Federated Learning of Oligonucleotide Drug Molecule Thermodynamics with Differentially Private ADMM-Based SVM
MACHINE LEARNING AND PRINCIPLES AND PRACTICE OF KNOWLEDGE DISCOVERY IN DATABASES, PT II(2021)
摘要
A crucial step to assure drug safety is predicting off-target binding. For oligonucleotide drugs this requires learning the relevant thermodynamics from often large-scale data distributed across different organisations. This process will respect data privacy if distributed and private learning under limited and private communication between local nodes is used. We propose an ADMM-based SVM with differential privacy for this purpose. We empirically show that this approach achieves accuracy comparable to the non-private one, i.e. similar to 86%, while yielding tight empirical privacy guarantees even after convergence.
更多查看译文
关键词
Differential privacy, Distributed learning, Federated learning, Oligonucleotide drug molecules, ADMM, SVM
AI 理解论文
溯源树
样例
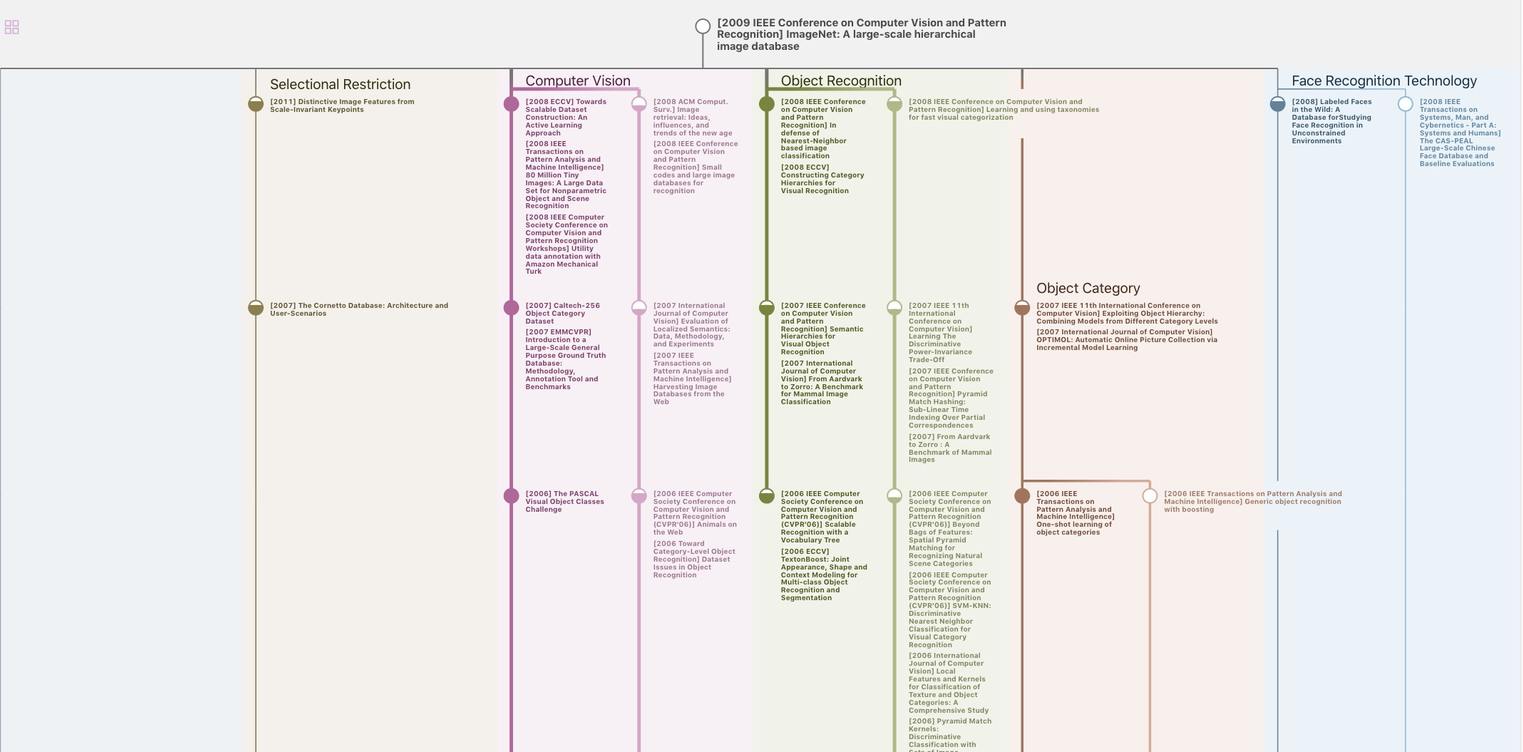
生成溯源树,研究论文发展脉络
Chat Paper
正在生成论文摘要