Kernel Product Neural Networks
IEEE ACCESS(2021)
摘要
Attention is an important field to explore the importance of each convolutional kernel channel/weight. The existing attention methods mostly use the Squeeze-and-Excitation (SE) technology to extract the global nonlinear feature vectors as the weights of corresponding feature maps. However, the pooling operators and fully-connected layers used in SE technology extract global features at the cost of much valuable information loss and the parameter amount increase. Actually, the feature map containing full information is a ready-made and better attention for other feature maps in the same layer. Simultaneously the products of feature maps will bring powerful non-linearity. Seeing this, Kernel Product (KP) technology is proposed to simply get useful nonlinear attention. To verify the effectiveness of KP, the proposed KP module is employed on Selective Kernel Networks (SKNets) to take the place of the original SE technology. The variety of SKNets is called Kernel Product Networks (KPNets) in this paper. In addition, identity mapping is used to solve the non-convergence problem in very deep neural networks. The KPNets are evaluated on ImageNet-1k, CIFAR-10, and CIFAR-100. The experiment results show that KPNets outperform many state-of-the-art methods and get a similar but more efficient performance than its SKNets with counterpart.
更多查看译文
关键词
Feature extraction,Kernel,Convolution,Fuses,Data mining,Visualization,Linearity,Attention,non-linearity,kernel product
AI 理解论文
溯源树
样例
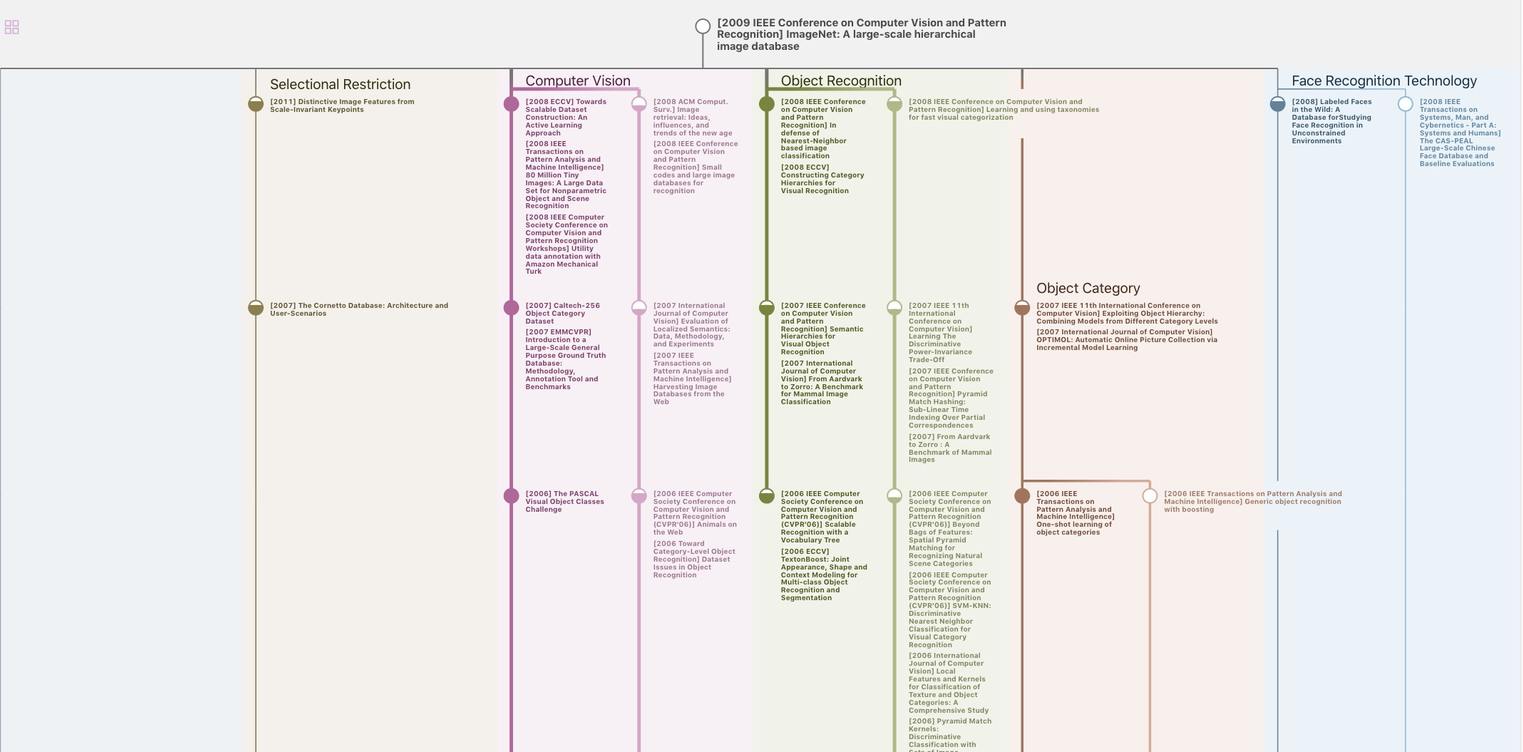
生成溯源树,研究论文发展脉络
Chat Paper
正在生成论文摘要