Intrinsically Motivated Lifelong Exploration in Reinforcement Learning
ADVANCES IN ARTIFICIAL INTELLIGENCE(2021)
摘要
Long-term horizon exploration remains a challenging problem in deep reinforcement learning, especially when an environment contains sparse or poorly-defined extrinsic rewards. To tackle this challenge, we propose a reinforcement learning agent to solve hard exploration tasks by leveraging a lifelong exploration bonus. Our method decomposes this bonus into a short-term and a long-term intrinsic reward. The former deals with local exploration - exploring the consequences of short-term decisions, while the latter explicitly encourages deep exploration strategies by remaining large throughout the training process. As formulation of intrinsic novelty, we propose to measure the reconstruction error of an observation given its context to capture flexible exploration behaviors characterized by different time horizons. We demonstrate the effectiveness of our approach in visually rich environments in Minigrid, DMLab, and Atari games. Experimental results validate that our method outperforms baselines in most tasks in terms of scores and exploration efficiency.
更多查看译文
关键词
Reinforcement learning, Exploration, Curiosity in reinforcement learning, Lifelong reinforcement learning
AI 理解论文
溯源树
样例
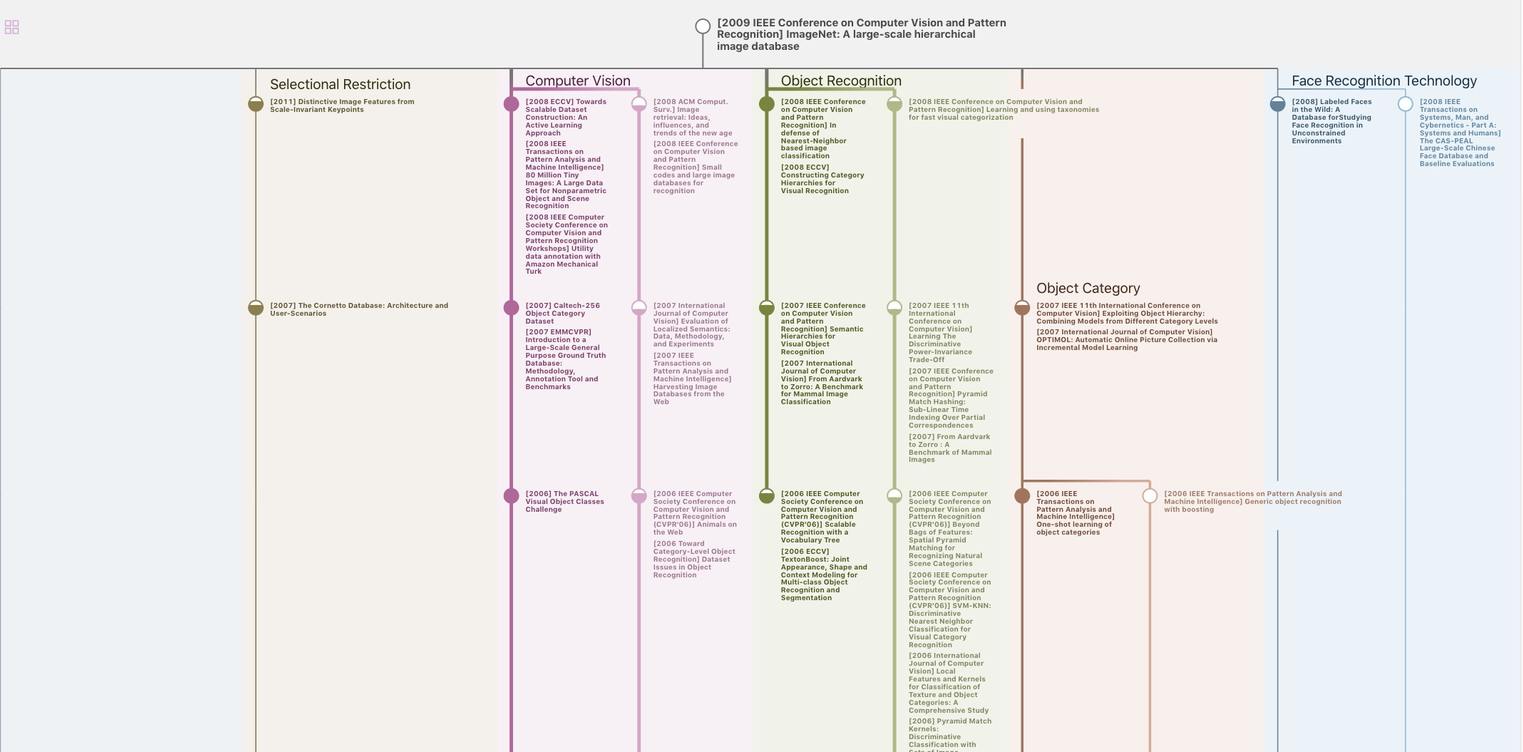
生成溯源树,研究论文发展脉络
Chat Paper
正在生成论文摘要