Moran eigenvector filtering of multi-year yield data with application to zone development
AGROSYSTEMS GEOSCIENCES & ENVIRONMENT(2021)
摘要
A time-series of yield monitor data may be used to identify field areas of consistently low or high yield to serve as productivity zones for site-specific crop management. However, transient factors that affect yield in 1 yr, but not every year, detract from this approach. The objective of this study was to illustrate Moran eigenvector spatial filtering (MESF) with results from analysis of multi-year crop yield data from two farm fields in the United States. The MESF method accounts for temporal autocorrelation within a common factor map representing the correlation across years and partitions stochastic geographic variation into spatially structured and unstructured components. Crop rotation data were utilized from a dryland field in east-central South Dakota and an irrigated field in southwestern Georgia. A random effects (RE) model was estimated that utilized eigenfunctions of a geographic connectivity matrix to account for spatially structured random effects (SSRE) and unstructured random effects (SURE) in standardized z scores of multi-year crop yield. The MESF method was evaluated with conventional averaging of unfiltered yield data as a reference for comparison. In South Dakota, the SSRE accounted for 26% of the yield variance shared across years. Distinct patterns appeared to be related to changes in soil type and landscape position. The Georgia field yielded similar results. The MESF is effective for revealing structured variation in a time series of yield monitor data and may be useful for defining productivity zones within fields.
更多查看译文
关键词
zone development,yield
AI 理解论文
溯源树
样例
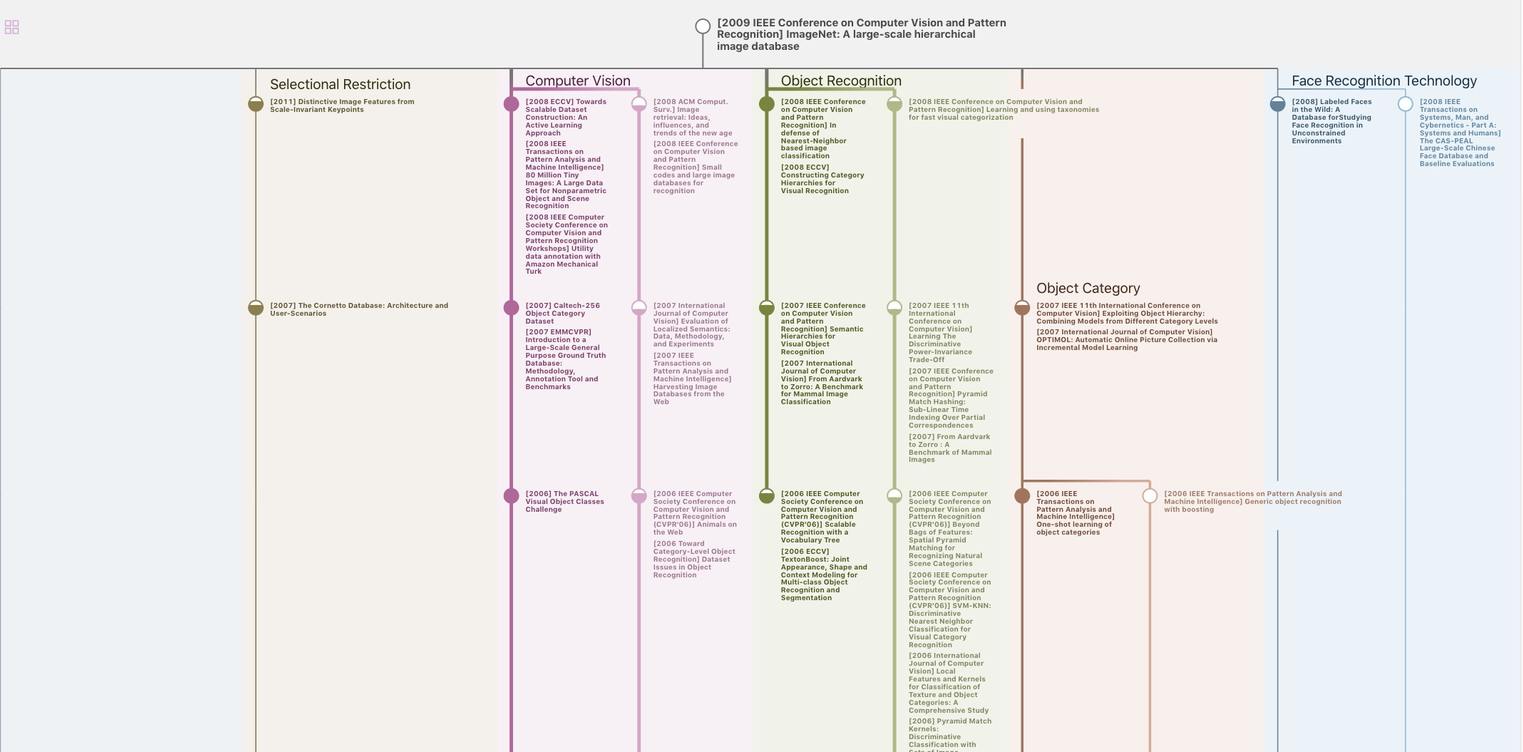
生成溯源树,研究论文发展脉络
Chat Paper
正在生成论文摘要