Machine learning and in-silico screening of metal-organic frameworks for O2/N2 dynamic adsorption and separation
CHEMICAL ENGINEERING JOURNAL(2022)
摘要
It remains a great challenge to separate O-2 from N-2 at room temperature. Pressure swing adsorption (PSA) technology is a potential candidate, and the development of high-efficiency adsorbents for O-2/N-2 separation at room temperature has attracted a great deal of interest. In this work, machine learning (ML)-assisted high-throughput computational screening (HTCS) techniques were performed to screen the dynamic adsorption of O-2 and N-2 in 6,013 computation-ready experimental metal-organic frameworks (CoRE-MOFs), including the competitive adsorption of O-2 and the diffusion of pure N-2 and O-2, to identify the best materials for O-2/N-2 separation. First, based on HTCS, we established the relationships between the structural/energetic descriptors with the performance indicators. Three machine learning (ML) algorithms were then applied to predict the performance indicators of MOFs. In addition, the relative importance of the structural/energetic descriptors and metal center type in MOFs toward the separation performance was evaluated, indicating that the metal center type of MOFs is a key factor for the separation of O-2/N-2. Transition metal elements were determined to have highest importance by ML. Moreover, the 13 best MOFs were identified for the dynamic adsorption of O-2 from the air. Finally, three types of design strategies could significantly improve the performance of MOFs, such as regulating the topology and alternating the metal node and organic linker. The combination of HTCS, ML, and design strategies from bottom to top provide powerful microscopic insights for the development of MOF adsorbents for the separation of O-2 at room temperature.
更多查看译文
关键词
Metal-organic frameworks,Simulation,High-throughput computational screening,Machine learning
AI 理解论文
溯源树
样例
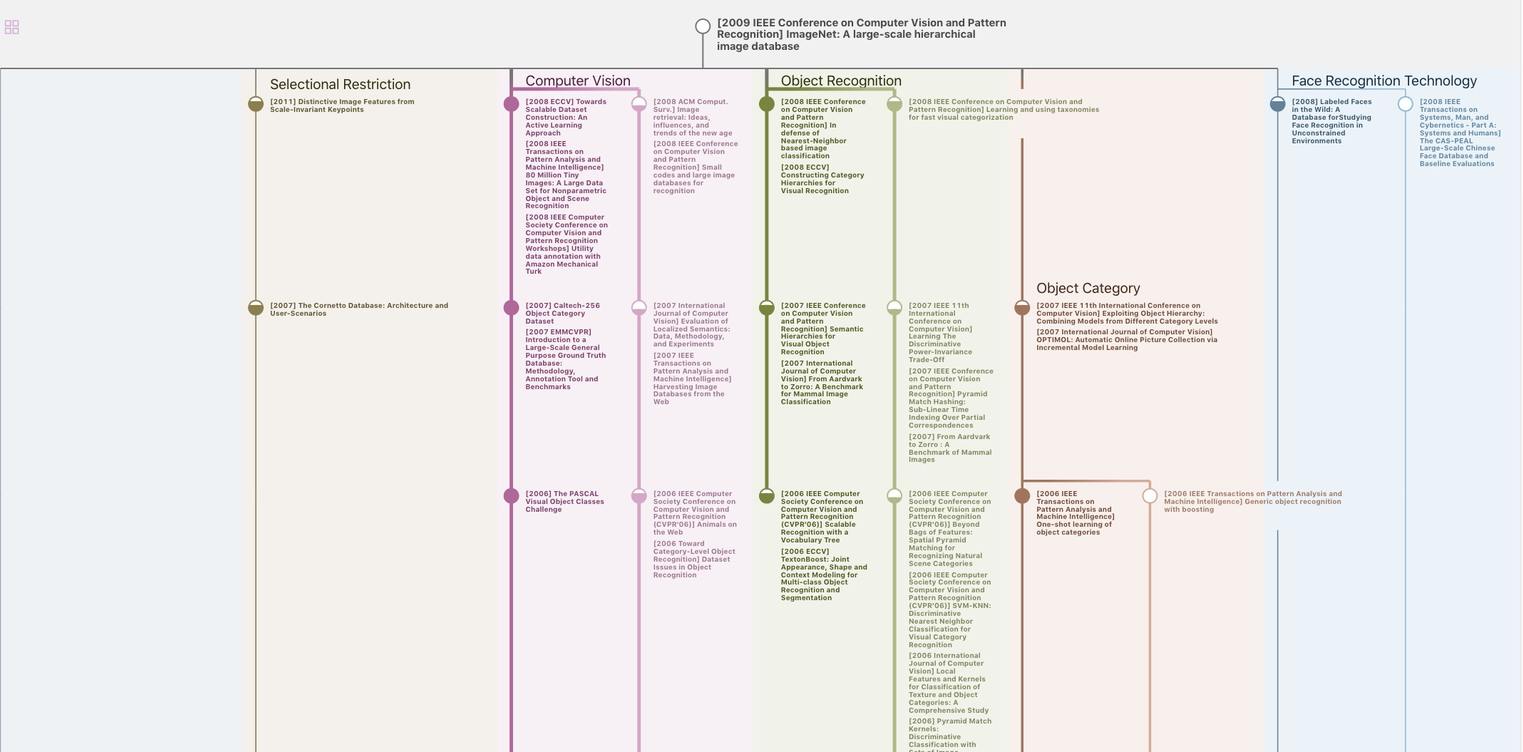
生成溯源树,研究论文发展脉络
Chat Paper
正在生成论文摘要