variety of smart appliances and different criteria for customer satisfaction. In this paper, an advanced satisfaction-based HEM system using deep reinforcement learning is proposed to hourly schedule the controllable and time-shiftable appliances, including electric vehicle, air conditioner, and lighting system as controllable loads and washing machine, and dishwasher as time-shiftable loads. The proposed framework deploys a Deep Q-Network (DQN) method. Regarding customer dissatisfaction, this paper takes into consideration nonlinear precise functions. The Kano model for EV departure SoC, charging duration and lighting system satisfaction, desired temperature span for air conditioner, and the desirable operation period, waiting time, and consecutive mode of dishwasher and washing machine are taken into account. The proposed HEM system is applied to a smart home, and the results are compared with those of the Q-Learning algorithm. Numerical results prove the effectiveness of the proposed HEM system in reducing electricity cost and customer dissatisfaction, as well as the superiority of DQN over Q-Learning as well.
更多查看译文
关键词
Home appliances, Q-learning, Load modeling, Atmospheric modeling, Energy consumption, Smart homes, Costs, Deep reinforcement learning, demand response, home energy management, customer dissatisfaction
AI 理解论文
溯源树
样例
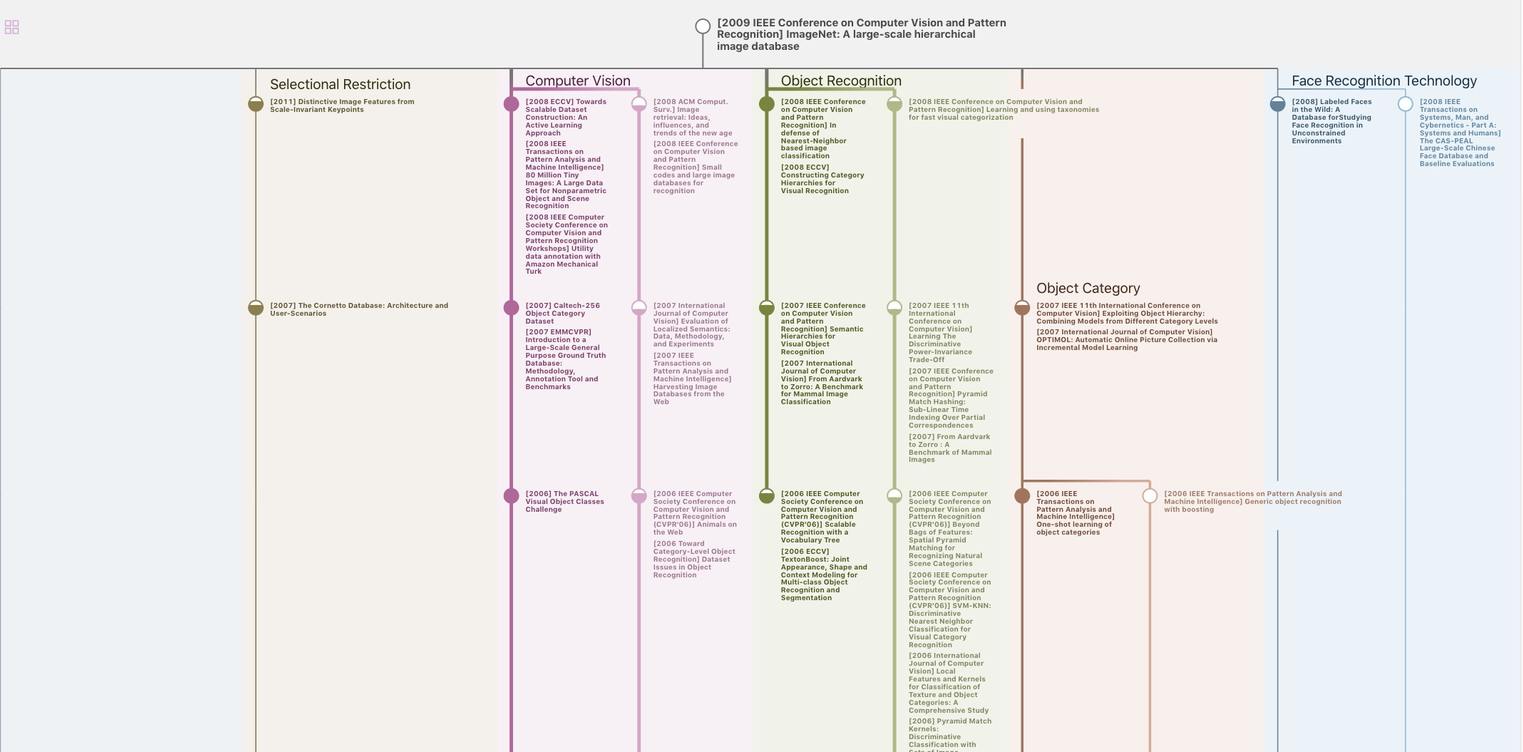
生成溯源树,研究论文发展脉络
Chat Paper
正在生成论文摘要