Ecophysiological modeling of yield and yield components in winter wheat using hierarchical Bayesian analysis
CROP SCIENCE(2022)
摘要
Yield components are widely recognized as drivers of wheat (Triticum aestivum L.) yield across environments and genotypes. In this study, we used a hierarchical Bayesian approach to model wheat grain yield in Oklahoma on an eco-physiological basis using yield component traits thousand kernel weight (TKW) and nonyield biomass (NYB). The Bayesian approach allowed us to quantify uncertainties around the parameter values rather than obtaining a single value estimate for a parameter. The main objectives of this study were to (a) explain wheat yield as a function of component traits TKW and NYB, and thereby examine the implications for source-sink balance; and (b) assess their association with weather conditions during key stages of wheat development. A secondary objective was to introduce Bayesian estimation for eco-physiological modeling. Fifteen wheat genotypes planted in three locations in Oklahoma (Altus, Chickasha, and Lahoma) were evaluated across three harvest years (2017 to 2019), whereby the combination of location and year defined an environment. Results indicate that the environment explained a greater proportion of the variability in yield than genotypes or than genotype x environment (G x E) interaction; however, evidence for G x E was substantial. Yield was expected to increase with increasing TKW and NYB, which would suggest a source limitation to achieve potential yield. Yet, the contribution of early reproductive stage weather variables to the relationship between yield and NYB pointed in the direction of sink strength being compromised. In summary, our approach provides evidence for source-sink co-limitation in grain yield of this sample of hard red winter wheat genotypes.
更多查看译文
关键词
bayesian analysis,winter wheat,yield components,ecophysiological modeling
AI 理解论文
溯源树
样例
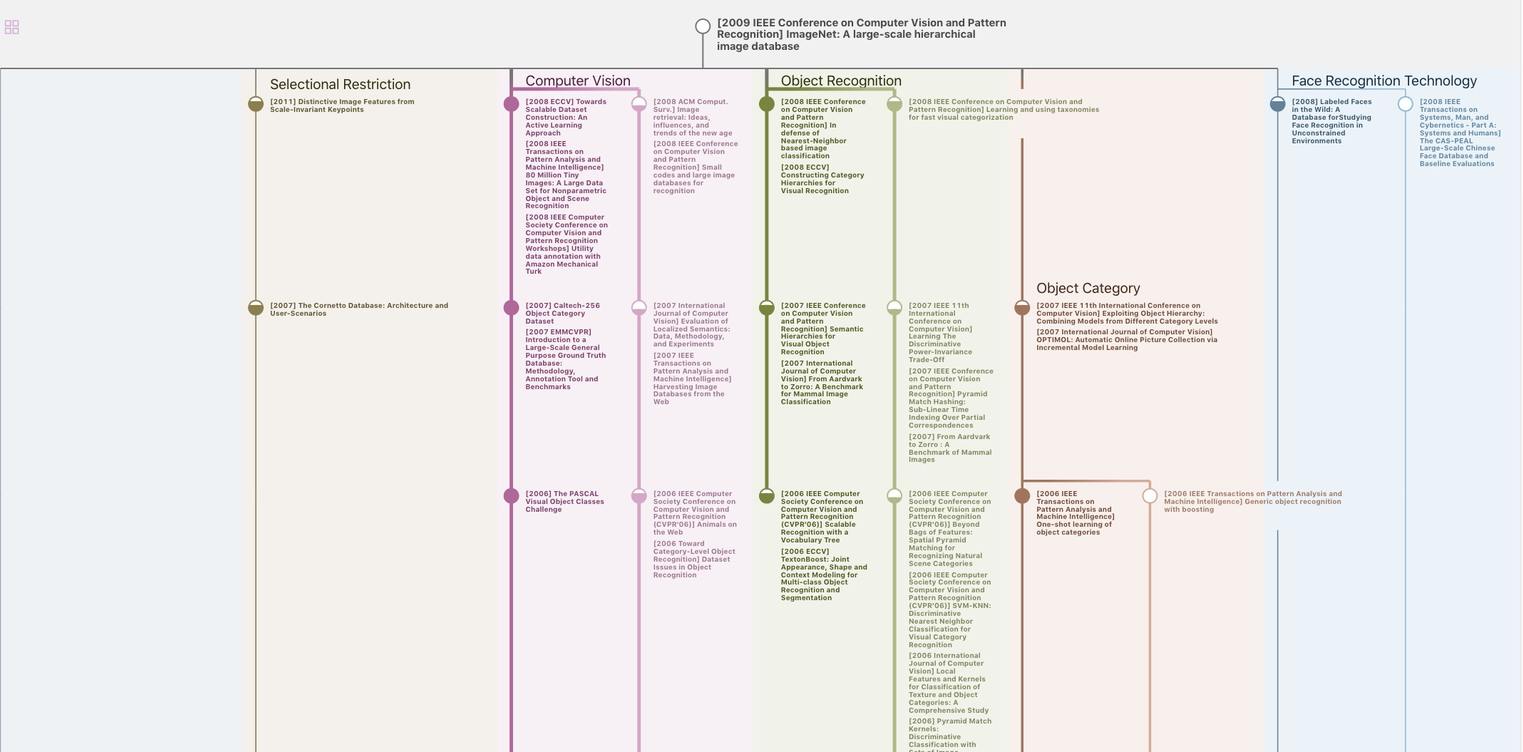
生成溯源树,研究论文发展脉络
Chat Paper
正在生成论文摘要