A Novel Multiple Feature-Based Engine Knock Detection System Using Sparse Bayesian Extreme Learning Machine
Cognitive computation(2022)
摘要
Automotive engine knock is an abnormal combustion phenomenon that affects engine performance and lifetime expectancy, but it is difficult to detect. Collecting engine vibration signals from an engine cylinder block is an effective way to detect engine knock. This paper proposes an intelligent engine knock detection system based on engine vibration signals. First, filtered signals are obtained by utilizing variational mode decomposition (VMD), which decomposes the original time domain signals into a series of intrinsic mode functions (IMFs). Moreover, the values of the balancing parameter and the number of IMF modes are optimized using genetic algorithm (GA). IMFs with sample entropy higher than the mean are then selected as sensitive subcomponents for signal reconstruction and subsequently removed. A multiple feature learning approach that considers time domain statistical analysis (TDSA), multi-fractal detrended fluctuation analysis (MFDFA) and alpha stable distribution (ASD) simultaneously, is utilized to extract features from the denoised signals. Finally, the extracted features are trained by sparse Bayesian extreme learning machine (SBELM) to overcome the sensitivity of hyperparameters in conventional machine learning algorithms. A test rig is designed to collect the raw engine data. Compared with other technology combinations, the optimal scheme from signal processing to feature classification is obtained, and the classification accuracy of the proposed integrated engine knock detection method can achieve 98.27%. We successfully propose and test a universal intelligence solution for the detection task.
更多查看译文
关键词
Engine Knock Detection,Variational Mode Decomposition,Multiple Feature Learning,Sample Entropy,Sparse Bayesian Extreme Learning Machine
AI 理解论文
溯源树
样例
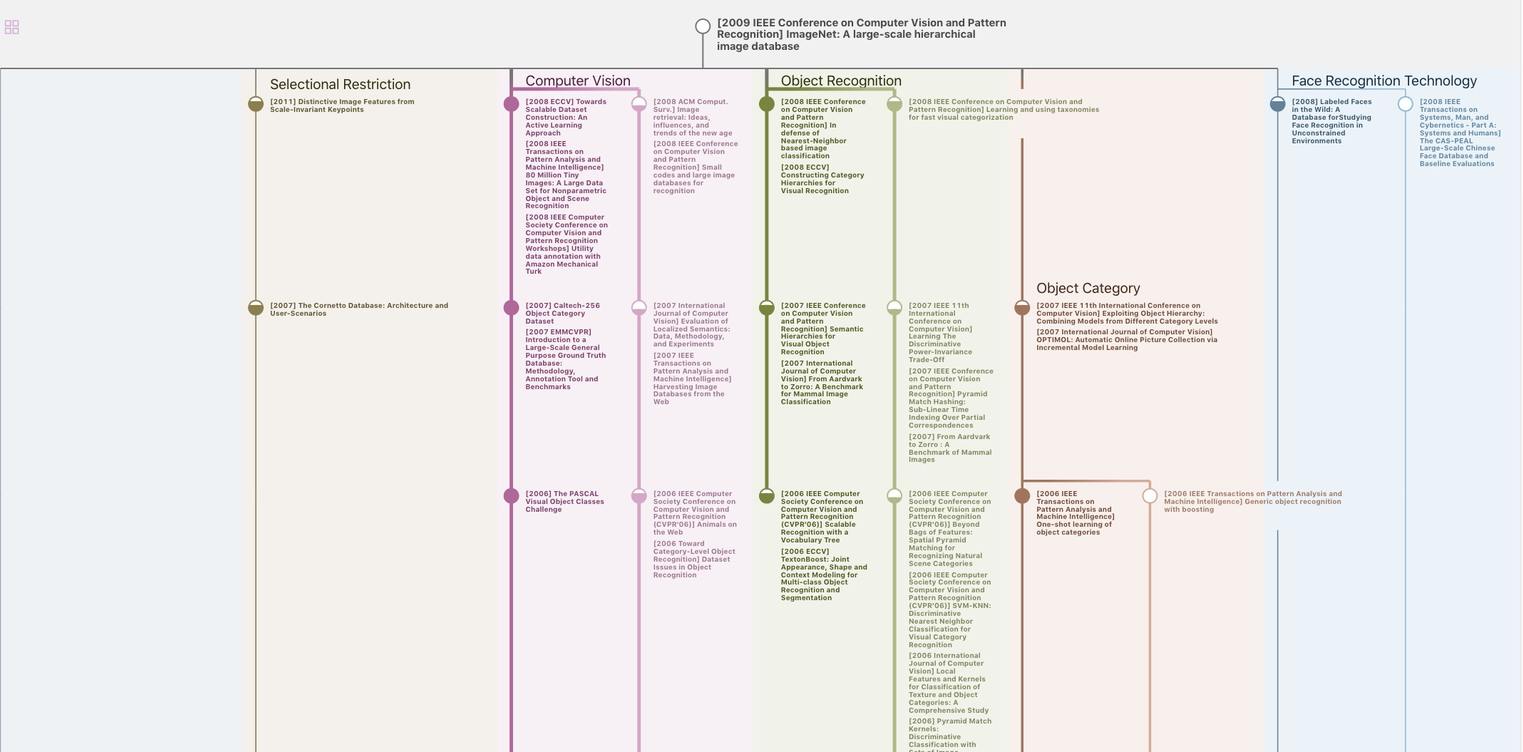
生成溯源树,研究论文发展脉络
Chat Paper
正在生成论文摘要